Predicting Growing Stock Volume of Boreal Forests Using Very Long Time Series of Sentinel-1 Data
IGARSS 2020 - 2020 IEEE INTERNATIONAL GEOSCIENCE AND REMOTE SENSING SYMPOSIUM(2020)
摘要
In this study, we assess the potential of long time series of Sentinel-1 SAR data in forest growing stock volume (GSV) estimation. The study site with 17,762 forest stands is located near the Hyytiälä forestry field station in Finland and represents the boreal coniferous forest. Altogether 96 images spanning more than three years of observations have been studied using linear and random forest regression approaches. Our analysis demonstrates considerable decrease in the prediction errors of GSV as the the number of input scenes increases. The use of feature extraction and dimensionality reduction techniques allows to achieve to nearly optimal performance already with 10 scenes. While the GSV prediction errors using individual Sentinel-1 scenes varied considerably from 86 to 93 m
3
/ha, the prediction accuracy with combined scenes improved to 76 m
3
/ha (44.9%) RMSE.
更多查看译文
关键词
synthetic aperture radar, forest stem volume, boreal forest, Sentinel-1, support vector regression, random forest regression
AI 理解论文
溯源树
样例
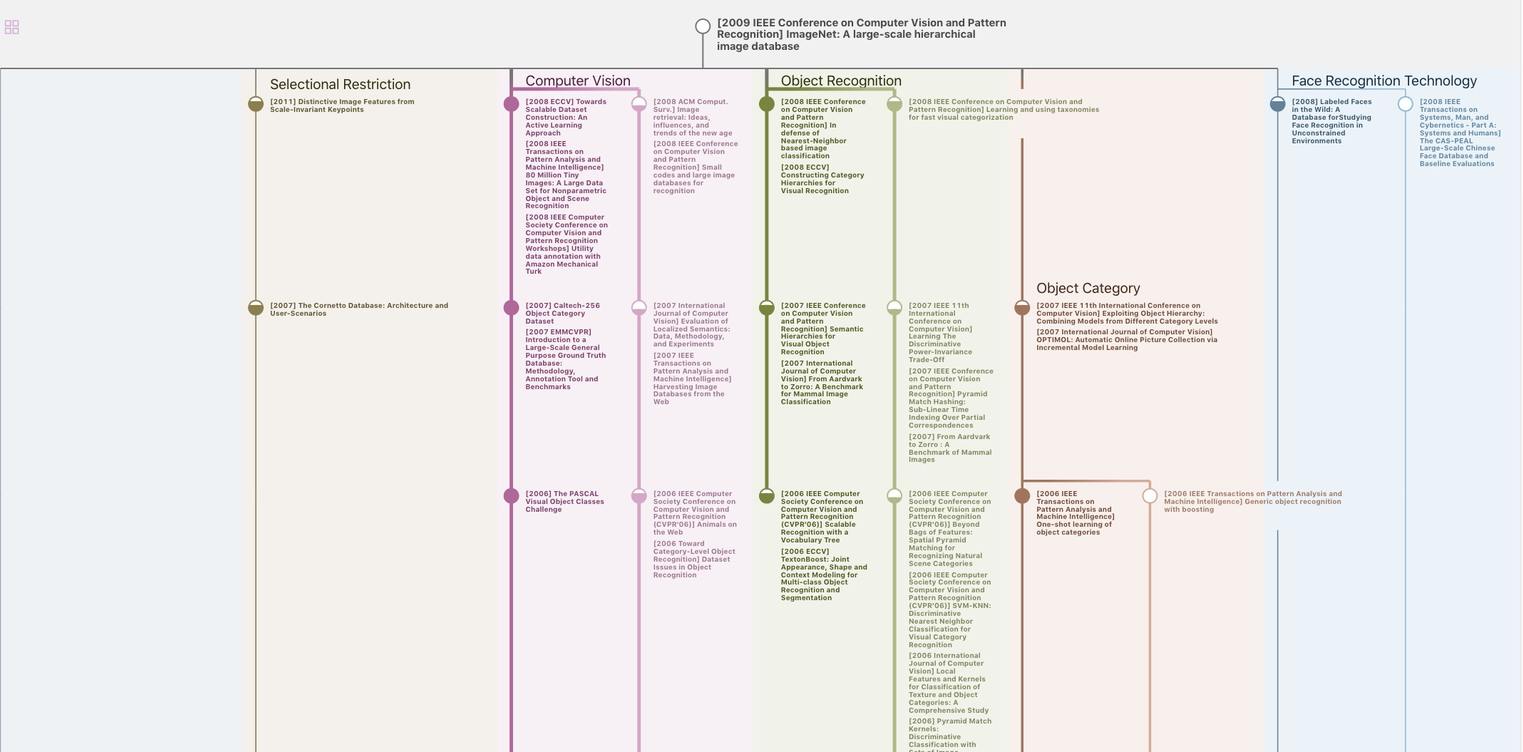
生成溯源树,研究论文发展脉络
Chat Paper
正在生成论文摘要