Improving the Accuracy of Clustering Electric Utility Net Load Data using Dynamic Time Warping
ieee/pes transmission and distribution conference and exposition(2020)
摘要
Identifying patterns in electric utility net load data in a time-series format is very useful in preparing the operation for next day. Machine learning algorithms have been used in other domains and those concepts are applied in this paper on real-world net load measurement data. Clustering is the practice of grouping data with similar characteristics as determined by the distance measure. The K-means clustering algorithm is utilized here with actual electric utility data. The paper uses the standard distance measure, Euclidean distance (ED), and compares its performance against the dynamic time warping (DTW) measure. An actual case study with real data is presented, and DTW distance measure-based method observed to result better accuracy compared to the ED based method for substation net load measurements predominantly with residential customers.
更多查看译文
关键词
Electric load profiles,machine learning,time-series,clustering,pattern recognition
AI 理解论文
溯源树
样例
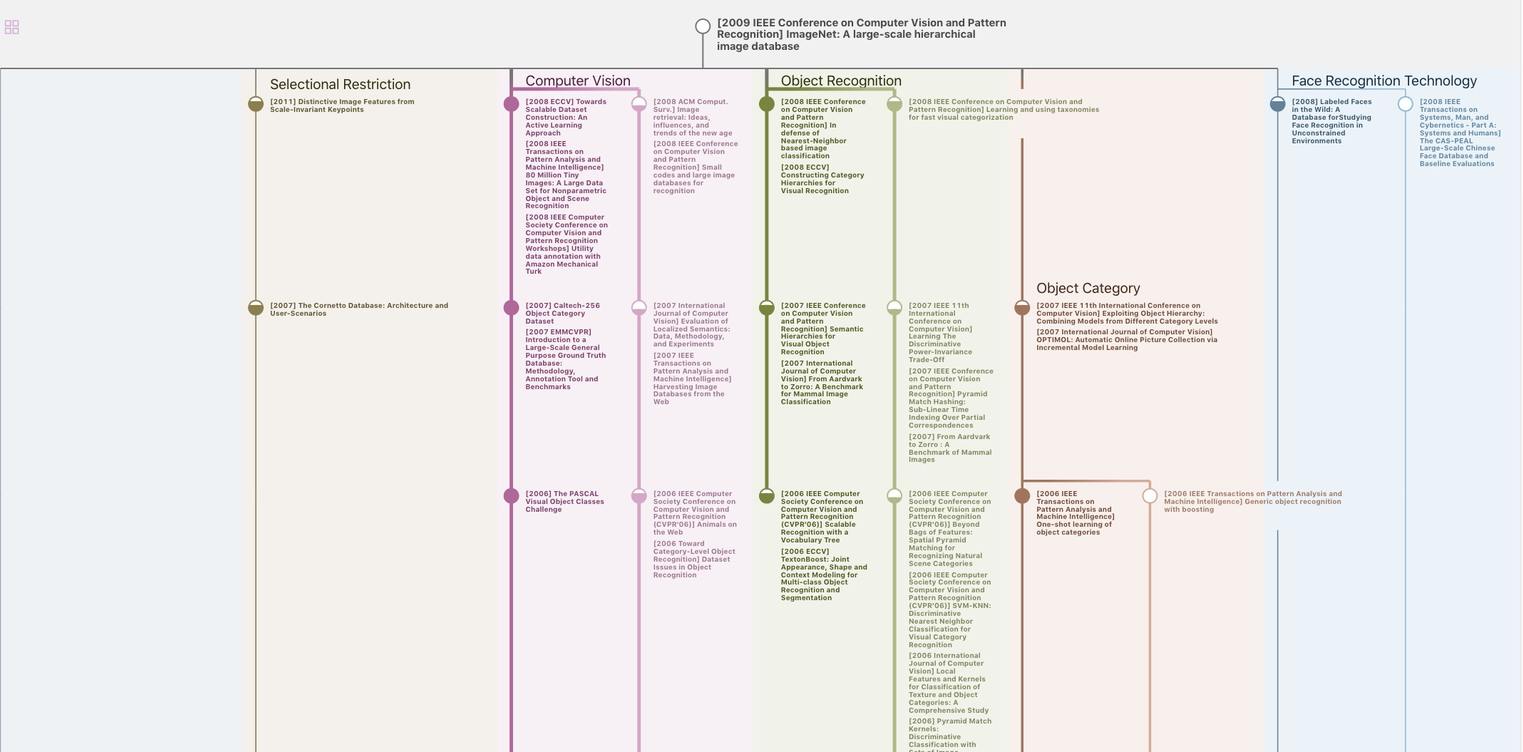
生成溯源树,研究论文发展脉络
Chat Paper
正在生成论文摘要