Sugarcane Yield Mapping Using High-Resolution Imagery Data And Machine Learning Technique
REMOTE SENSING(2021)
摘要
Yield maps provide essential information to guide precision agriculture (PA) practices. Yet, on-board yield monitoring for sugarcane can be challenging. At the same time, orbital images have been widely used for indirect crop yield estimation for many crops like wheat, corn, and rice, but not for sugarcane. Due to this, the objective of this study is to explore the potential of multi-temporal imagery data as an alternative for sugarcane yield mapping. The study was based on developing predictive sugarcane yield models integrating time-series orbital imaging and a machine learning technique. A commercial sugarcane site was selected, and Sentinel-2 images were acquired from the beginning of the ratoon sprouting until harvesting of two consecutive cropping seasons. The predictive yield models RF (Random forest) and MLR (Multiple Linear Regression) were developed using orbital images and yield maps generated by a commercial sensor-system on harvesting. Original yield data were filtered and interpolated with the same spatial resolution of the orbital images. The entire dataset was divided into training and testing datasets. Spectral bands, especially the near-infrared at tillering crop stage showed greater contribution to predicting sugarcane yield than the use of derived spectral vegetation indices. The Root Mean Squared Error (RMSE) obtained for the RF regression based on multiple spectral bands was 4.63 Mg ha(-1) with an R-2 of 0.70 for the testing dataset. Overall, the RF regression had better performance than the MLR to predict sugarcane yield.
更多查看译文
关键词
orbital images, precision agriculture, remote sensing, vegetation index
AI 理解论文
溯源树
样例
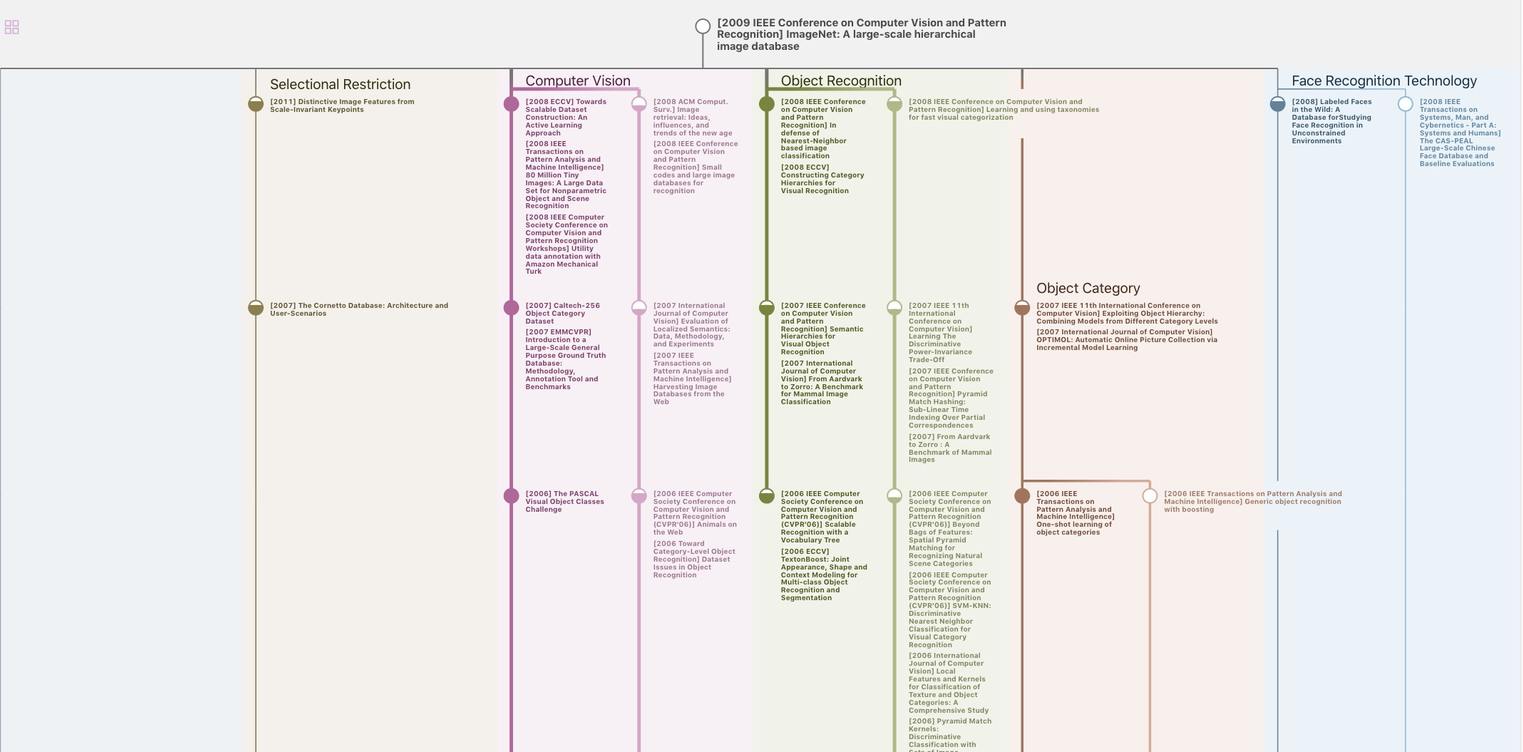
生成溯源树,研究论文发展脉络
Chat Paper
正在生成论文摘要