CFFNN: Cross Feature Fusion Neural Network for Collaborative Filtering
IEEE Transactions on Knowledge and Data Engineering(2022)
摘要
Numerous state-of-the-art recommendation frameworks employ deep neural networks in Collaborative Filtering (CF). In this paper, we propose a cross feature fusion neural network (CFFNN) for the enhancement of CF. Existing studies overlook either user preferences for various item features or the relationship between item features and user features. To solve this problem, we construct a cross feature fusion network to enable the fusion of user features and item features as well as a self-attention network to determine users’ preferences for items. Specifically, we design a feature extraction layer with multiple MLP (Multilayer Perceptrons) modules to extract both user features and item features. Then, we introduce a cross feature fusion mechanism for an accurate determination of the relationship between different user-item interactions. The features of users and items are crossly embedded and then fed into a prediction network. The attention mechanism enables the model to focus on more effective features. The effectiveness of CFFNN model is demonstrated through extensive experiments on four real-world datasets. The experimental results indicate that CFFNN significantly outperforms the existing state-of-the-art models, with a relative improvement of 3.0 to 12.1 percent on hit ratio (HR) and normalized discounted cumulative gain (NDCG) compared with the baselines.
更多查看译文
关键词
Collaborative filtering,neural recommendation models,cross feature fusion,attention networks
AI 理解论文
溯源树
样例
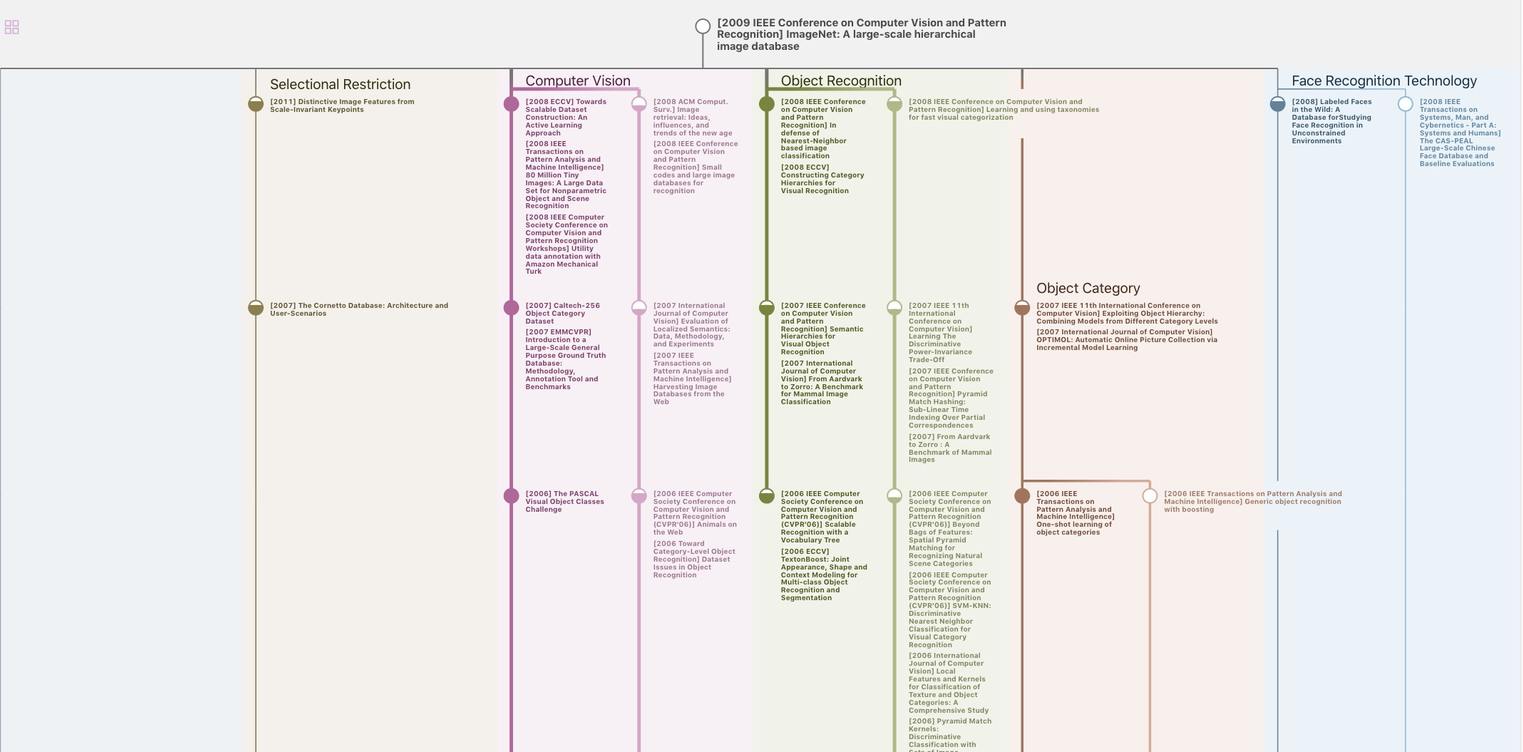
生成溯源树,研究论文发展脉络
Chat Paper
正在生成论文摘要