A Probabilistic Function To Model The Relationship Between Quality Of Chest Compressions And The Physiological Response For Patients In Cardiac Arrest
2020 COMPUTING IN CARDIOLOGY(2020)
摘要
Cardiopulmonary resuscitation quality (CPRQ) parameters can be derived from electric signals obtained during resuscitation. We propose to model a probabilistic relationship between CPRQ parameters and the physiological response as judged by ECG-features, to guide therapy in a clinical context.A total of 821 compression sequences were extracted from 394 out-of-hospital resuscitation episodes. Sequences were categorized as effective if the post sequence cardiac rhythm had better prognosis than the pre-sequence rhythm by a positive difference, otherwise as non effective if the difference was negative. CPRQ parameters related to depth and rate were calculated. Three alternative approaches were designed for the binary classifier based on the CPRQ parameters: quadratic discriminant analysis (QDA), logistic regression (LR) and artificial neural networks (ANN). The positive class discriminant function defined the probability of effective compressions (Pec). The classification accuracies were around 0.6 for all three models. The highest probability estimates of effective chest compressions corresponded to the depth (5-6 cm) and rate (100-120 min-1) currently recommended in the CPR guidelines.We have proposed a novel method to relate the quality of chest compressions to the physiologic response to CPR.
更多查看译文
关键词
probabilistic function,cardiac arrest,cardiopulmonary resuscitation quality parameters,electric signals,out-of-hospital resuscitation episodes,post sequence cardiac rhythm,logistic regression,chest compressions,presequence rhythm,compression sequences,ECG,feature extraction,quadratic discriminant analysis,artificial neural networks
AI 理解论文
溯源树
样例
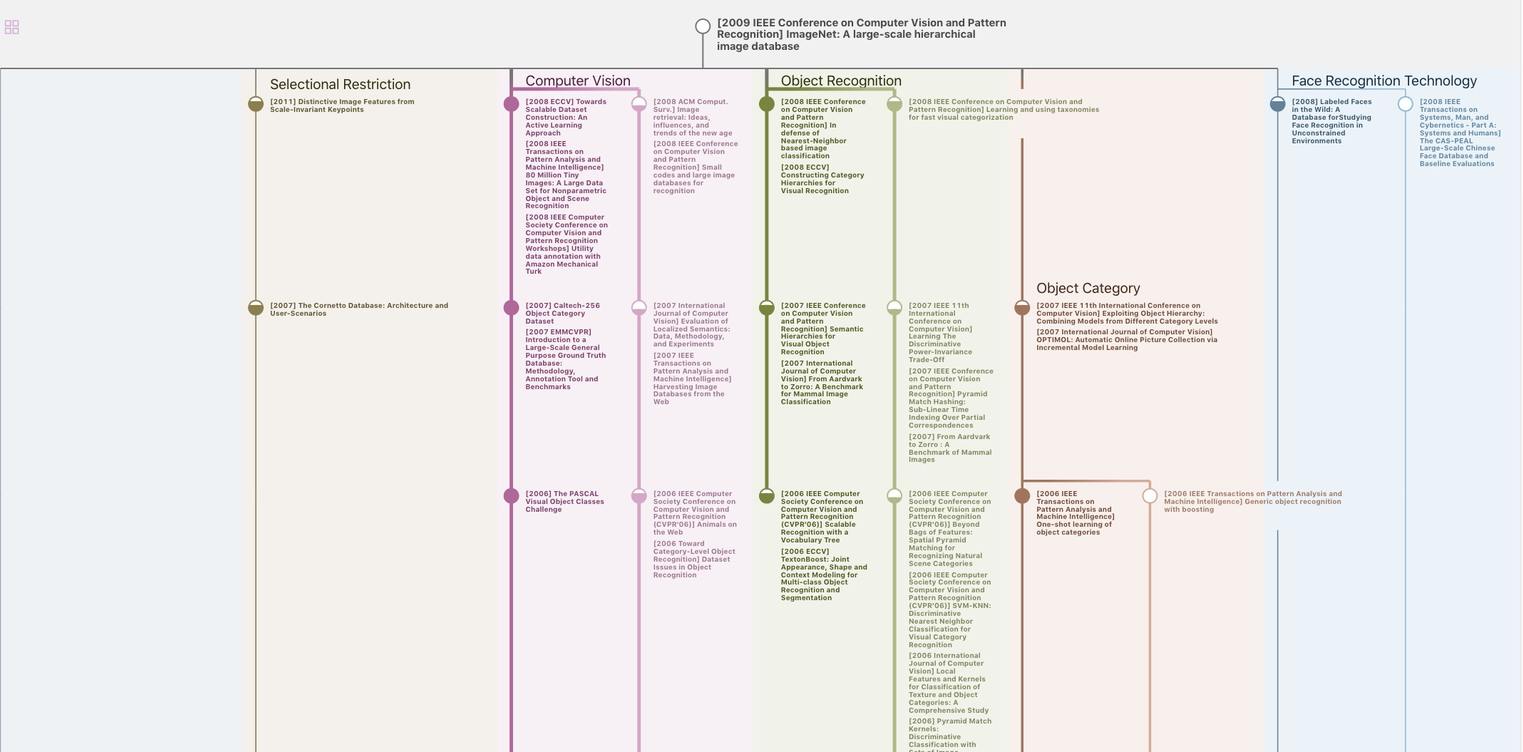
生成溯源树,研究论文发展脉络
Chat Paper
正在生成论文摘要