Robust People Indoor Localization With Omnidirectional Cameras Using A Grid Of Spatial-Aware Classifiers
SIGNAL PROCESSING-IMAGE COMMUNICATION(2021)
摘要
This paper describes a system for people indoor localization using omnidirectional cameras and machine learning that significantly reduces the database annotation requirements for the training stage. The most prominent works for people detection are based on machine learning techniques that requires large databases with bounding box annotations (that enclose the people). In this work, a novel multiple classifier system, called Grid of Spatial-Aware Classifiers (GSAC), is proposed, which only requires point-based annotations, allowing to create datasets much faster (and therefore speeding up the system deployment). On the other hand, omnidirectional images have a wider field of view than traditional ones, allowing to monitor a wider area, and thus reducing deployment costs. But in return, they present severe geometric distortions that degrade the performance of state of the art detectors, due to the strong changes in the person appearance caused by the position-dependent distortion. The proposed GSAC satisfactorily addresses this problem by distributing the detection task among all spatial-aware classifiers, so that each classifier has only to deal with a subrange of appearances and distortions. Lastly, a thorough evaluation has been performed on two databases of omnidirectional images: a well-known one and one specifically designed to assess the people detection performance.
更多查看译文
关键词
Spatial grid, Spatial-aware classifiers, Omnidirectional cameras, Fish-eye, Indoor localization, People detection, Object detection, Machine learning
AI 理解论文
溯源树
样例
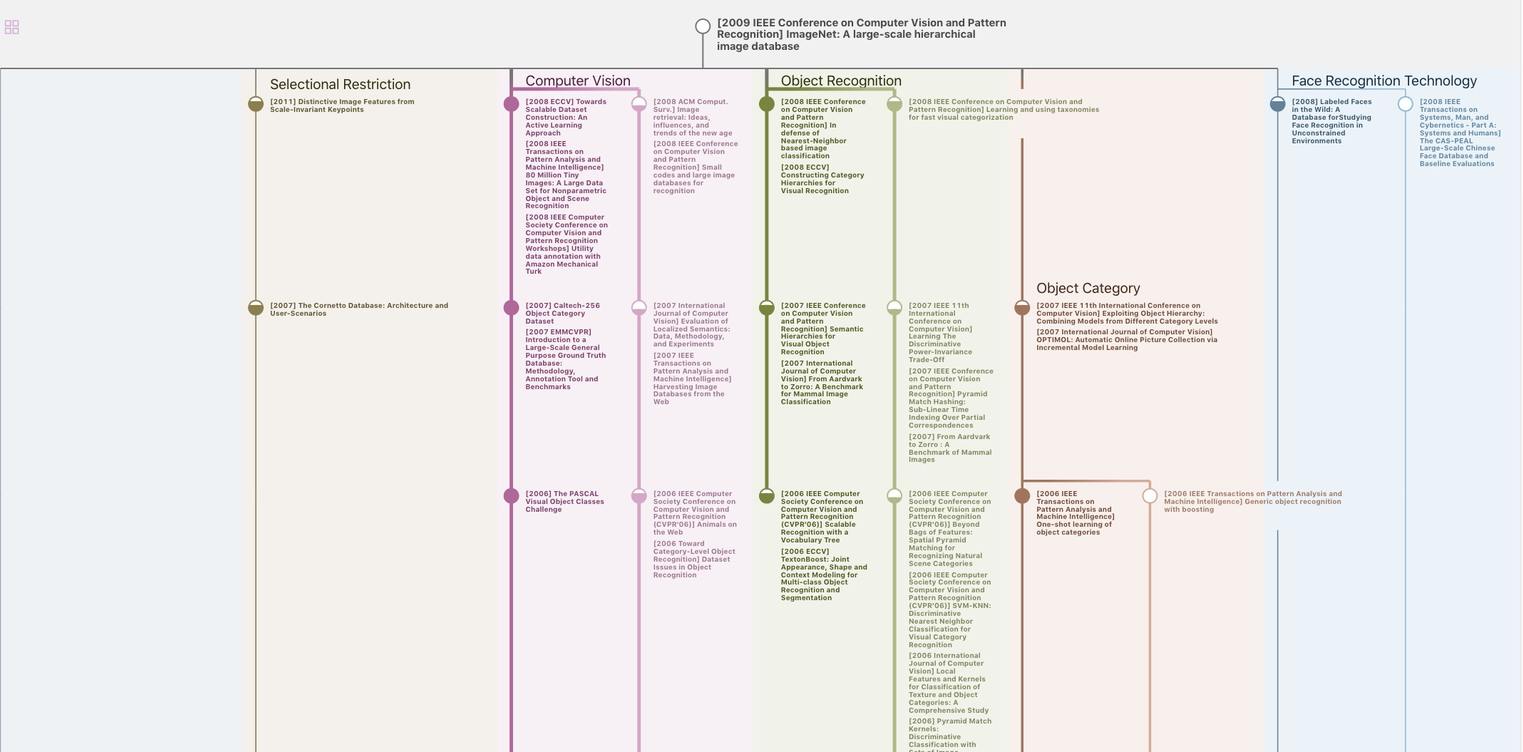
生成溯源树,研究论文发展脉络
Chat Paper
正在生成论文摘要