A Quantitative Analysis on the Use of Supervised Machine Learning in Earth Science
IGARSS 2020 - 2020 IEEE INTERNATIONAL GEOSCIENCE AND REMOTE SENSING SYMPOSIUM(2020)
摘要
Recent review papers have discussed the opportunities and challenges of applying machine learning (ML) techniques to Earth science data. A common challenge cited in these papers is the lack of labeled training data. A literature review of Earth science papers over the last 10 years demonstrates that while there is rapid adoption of ML, particularly in biogeoscience and land surface research, the training datasets typically contain only hundreds of samples. This lack of training data limits the use of deep learning algorithms, which require larger volumes of labeled data. In situ training data are most frequently used in almost all domains, followed by model output and satellite data. The atmosphere and solid Earth domains use the largest training datasets, an order of magnitude larger than in biogeoscience papers. Random forest is the most commonly applied ML algorithm in all domains except atmospheric science and biogeoscience, which more frequently use fully connected neural networks.
更多查看译文
关键词
supervised machine learning, Earth science, deep learning
AI 理解论文
溯源树
样例
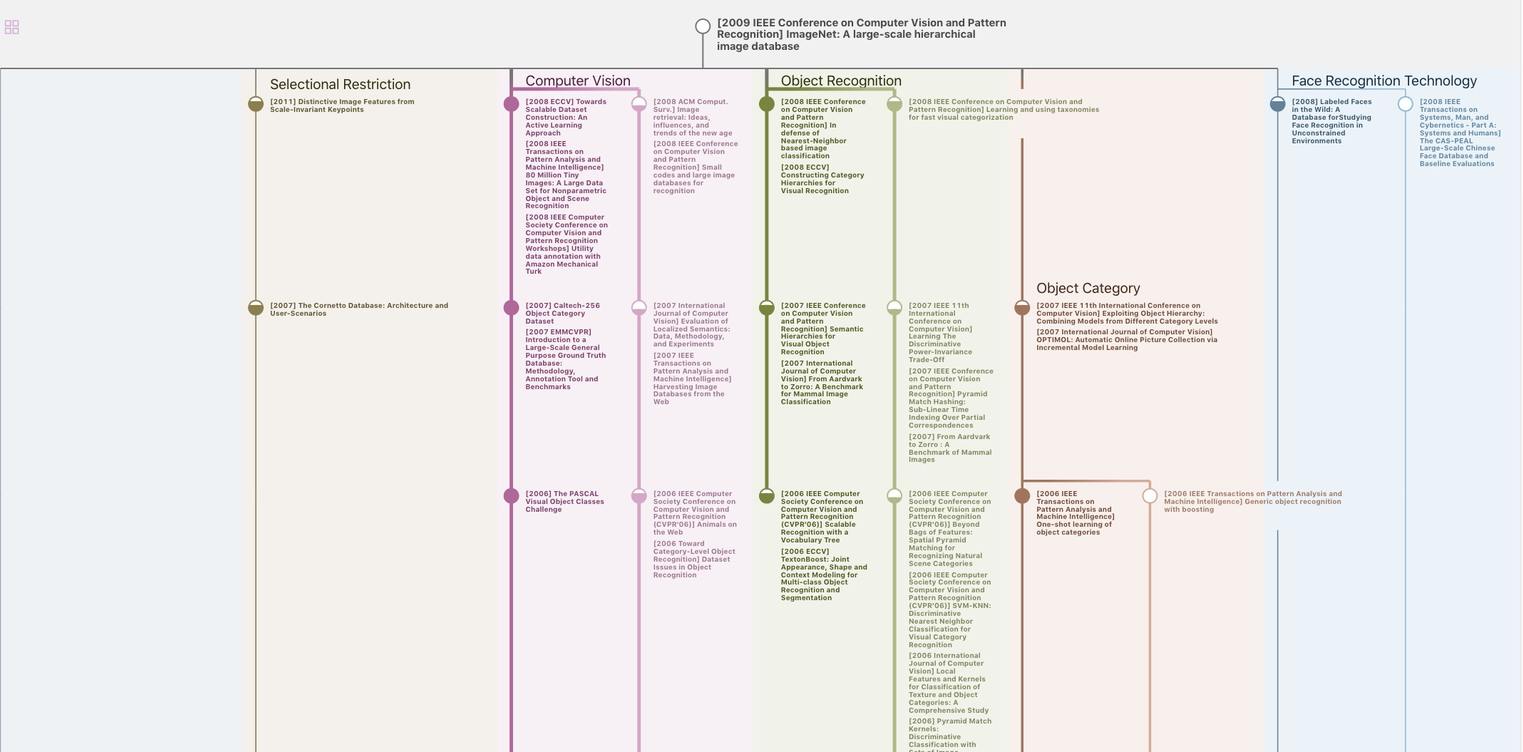
生成溯源树,研究论文发展脉络
Chat Paper
正在生成论文摘要