Deep Learning for Suicide and Depression Identification with Unsupervised Label Correction
ARTIFICIAL NEURAL NETWORKS AND MACHINE LEARNING, ICANN 2021, PT V(2021)
摘要
Early detection of suicidal ideation in depressed individuals can allow for adequate medical attention and support, which can be life-saving. Recent NLP research focuses on classifying, from given text, if an individual is suicidal or clinically healthy. However, there have been no major attempts to differentiate between depression and suicidal ideation, which is a separate and important clinical challenge. Due to the scarce availability of EHR data, suicide notes, or other verified sources, web query data has emerged as a promising alternative. Online sources, such as Reddit, allow for anonymity, prompting honest disclosure of symptoms, making it a plausible source even in a clinical setting. However, online datasets also result in inherent noise in web-scraped labels, which necessitates a noise-removal process to improve performance. Thus, we propose SDCNL, a deep neural network approach for suicide versus depression classification. We utilize online content to train our algorithm, and to verify and correct noisy labels, we propose a novel unsupervised label correction method which, unlike previous work, does not require prior noise distribution information. Our extensive experimentation with various deep word embedding models and classifiers display strong performance of SDCNL as a new clinical application for a challenging problem (We make our supplemental, dataset, web-scraping script, and code (with hyperparameters) available at https://github.com/ayaanzhaque/SDCNL).
更多查看译文
关键词
Suicide/Depression, Noisy labels, Deep learning, Online content, Natural Language Processing, Unsupervised learning
AI 理解论文
溯源树
样例
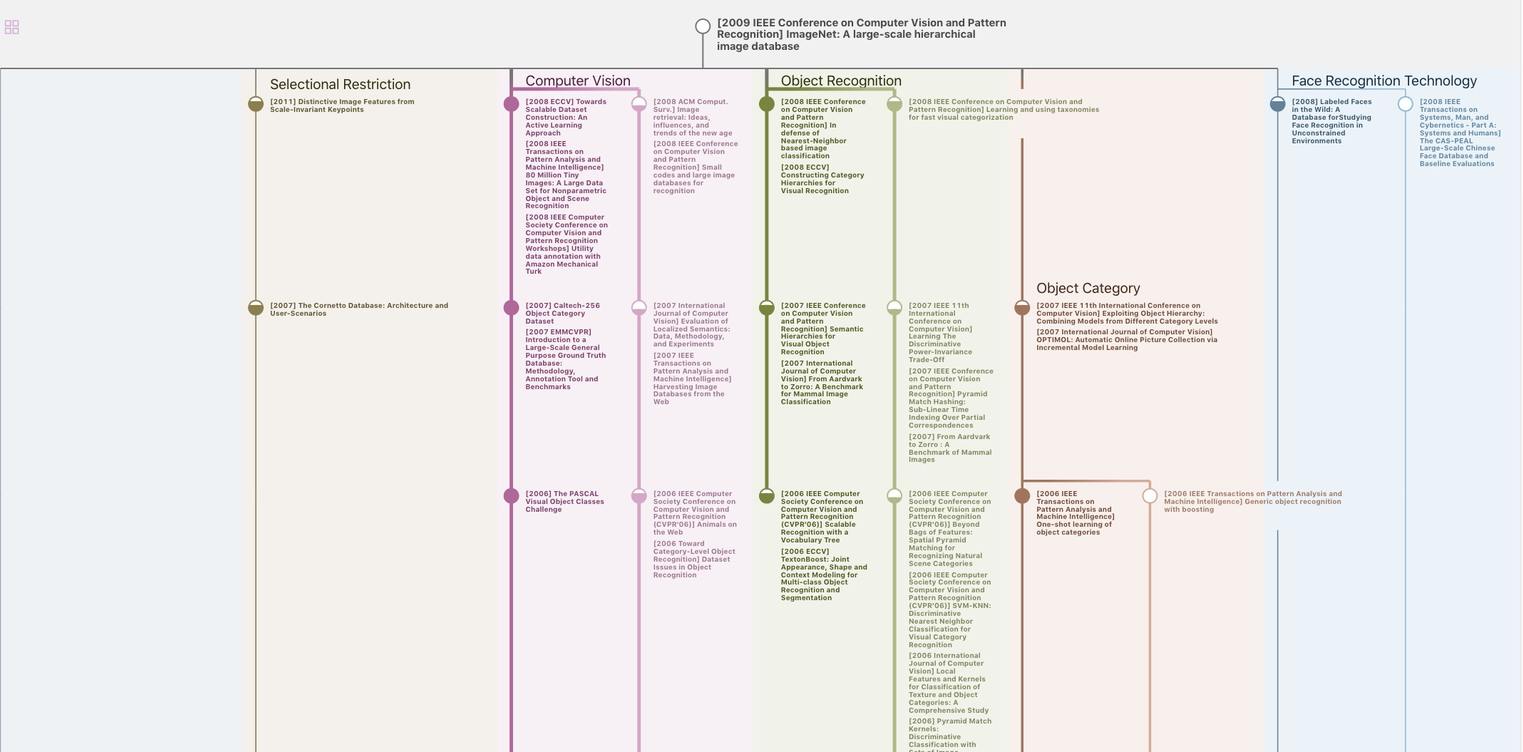
生成溯源树,研究论文发展脉络
Chat Paper
正在生成论文摘要