DUAL-PATH MODELING FOR LONG RECORDING SPEECH SEPARATION IN MEETINGS
2021 IEEE INTERNATIONAL CONFERENCE ON ACOUSTICS, SPEECH AND SIGNAL PROCESSING (ICASSP 2021)(2021)
摘要
The continuous speech separation (CSS) is a task to separate the speech sources from a long, partially overlapped recording, which involves a varying number of speakers. A straightforward extension of conventional utterance-level speech separation to the CSS task is to segment the long recording with a size-fixed window and process each window separately. Though effective, this extension fails to model the long dependency in speech and thus leads to sub-optimum performance. The recent proposed dual-path modeling could be a remedy to this problem, thanks to its capability in jointly modeling the cross-window dependency and the local-window processing. In this work, we further extend the dual-path modeling framework for CSS task. A transformer-based dual-path system is proposed, which integrates transform layers for global modeling. The proposed models are applied to LibriCSS, a real recorded multi-talk dataset, and consistent WER reduction can be observed in the ASR evaluation for separated speech. Also, a dual-path transformer equipped with convolutional layers is proposed. It significantly reduces the computation amount by 30% with better WER evaluation. Furthermore, the online processing dual-path models are investigated, which shows 10% relative WER reduction compared to the baseline.
更多查看译文
关键词
continuous speech separation, long recording speech separation, online processing, dual-path modeling
AI 理解论文
溯源树
样例
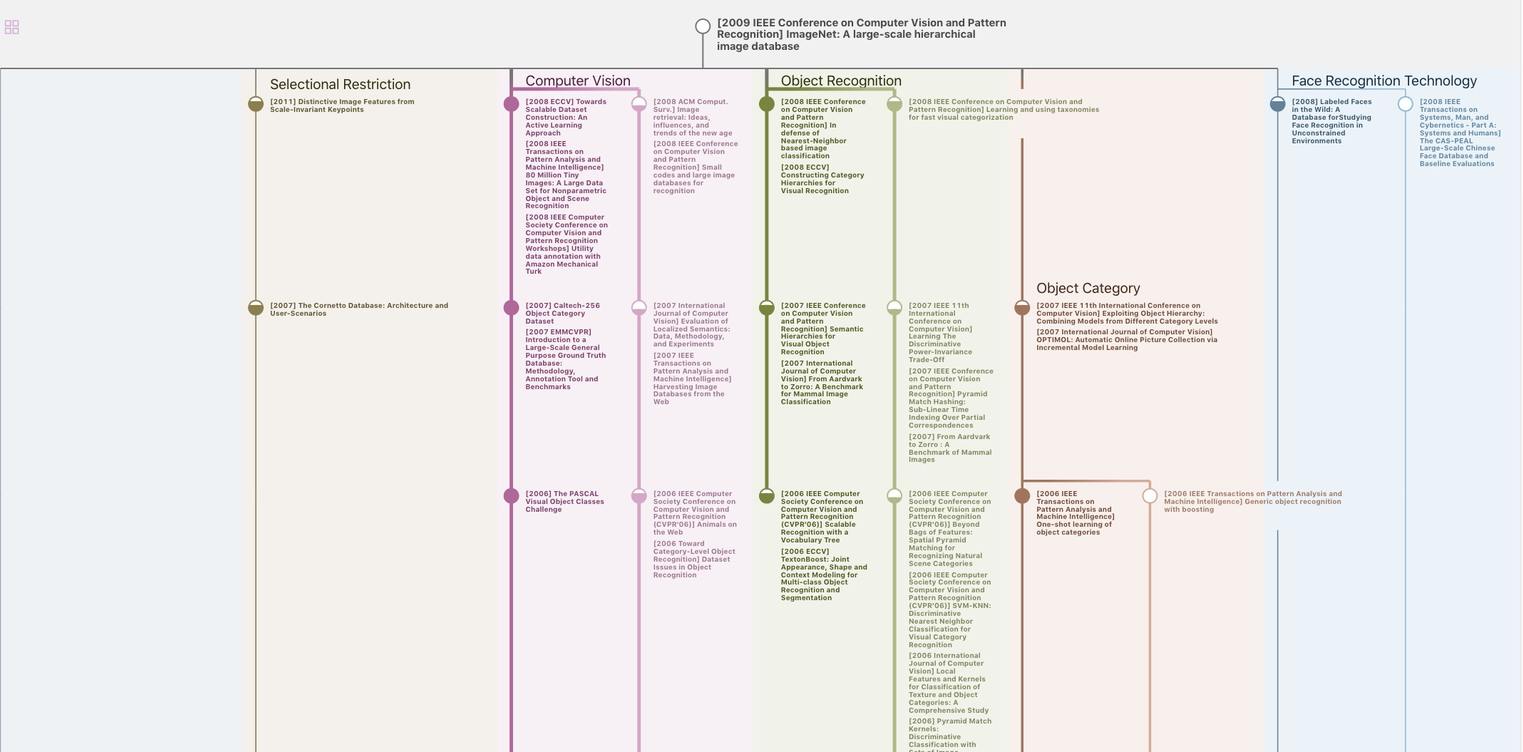
生成溯源树,研究论文发展脉络
Chat Paper
正在生成论文摘要