Predicting The Sugarcane Yield In Real-Time By Harvester Engine Parameters And Machine Learning Approaches
COMPUTERS AND ELECTRONICS IN AGRICULTURE(2021)
摘要
Information regarding sugarcane yield is the starting point for the application of Precision Agriculture (PA) strategies to the management of sugarcane fields. Over the years, many sugarcane yield monitoring approaches have been developed and tested, but its use is still limited. One option is to use data generated by the sugarcane harvester provided in the controller area network (CAN), which allows getting the messages related to the harvester operation. Our goal was to train and to test random forest (RF), multiple linear regression (MLR), and artificial neural network (ANN) using CAN data available on the on-board computer of the harvester. All predictive models were trained and tested using the main engine parameters: fuel consumption, engine rotation, engine power, and specific fuel consumption (SFC) as data input. A scale yield monitor was installed on the harvester elevator to provide the georeferenced yield data. The yield prediction accuracy of the predictive models using the four parameters was compared. All models followed the observed sugarcane yield and recognized the changes over the time of data collection. The MLR and ANN models have a higher error of prediction in the extreme values of yield. Over the testing time, the ANN underestimated the sugarcane yield, and the RF prediction by the engine parameters of the harvester was coming closest to the observed yield. The best results for predicting sugarcane yield by engine parameters were obtained from the RF model with a mean absolute percent error (MAPE) of 5.6%, and root mean square error (RMSE) of 7.0 Mg ha(-1). The MLR and ANN models have a MAPE of 7.8% and 5.6%, respectively. All the variables were necessary for a better performance of the models; however, SFC was the most important variable for predicting sugarcane yield.
更多查看译文
关键词
Artificial intelligence, CAN data, Precision agriculture, Yield monitor
AI 理解论文
溯源树
样例
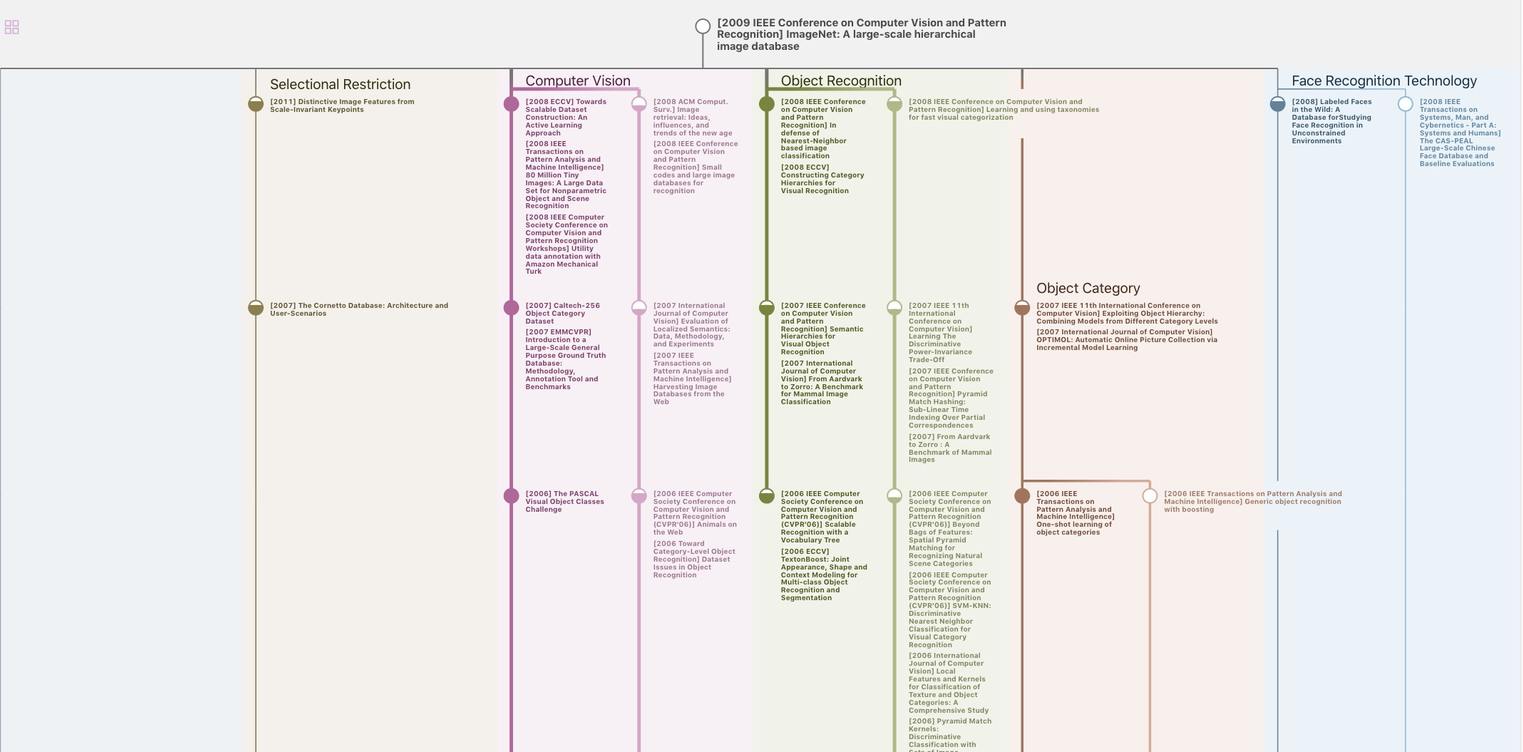
生成溯源树,研究论文发展脉络
Chat Paper
正在生成论文摘要