Identity-constrained noise modeling with metric learning for face anti-spoofing.
Neurocomputing(2021)
摘要
Face presentation attack detection (PAD) has become a key component in face-based application systems. Typical face de-spoofing algorithms estimate the noise pattern of a spoof image to detect presentation attacks. These algorithms are device-independent and have good generalization ability. However, the noise modeling is not very effective because there is no ground truth (GT) with identity information for training the noise modeling network. To address this issue, we propose using the bona fide image of the corresponding subject in the training set as a type of GT called appr-GT with the identity information of the spoof image. A metric learning module is proposed to constrain the generated bona fide images from the spoof images so that they are near the appr-GT and far from the input images. This can reduce the influence of imaging environment differences between the appr-GT and GT of a spoof image. Extensive experimental results demonstrate that the reconstructed bona fide image and noise with high discriminative quality can be clearly separated from a spoof image. The proposed algorithm achieves competitive performance.
更多查看译文
关键词
Presentation attack detection,Identity constrain,Noise modeling,Metric learning
AI 理解论文
溯源树
样例
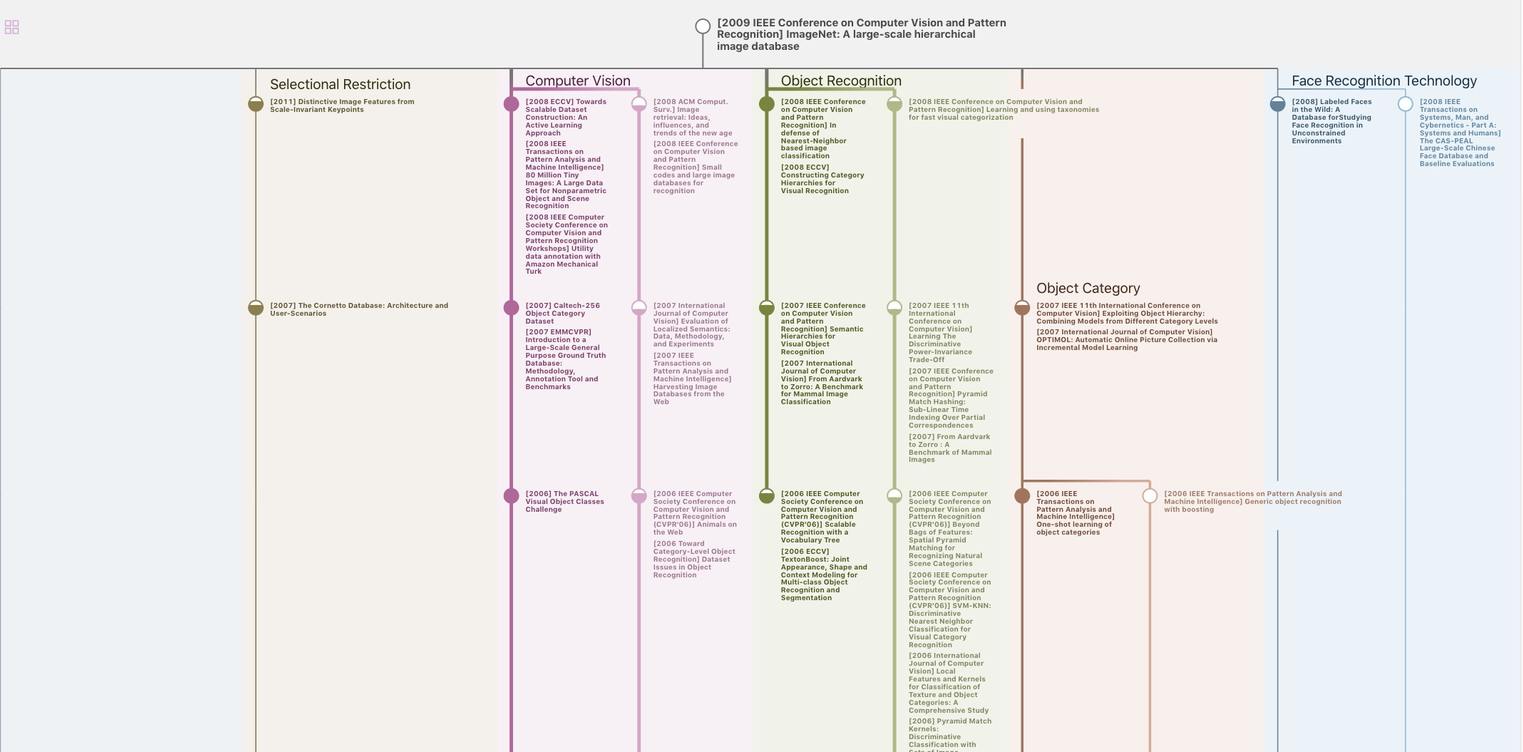
生成溯源树,研究论文发展脉络
Chat Paper
正在生成论文摘要