Machine Learning-Based Automatic Segmentation Of Region Of Interest In Dynamic Optical Imaging
AIP ADVANCES(2021)
摘要
Compared with static optical imaging, dynamic optical imaging technology can obtain quantitative pharmacokinetics information, such as the probe metabolism curve, removal rate, and binding potential of the receptor. Accurate segmentation of the region of interest (ROI) is an important step in dynamic optical imaging. Generally, the ROI is manually labeled by researchers based on experience. This will lead to two unavoidable problems. First, manual segmentation of the ROI is very time consuming, especially when there are many sequential dynamic optical images. Second, manual segmentation cannot ensure accuracy when the optical signal gradually decays to a point at which it is difficult to distinguish by using the naked eyes. These problems will inevitably lead to inaccuracy of quantitative results of dynamic optical imaging. Here, we presented a machine learning-based automatic segmentation method to avoid these time-consuming and inaccuracy problems caused by manual segmentation. The K-means clustering algorithm and fuzzy c-means clustering algorithm were implemented to separate the ROI from the background of sequential dynamic optical images. Automatic selection of clustering results was completed by mathematical methods. The accuracy and feasibility of machine learning-based methods were verified by comparing their results with the manual segmentation results. The preliminary results demonstrated that the machine learning-based automatic segmentation has coherent performance with the manual one.
更多查看译文
AI 理解论文
溯源树
样例
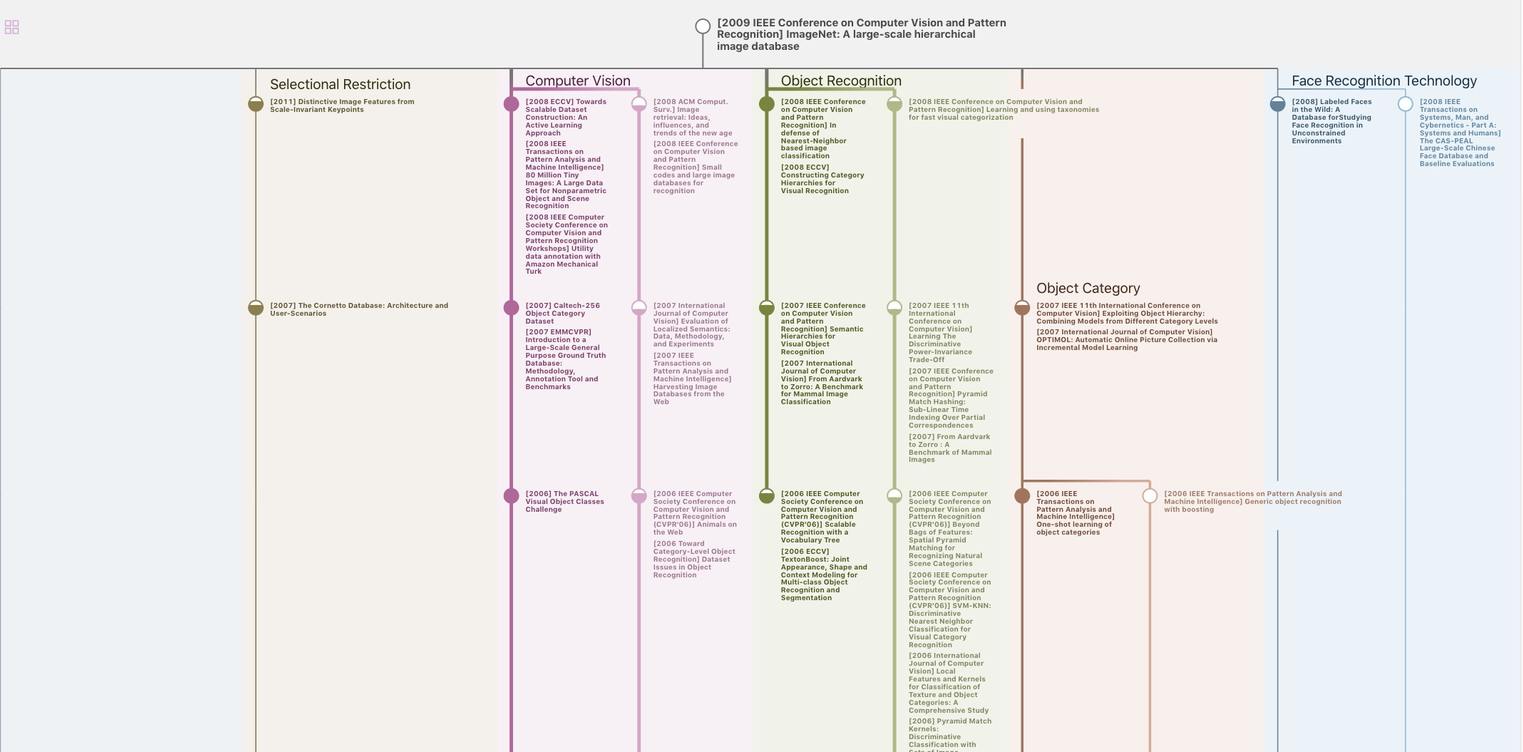
生成溯源树,研究论文发展脉络
Chat Paper
正在生成论文摘要