Using Generative Adversarial Networks And Parameter Optimization Of Convolutional Neural Networks For Lung Tumor Classification
APPLIED SCIENCES-BASEL(2021)
摘要
Cancer is the leading cause of death worldwide. Lung cancer, especially, caused the most death in 2018 according to the World Health Organization. Early diagnosis and treatment can considerably reduce mortality. To provide an efficient diagnosis, deep learning is overtaking conventional machine learning techniques and is increasingly being used in computer-aided design systems. However, a sparse medical data set and network parameter tuning process cause network training difficulty and cost longer experimental time. In the present study, the generative adversarial network was proposed to generate computed tomography images of lung tumors for alleviating the problem of sparse data. Furthermore, a parameter optimization method was proposed not only to improve the accuracy of lung tumor classification, but also reduce the experimental time. The experimental results revealed that the average accuracy can reach 99.86% after image augmentation and parameter optimization.
更多查看译文
关键词
lung cancer, convolutional neural network, generative adversarial network, image augmentation, parameter optimization
AI 理解论文
溯源树
样例
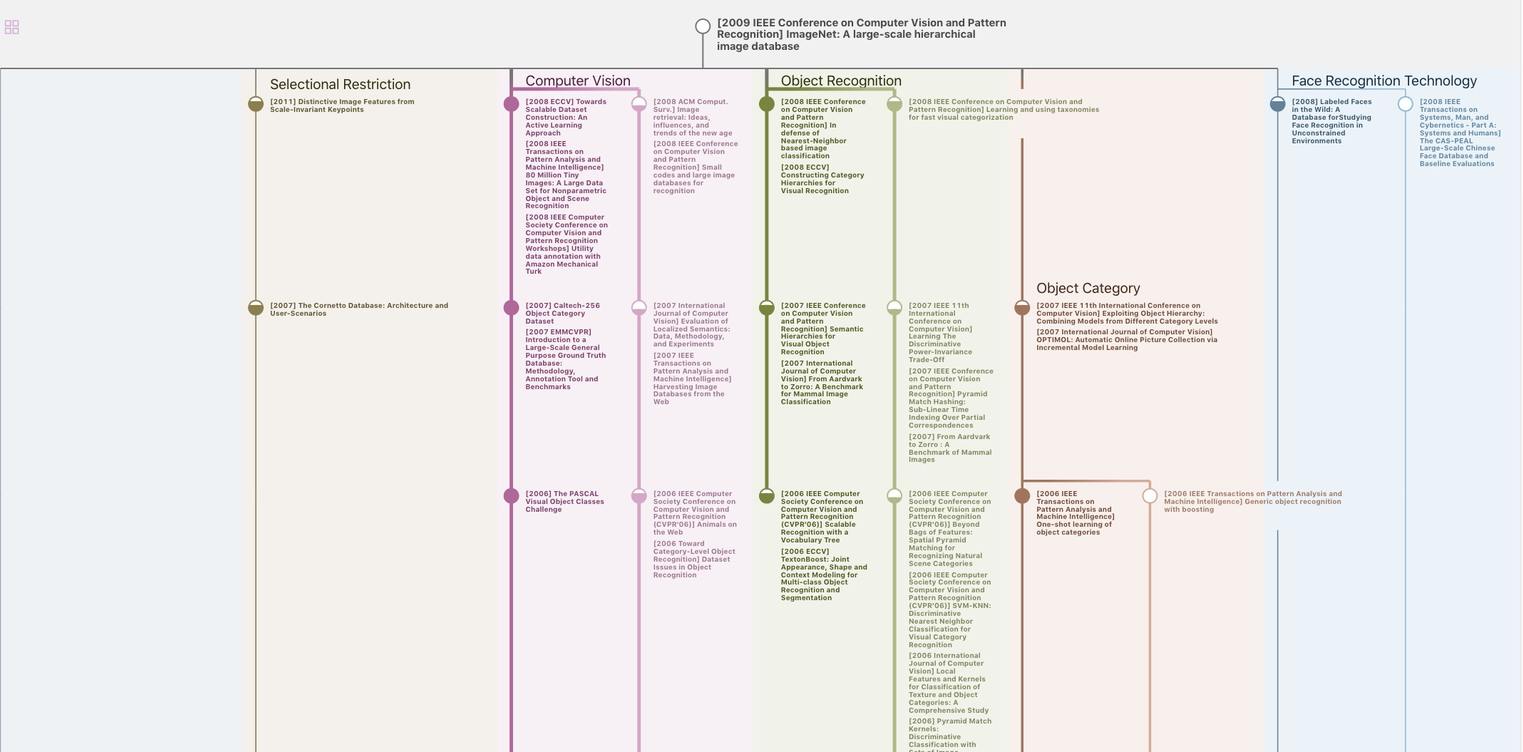
生成溯源树,研究论文发展脉络
Chat Paper
正在生成论文摘要