E(n) Equivariant Graph Neural Networks
INTERNATIONAL CONFERENCE ON MACHINE LEARNING, VOL 139(2021)
摘要
This paper introduces a new model to learn graph neural networks equivariant to rotations, translations, reflections and permutations called E(n)-Equivariant Graph Neural Networks (EGNNs). In contrast with existing methods, our work does not require computationally expensive higher-order representations in intermediate layers while it still achieves competitive or better performance. In addition, whereas existing methods are limited to equivariance on 3 dimensional spaces, our model is easily scaled to higher-dimensional spaces. We demonstrate the effectiveness of our method on dynamical systems modelling, representation learning in graph autoencoders and predicting molecular properties.
更多查看译文
关键词
neural networks,graph
AI 理解论文
溯源树
样例
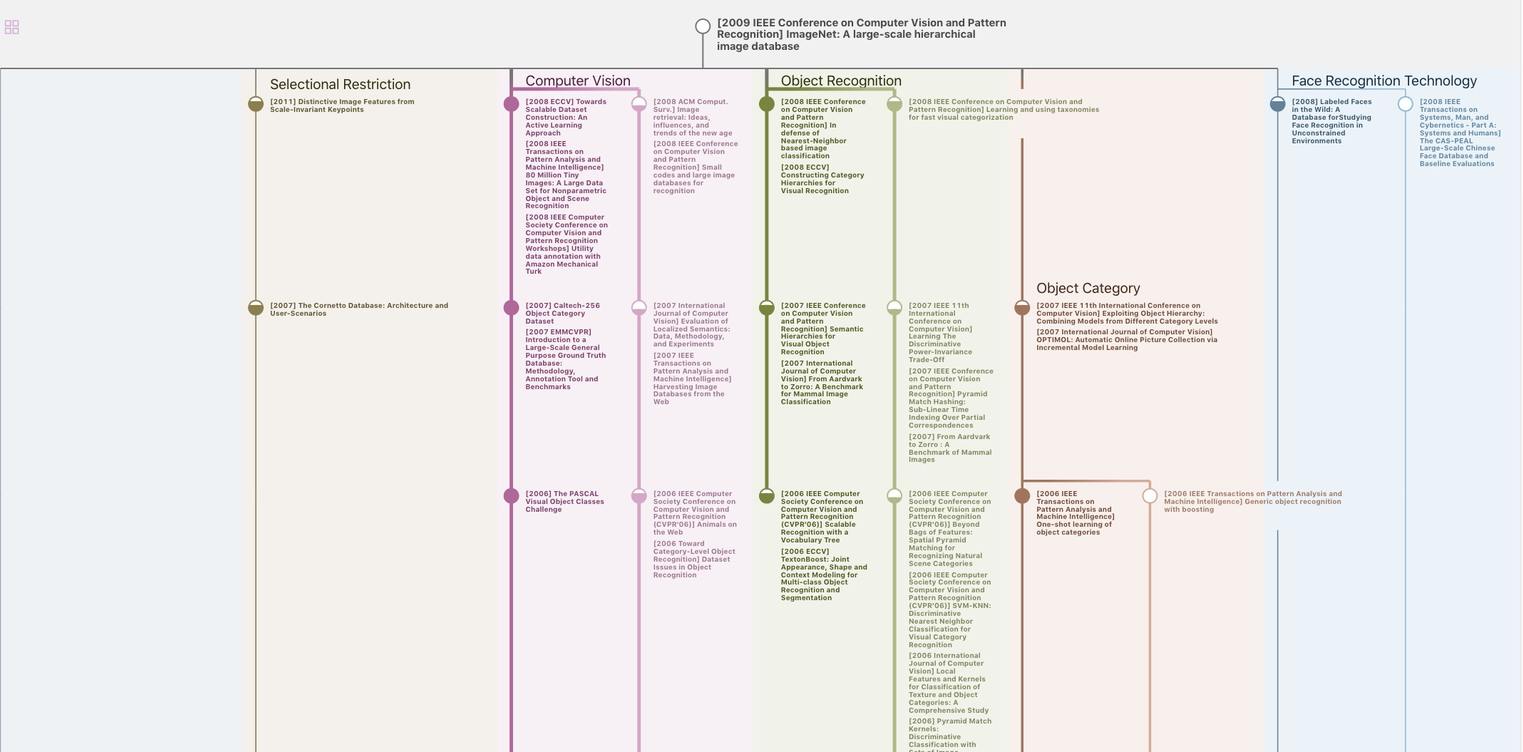
生成溯源树,研究论文发展脉络
Chat Paper
正在生成论文摘要