Fadenet: Deep Learning-Based Mm-Wave Large-Scale Channel Fading Prediction And Its Applications
IEEE ACCESS(2021)
摘要
Accurate prediction of the large-scale channel fading is fundamental to planning and optimization in 5G millimeter-wave cellular networks. The current prediction methods, which are either too computationally expensive or inaccurate, are unsuitable for city-scale cell planning and optimization. This paper presents FadeNet, a convolutional neural-network enabled alternative for predicting large-scale fading with high computation speed and accuracy. By using carefully designed input features and neural-network architecture to capture topographical information, FadeNet accurately predicts the large-scale fading from a base station to each location in its coverage area. Evaluations on realistic data, derived from millimeter-wave cells across multiple cities, suggest that FadeNet can achieve a prediction accuracy of 5.6 decibels in root mean square error. In addition, by leveraging the parallel processing capabilities of a graphics processing unit, FadeNet can reduce the prediction time by 40X - 1000X in comparison to industry prevalent methods like ray-tracing. Generalizations of FadeNet, that can handle variable topographies and base station heights, and its use for optimal cell site selection are also explored.
更多查看译文
关键词
Cell planning, channel modeling, convolutional networks, deep learning, large scale fading, mm-Wave, pathloss, U-net
AI 理解论文
溯源树
样例
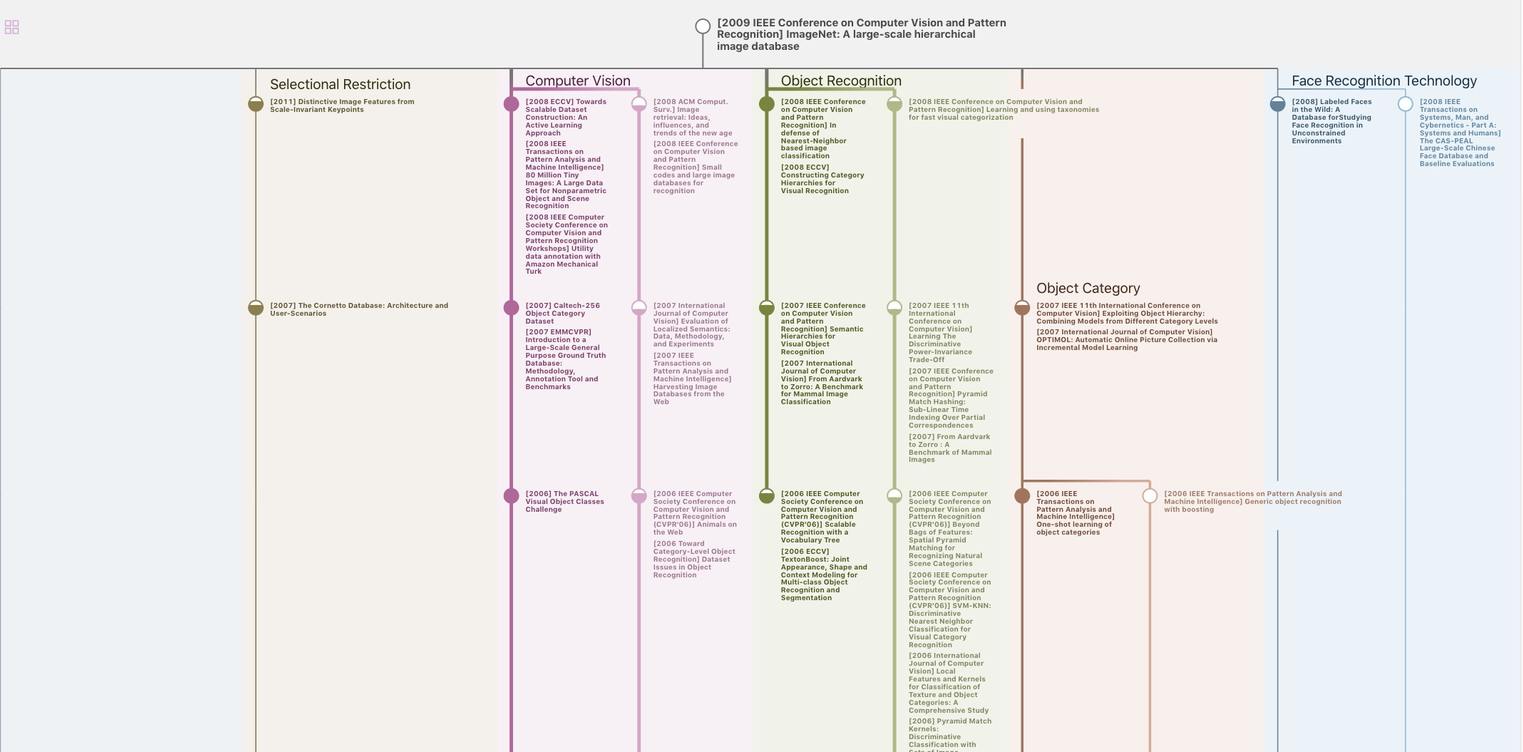
生成溯源树,研究论文发展脉络
Chat Paper
正在生成论文摘要