Robust and high-order correlation alignment for unsupervised domain adaptation
NEURAL COMPUTING & APPLICATIONS(2021)
摘要
How to measure the domain discrepancy is of significant importance in the field of unsupervised domain adaptation. Among them, Correlation Alignment (CORAL), aligning second-order statistics of source and target domains, has become one of the most widely used discrepancy-based methods. However, the performance of CORAL is limited by: (1) aligning covariance with usual Euclidean metric is suboptimal, and (2) second-order statistics have limited expression for the non-Gaussian distribution. To address these limitations, we propose a Robust Correlation Alignment as well as a High-order Correlation Alignment method. The Robust Correlation Alignment exploits the geometric structure of covariance with matrix square-root normalization. To circumvent unstable and time-consuming properties of the Singular Value Decomposition, we employ the variant of Newton iteration to compute the matrix square-root. Besides, we also propose a High-order Correlation Alignment method, which exploits the third-order statistics for domain alignment. We show that the High-order CORAL can be generalized to Maximum Mean Discrepancy, CORAL and arbitrary-order statistics. Specifically, we propose group matching to reduce space complexity and improve the feasibility in real-world application. Extensive experiments on standard benchmark datasets demonstrate that our proposed methods outperform previous methods by a large margin.
更多查看译文
关键词
Unsupervised domain adaptation,Correlation Alignment,Riemannian manifold,Square-root normalization,High-order statistics
AI 理解论文
溯源树
样例
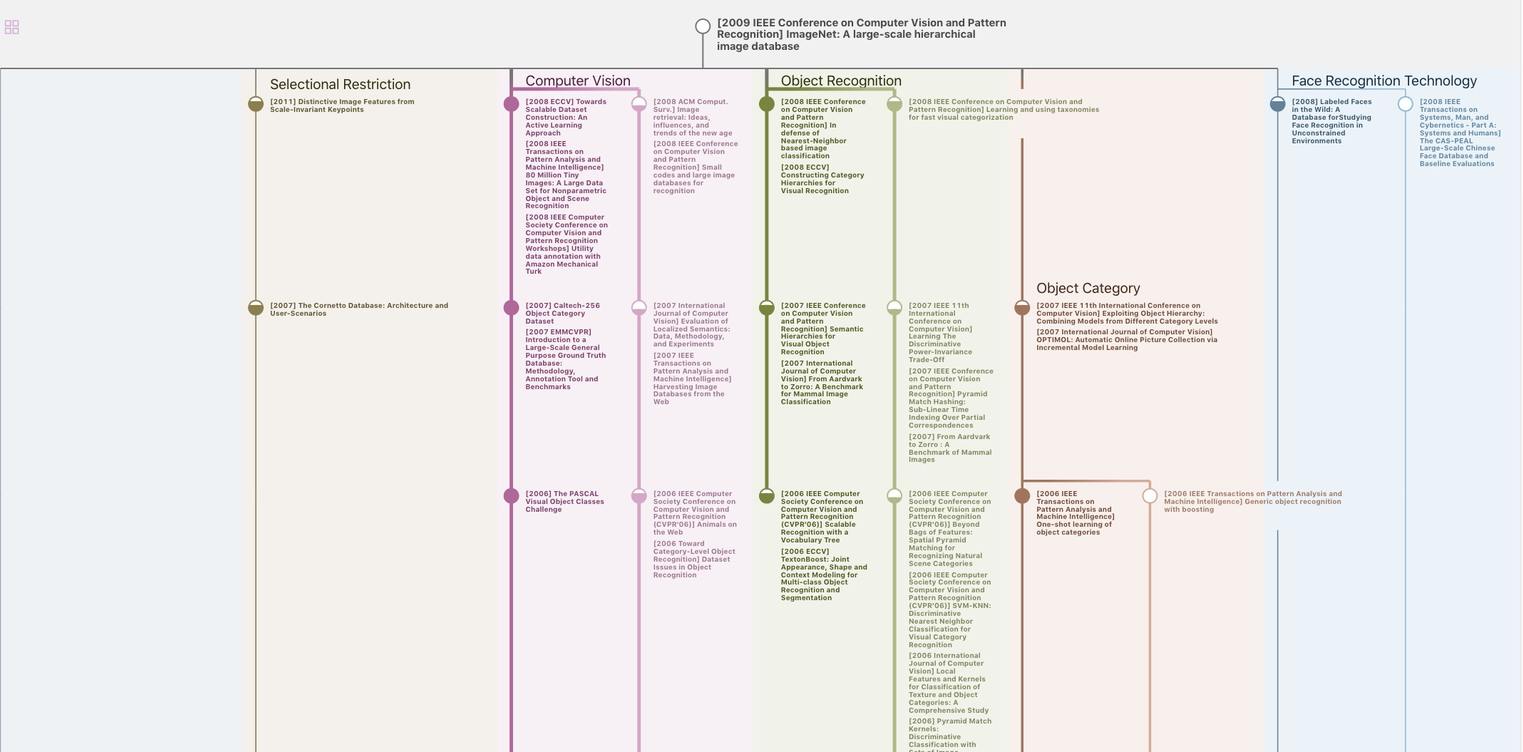
生成溯源树,研究论文发展脉络
Chat Paper
正在生成论文摘要