Modeling Early Indicators Of Grapevine Physiology Using Hyperspectral Imaging And Partial Least Squares Regression (Plsr)
IGARSS 2020 - 2020 IEEE INTERNATIONAL GEOSCIENCE AND REMOTE SENSING SYMPOSIUM(2020)
摘要
In this contribution, we use field-based hyperspectral imaging (HSI) and partial least squares regression (PLSR) to estimate early indicators of grapevine physiological indicators, and analyze identified significant spectral regions for fast and accurate plant health monitoring. HSI and physiological measurements were carried out at two commercial vineyards in California, USA. The PLSR models were developed between reflectance spectra extracted from hyperspectral images and four vine physiological parameters, including stomatal conductance (Gb) photosynthetic CO2 rate (A), intercellular CO2 concentration (C-1) and transpiration rate (E). The results demonstrate PLSR models to predict physiological parameters (R-2 >= 0.6), and the best model was found for G(s) (R-2 = 0.7). The identified significant spectral regions overlap with most commonly used remote sensing stress indicator, suggesting that HSI coupled with PLSR has great potential for upscaling and broader agricultural applications.
更多查看译文
关键词
Hyperspectral imaging, Grapevine, Physiology, Stress, PLSR
AI 理解论文
溯源树
样例
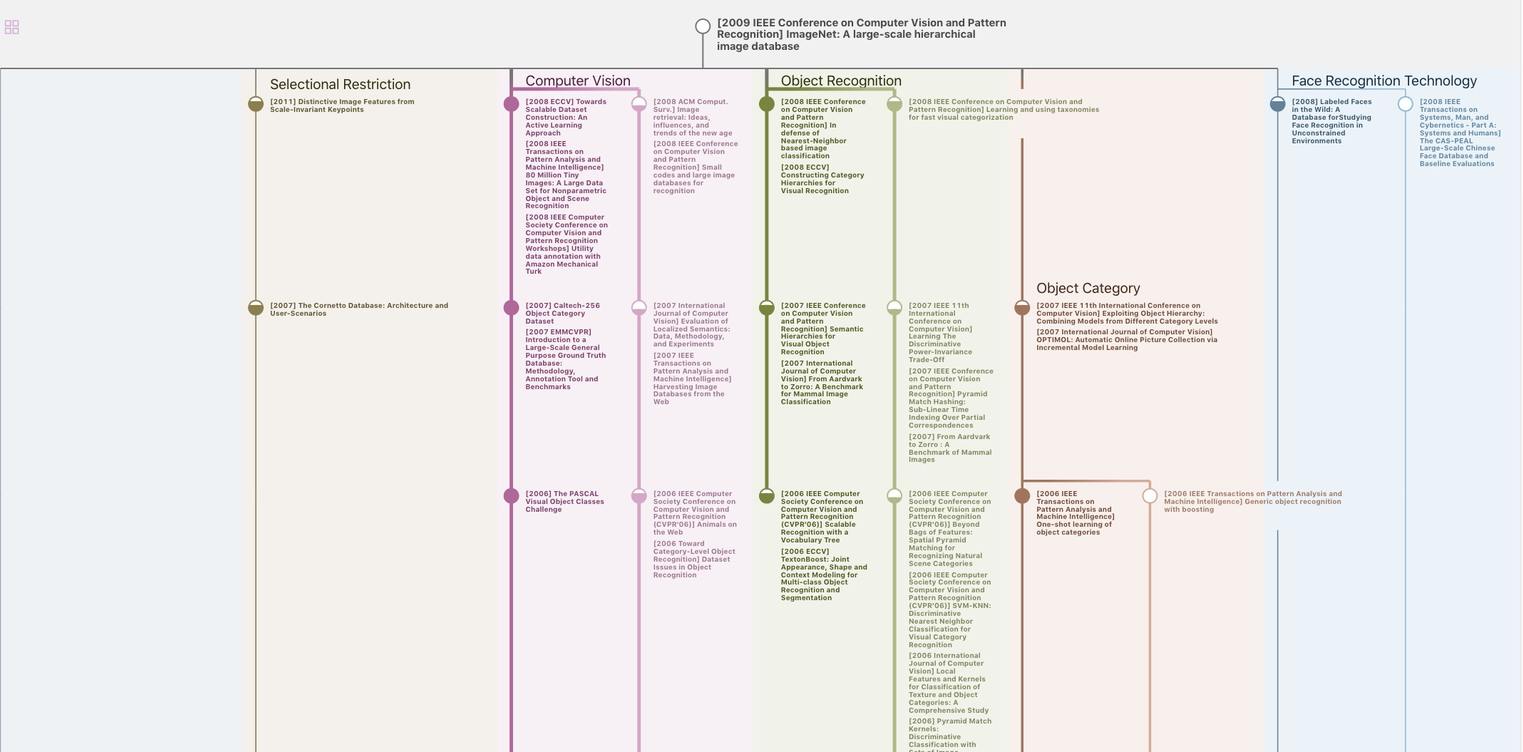
生成溯源树,研究论文发展脉络
Chat Paper
正在生成论文摘要