Bridging factor and sparse models
arxiv(2023)
摘要
Factor and sparse models are widely used to impose a low-dimensional structure in high-dimensions. However, they are seemingly mutually exclusive. We propose a lifting method that combines the merits of these two models in a supervised learning methodology that allows for efficiently exploring all the information in high-dimensional datasets. The method is based on a flexible model for high-dimensional panel data with observable and/or latent common factors and idiosyncratic components. The model is called the factor-augmented regression model. It includes principal components and sparse regression as specific models, significantly weakens the crosssectional dependence, and facilitates model selection and interpretability. The method consists of several steps and a novel test for (partial) covariance structure in high dimensions to infer the remaining cross-section dependence at each step. We develop the theory for the model and demonstrate the validity of the multiplier bootstrap for testing a high-dimensional (partial) covariance structure. A simulation study and applications support the theory.
更多查看译文
关键词
Factor models, penalized least-squares, high-dimensional inference, (partial) covari-ance structure, prediction, machine learning, supervised learning
AI 理解论文
溯源树
样例
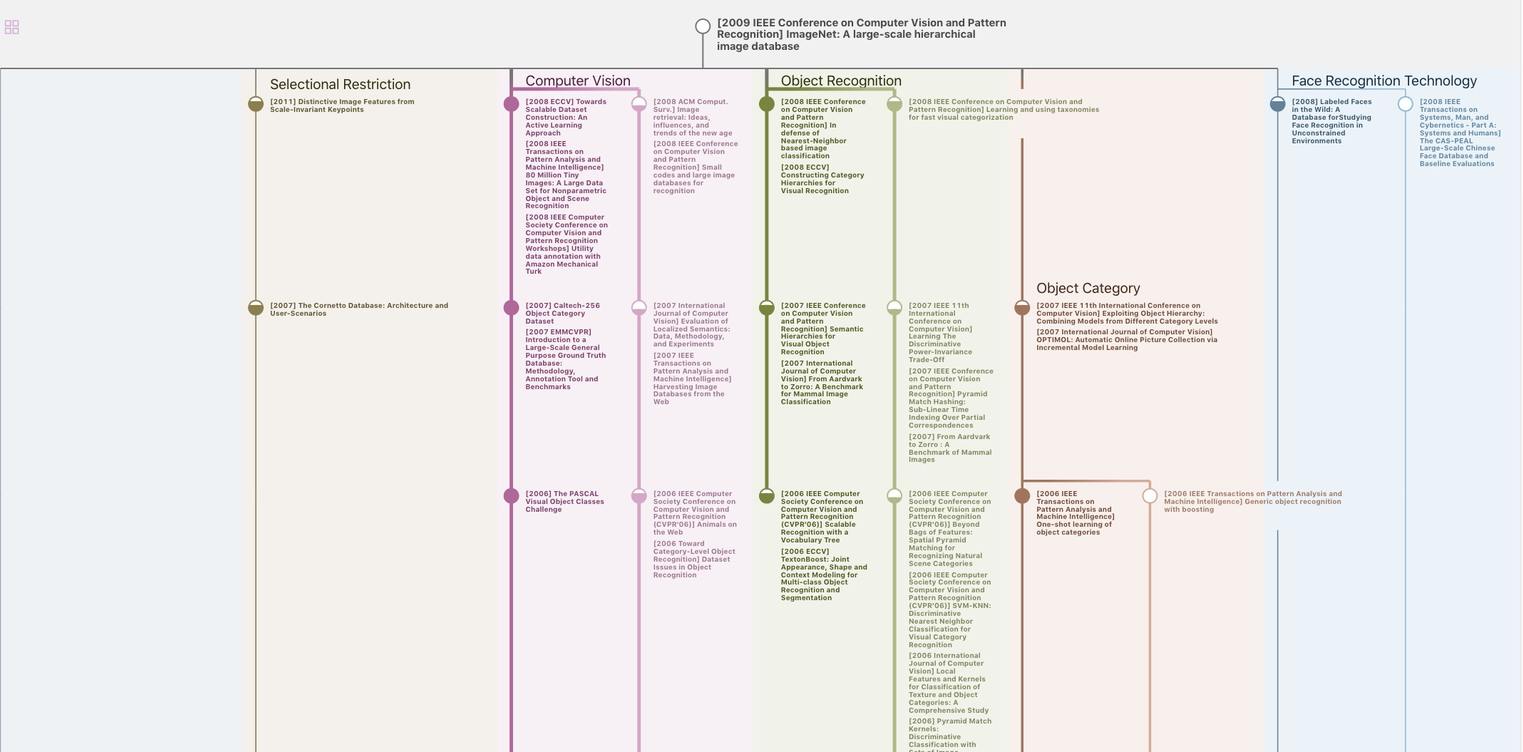
生成溯源树,研究论文发展脉络
Chat Paper
正在生成论文摘要