Community Detection with Local Metric Learning
2020 IEEE International Conference on Data Mining (ICDM)(2020)
摘要
Community detection in attributed networks has gained growing attention in recent years due to the booming of network data with both topological structure and attributes of nodes. To date, numerous algorithms have been proposed to leverage both kinds of information to yield high-quality communities based on homophily assumption (i.e., nodes are likely to link with those who share similar attributes). However, these approaches tend to focus on consistent information only and fail to consider the heterogeneity between topology and attributes. In light of the problem, we propose a new algorithm called CDLM (Community Detection via Local Metric learning) for attributed networks. The key point is to combine topological structure and node attributes in local metric space and perform community detection and local metric learning iteratively. With such a strategy, the learned local distance measures will benefit the performance of community detection, and in turn, the identification of intrinsic community structure helps to eliminate the negative effects of noisy edges in learning local metrics. Notably, homogeneity and heterogeneity between topological structure and node attributes are simultaneously considered to boost the performance of community detection. Experimental results on both synthetic and real-world networks have demonstrated the effectiveness of the proposed CDLM algorithm.
更多查看译文
关键词
Community Detection,Attributed Network,Metric Learning
AI 理解论文
溯源树
样例
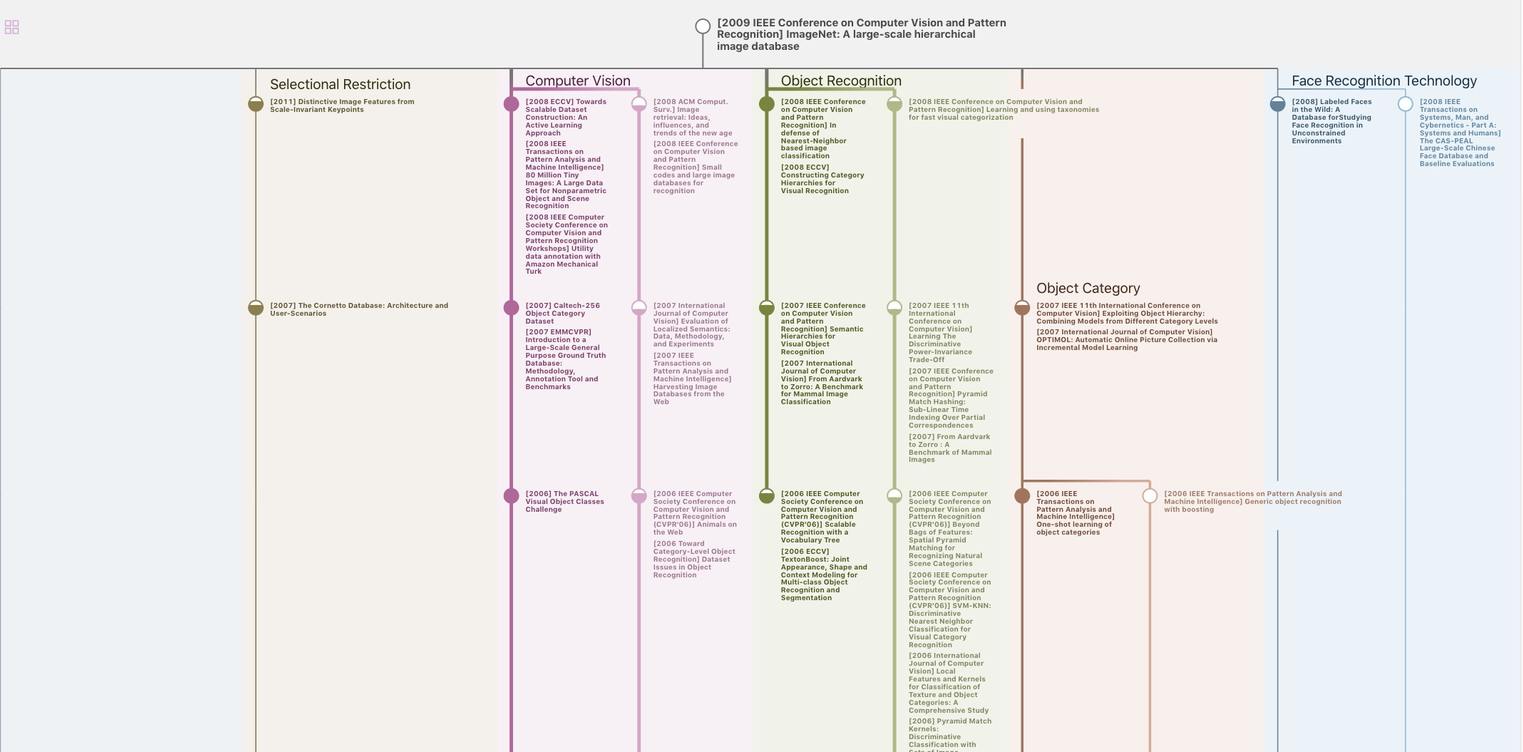
生成溯源树,研究论文发展脉络
Chat Paper
正在生成论文摘要