Novel Approaches to Feasibility Determination
ACM Transactions on Modeling and Computer Simulation(2021)
摘要
AbstractThis article proposes two-stage Bayesian and frequentist procedures for determining whether a number of systems—each characterized by the same number of performance measures—belongs to a set Γ defined by a finite collection of linear inequalities. A system is “in (not in) Γ” if the vector of the means is in (not in) Γ, where the means must be estimated using Monte Carlo simulation. We develop algorithms for classifying the systems with a user-specified level of confidence using the minimum number of simulation replications so the probability of correct classification over all r systems satisfies a user-specified minimum value. Once the analyst provides prior values for the means and standard deviations of the random variables in each system, an initial number of simulation replications is performed to obtain current estimates of the means and standard deviations to assess whether the systems can be classified with the desired level of confidence. For any system that cannot be classified, heuristics are proposed to determine the number of additional simulation replications that would enable correct classification. Our contributions include the introduction of intuitive algorithms that are not only easy to implement, but also effective with their performance. Compared to other feasibility determination approaches, they also appear to be competitive. While the algorithms were initially developed in settings where system variance is assumed to be known and the random variables are independent, their performance remains satisfactory when those assumptions are relaxed.
更多查看译文
关键词
Multiple performance measures, ranking and selection, stochastic constraints, classifying system
AI 理解论文
溯源树
样例
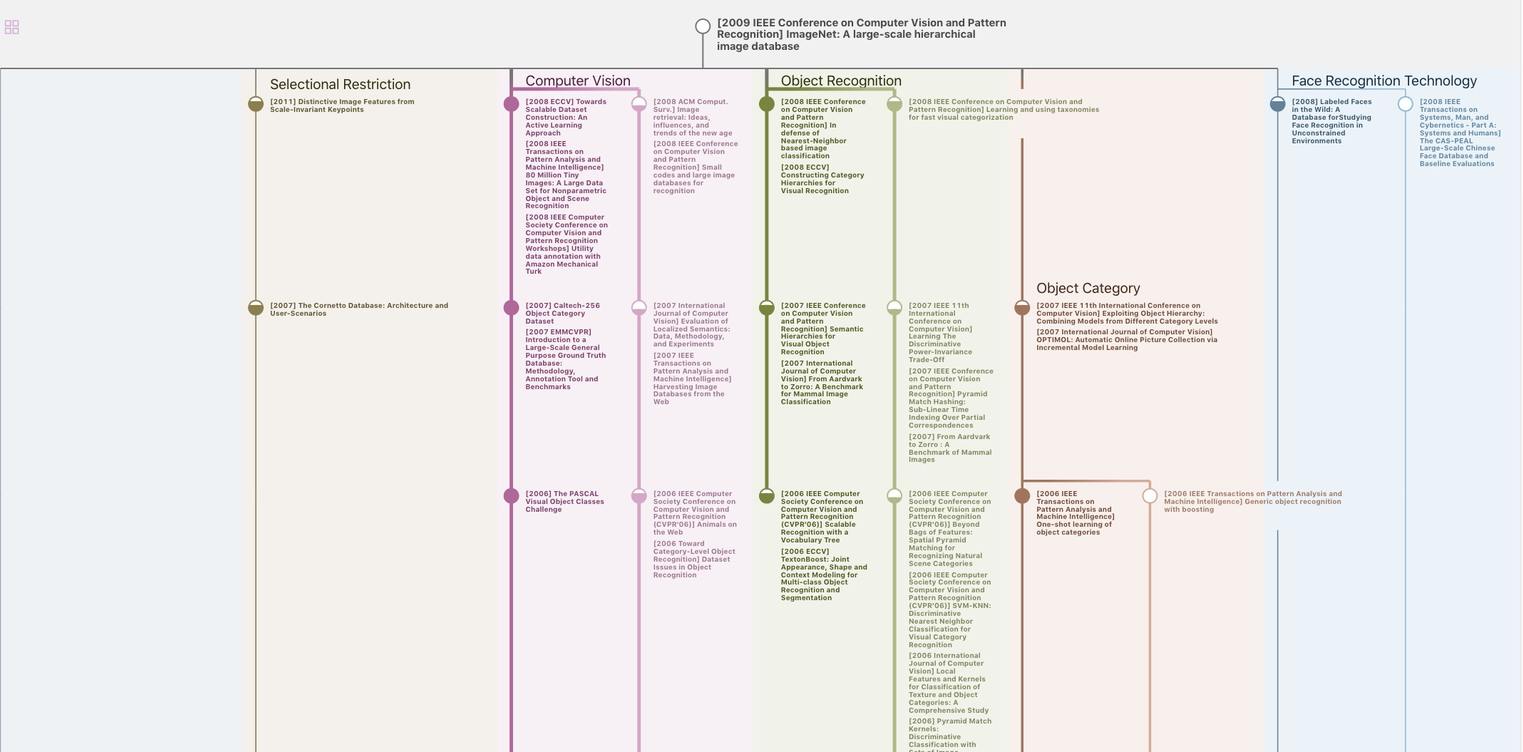
生成溯源树,研究论文发展脉络
Chat Paper
正在生成论文摘要