L-0-Motivated Low Rank Sparse Subspace Clustering For Hyperspectral Imagery
IGARSS 2020 - 2020 IEEE INTERNATIONAL GEOSCIENCE AND REMOTE SENSING SYMPOSIUM(2020)
摘要
Hyperspectral image (HSI) Clustering is an unsupervised task, which segments pixels into different groups without using labeled samples. Low-rank sparse subspace clustering (LRSSC) is often applied to achieve the clustering of high-dimensional data such as HSI. The LRSSC combines low-rank recovery and sparse representation to capture both global and local structures of the data. Nuclear and L-1-norm are often used to measure rank and sparsity in LRSSC since minimization of these two norms results in a convex optimization problem. However, the use of Nuclear and L-1-norm can only approximate the original problem, and may lead to over-penalization. Thus, the direct solution of a Schatten-0 (S-0) and L-0 quasi-norm regularized objective function has been proposed in the LRSSC for more accurate representation. This paper proposes to use the S-0/L-0-regulared LRSSC (S-0/L-0-LRSSC) for hyperspectral image clustering. To accommodate the large data size, an original HSI is pre-partitioned, and the S-0/L-0-LRSSC is implemented in a distributed way. Our experiments show that the performance of the S-0/L-0-LRSSC in hyperspectral image clustering is better than the original LRSSC and its variants based on Nuclear and L-1-norm minimization.
更多查看译文
关键词
Hyperspectral image (HSI), low-rank recovery, sparse representation, subspace clustering
AI 理解论文
溯源树
样例
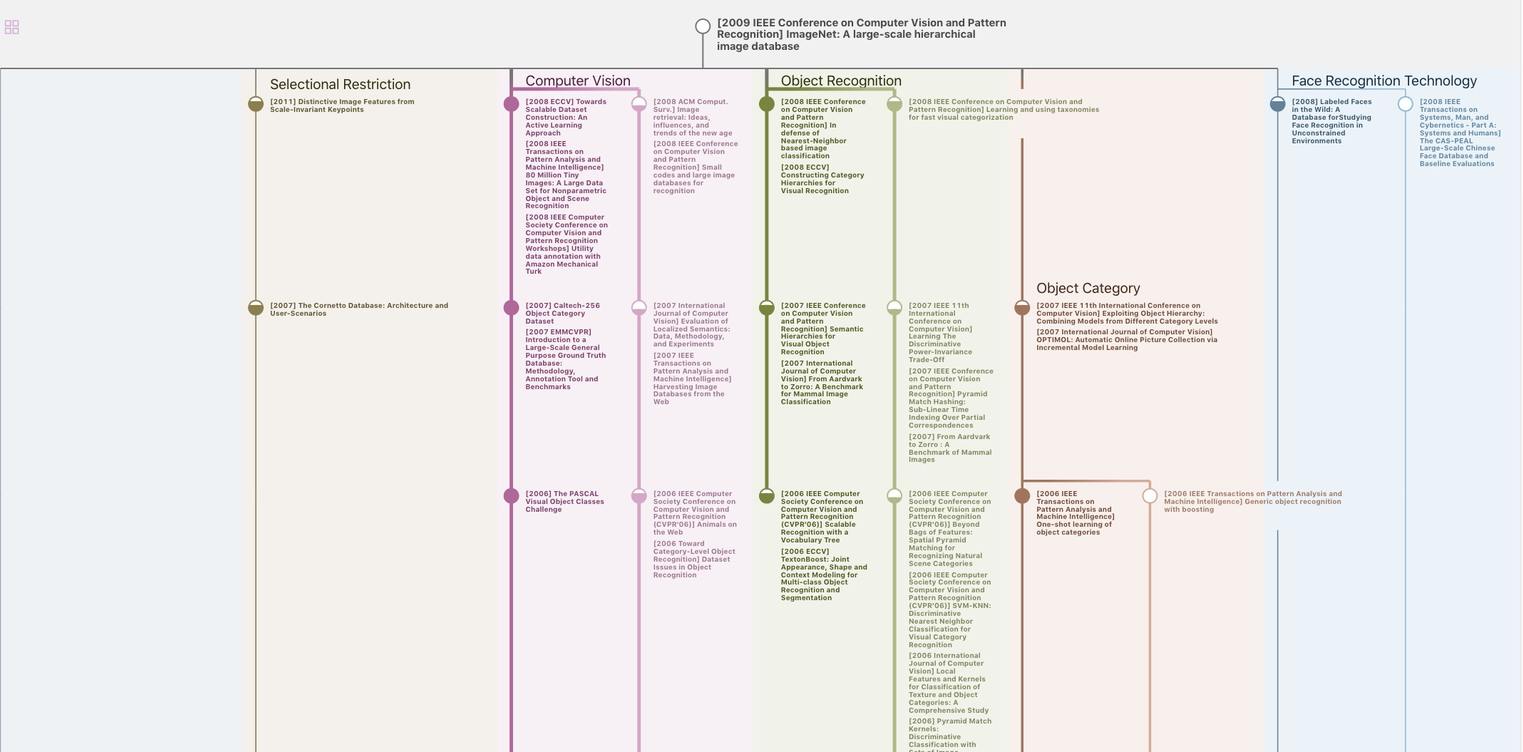
生成溯源树,研究论文发展脉络
Chat Paper
正在生成论文摘要