Automated iceberg tracking with a machine learning approach applied to SAR imagery: A Weddell sea case study
ISPRS Journal of Photogrammetry and Remote Sensing(2021)
摘要
Drifting icebergs represent a significant hazard for polar navigation and are able to impact the ocean environment around them. Freshwater flux and the associated cooling from melting icebergs can locally decrease salinity and temperature and thus affect ocean circulation, biological activity, sea ice, and –on larger spatial scales– the whole climate system. However, despite their potential impact, the large-scale operational monitoring of drifting icebergs in sea ice-covered regions is as of today typically restricted to giant icebergs, larger than 18.5 km in length. This is due to difficulties in accurately identifying and following the motion of much smaller features in the polar ocean from space. So far, tracking of smaller icebergs from satellite imagery thus has been limited to open-ocean regions not covered by sea ice. In this study, a novel automated iceberg tracking method, based on a machine learning-approach for automatic iceberg detection, is presented. To demonstrate the applicability of the method, a case study was performed for the Weddell Sea region, Antarctica, using 1213 Advanced Synthetic Aperture Radar (ASAR) satellite images acquired between 2002 and 2011. Overall, a subset of 414 icebergs (3134 re-detections in total) with surface areas between 3.4 km2 and 3612 km2 were investigated with respect to their prevalent drift patterns, size variability, and average disintegration. The majority of the tracked icebergs drifted between 1.3 km and 2679.2 km westward around the Antarctic continent, following the Antarctic Coastal Current (ACoC) and the Weddell Gyre, at an average drift speed of 3.6 ± 7.4 km day−1. The method also allowed us to estimate an average daily disintegration (i.e. iceberg area decrease) rate of ~0.13% (~37% year−1) for all icebergs. Using the sum of all detected individual surface area reductions, we estimate a total iceberg mass decrease of ~683 Gt year−1, which can be freshwater input and/or new ‘child’ icebergs calved from larger icebergs. The extension to an automated long-term tracking method for icebergs is challenging as the iceberg shape can vary significantly due to abrupt disintegration or calving of bergy bits. However, our machine learning approach extended by automatic shape-based tracking capabilities proved to be a reliable alternative for automatic detection and tracking of icebergs, even under the ambiguous SAR background signatures often found in the Southern Ocean. In particular, the method works in the challenging near-coastal environment where the presence of sea ice and coastal ocean dynamics such as surface waves usually pose major obstacles for other approaches.
更多查看译文
关键词
Icebergs,SAR,Tracking,Southern Ocean,Machine learning,Antarctic
AI 理解论文
溯源树
样例
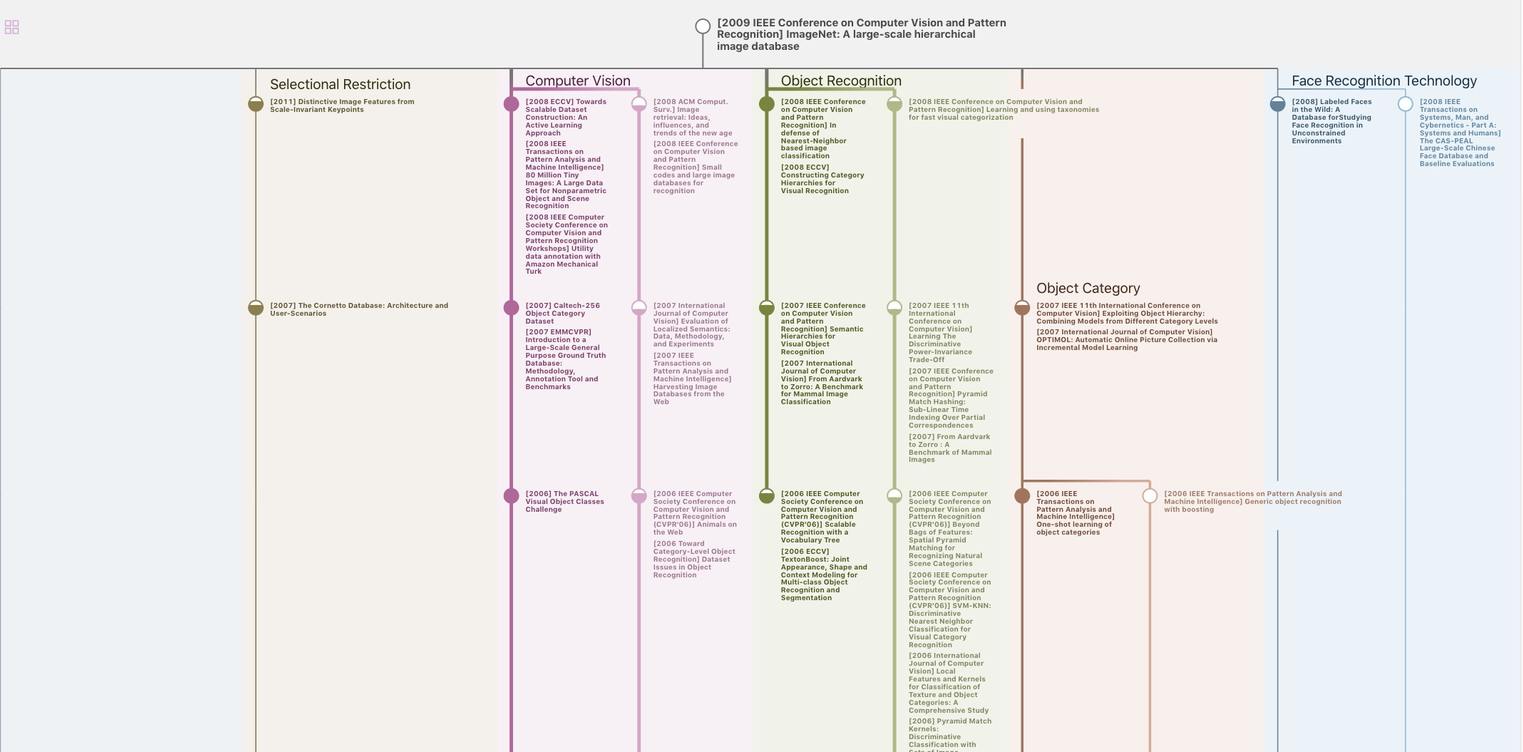
生成溯源树,研究论文发展脉络
Chat Paper
正在生成论文摘要