Cnn-Based Building Footprint Detection From Sentinel-1 Sar Imagery
IGARSS 2020 - 2020 IEEE INTERNATIONAL GEOSCIENCE AND REMOTE SENSING SYMPOSIUM(2020)
摘要
This research addresses the use of machine learning for detecting building footprints from dual-polarization multi-temporal SAR data. We assume that in SAR images, built-up areas exhibit very high backscattering values, making them brighter than other land cover classes in both the co- and cross-polarization channels. In addition the building class is considered to be stable in time. In this context, we propose to employ a convolutional neural network that integrates a set of SAR images of different dates and polarizations for delineating the building footprint. The algorithm was tested on Sentinel-1 datasets from eight different test sites located in Iraq. Four of the test sites along with their corresponding annotations (i.e. ground truth) constitute the training dataset, while the other four test sites are used for evaluation. All the datasets were provided in the framework of a humaritan AI4EO UNOSAT challenge launched in 2019 by Phi-Unet. The results evaluated by the F1 score with values of about 0.75 indicate that the proposed method is able to accurately detect the building footprint.
更多查看译文
关键词
building footprint, machine learning, polarization, synthetic aperture radar
AI 理解论文
溯源树
样例
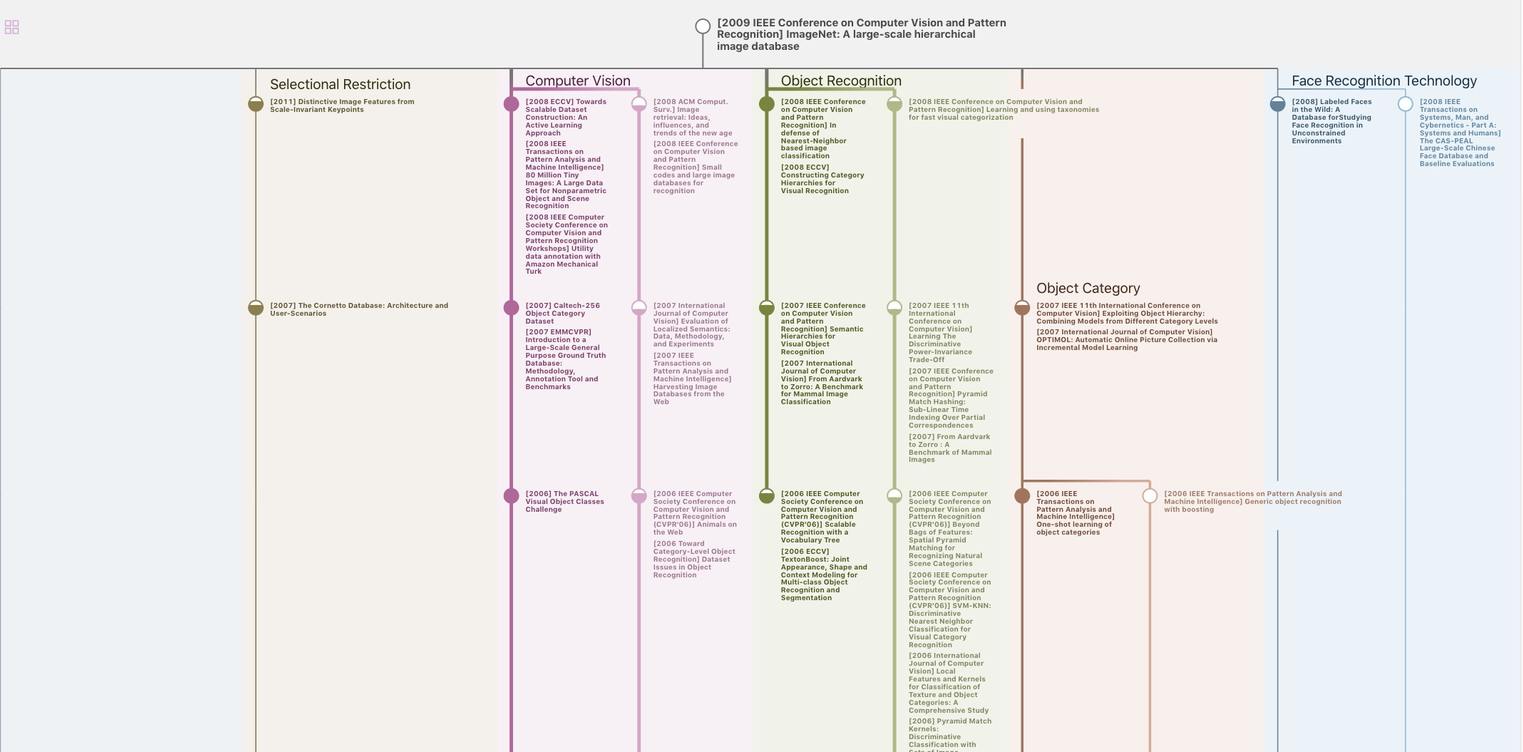
生成溯源树,研究论文发展脉络
Chat Paper
正在生成论文摘要