Entropy based optimal scale combination selection for generalized multi-scale information tables
INTERNATIONAL JOURNAL OF MACHINE LEARNING AND CYBERNETICS(2021)
摘要
In many real-life applications, data are often hierarchically structured at different levels of granulations. A multi-scale information table is a special hierarchical data set in which each object can take on as many values as there are scales under the same attribute. An important issue in such a data set is to select optimal scale combination in order to keep certain condition for final decision. In this paper, by employing Shannon’s entropy, we study the selection of optimal scale combination to maintain uncertain measure of a knowledge from a generalized multi-scale information table. We first review the concept of entropy and its basic properties in information tables. We then introduce the notion of scale combinations in a generalized multi-scale information table. We further define entropy optimal scale combination in generalized multi-scale information tables and generalized multi-scale decision tables. Finally, we examine relationship between the entropy optimal scale combination and the classical optimal scale combination. We show that, in either a generalized multi-scale information table or a consistent generalized multi-scale decision table, the entropy optimal scale combination and the classical optimal scale combination are equivalent. And in an inconsistent generalized multi-scale decision table, a scale combination is generalized decision optimal if and only if it is a generalized decision entropy optimal.
更多查看译文
关键词
Entropy, Granular computing, Information tables, Multi-scale information tables, Scale combinations
AI 理解论文
溯源树
样例
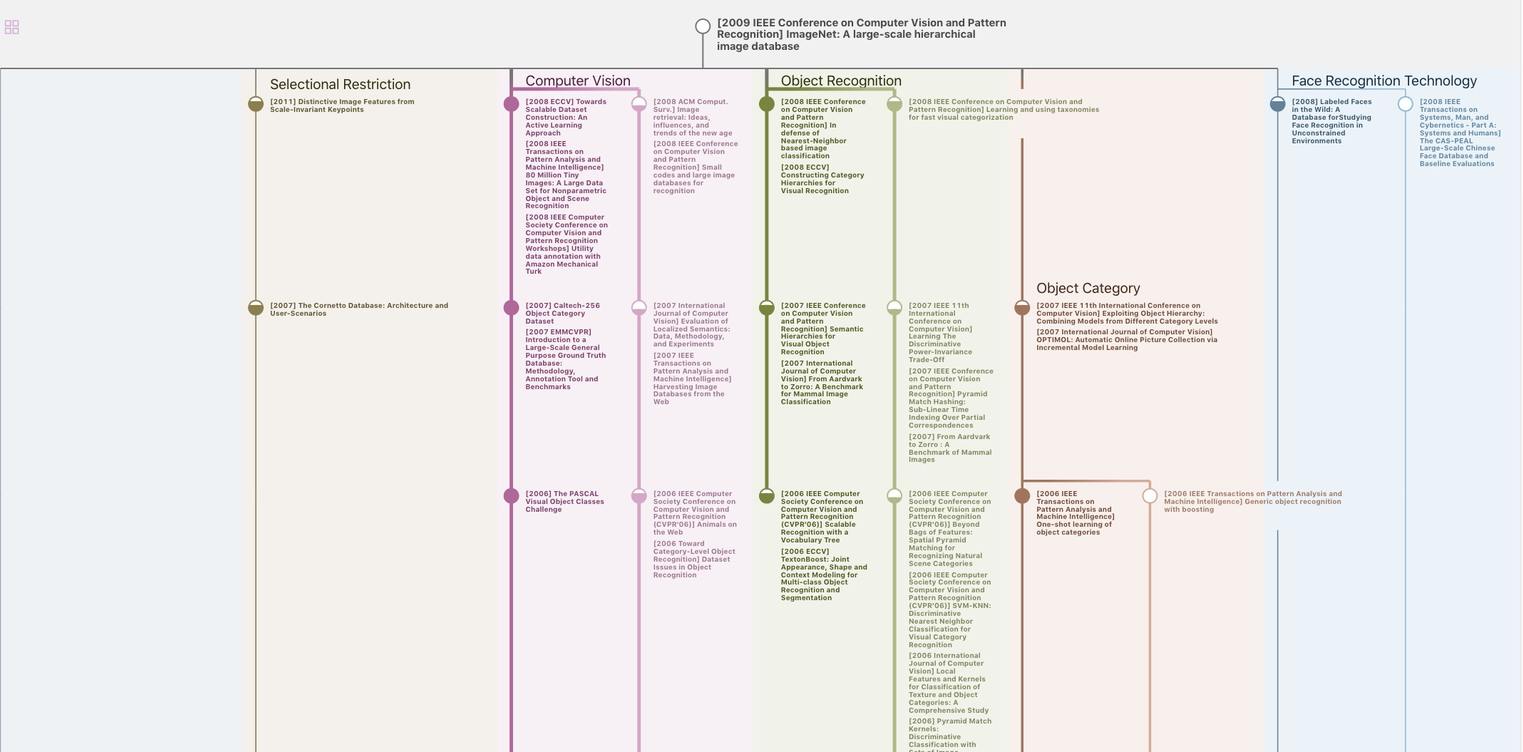
生成溯源树,研究论文发展脉络
Chat Paper
正在生成论文摘要