Divide-and-Conquer MCMC for Multivariate Binary Data
arxiv(2021)
摘要
We analyze a large database of de-identified Medicare Advantage claims from a single large US health insurance provider, where the number of individuals available for analysis are an order of magnitude larger than the number of potential covariates. This type of data, dubbed `tall data', often does not fit in memory, and estimating parameters using traditional Markov Chain Monte Carlo (MCMC) methods is a computationally infeasible task. We show how divide-and-conquer MCMC, which splits the data into disjoint subsamples and runs a MCMC algorithm on each sample in parallel before combining results, can be used with a multivariate probit factor model. We then show how this approach can be applied to large medical datasets to provide insights into questions of interest to the medical community. We also conduct a simulation study, comparing two posterior combination algorithms with a mean-field stochastic variational approach, showing that divide-and-conquer MCMC should be preferred over variational inference when estimating the latent correlation structure between binary responses is of primary interest.
更多查看译文
AI 理解论文
溯源树
样例
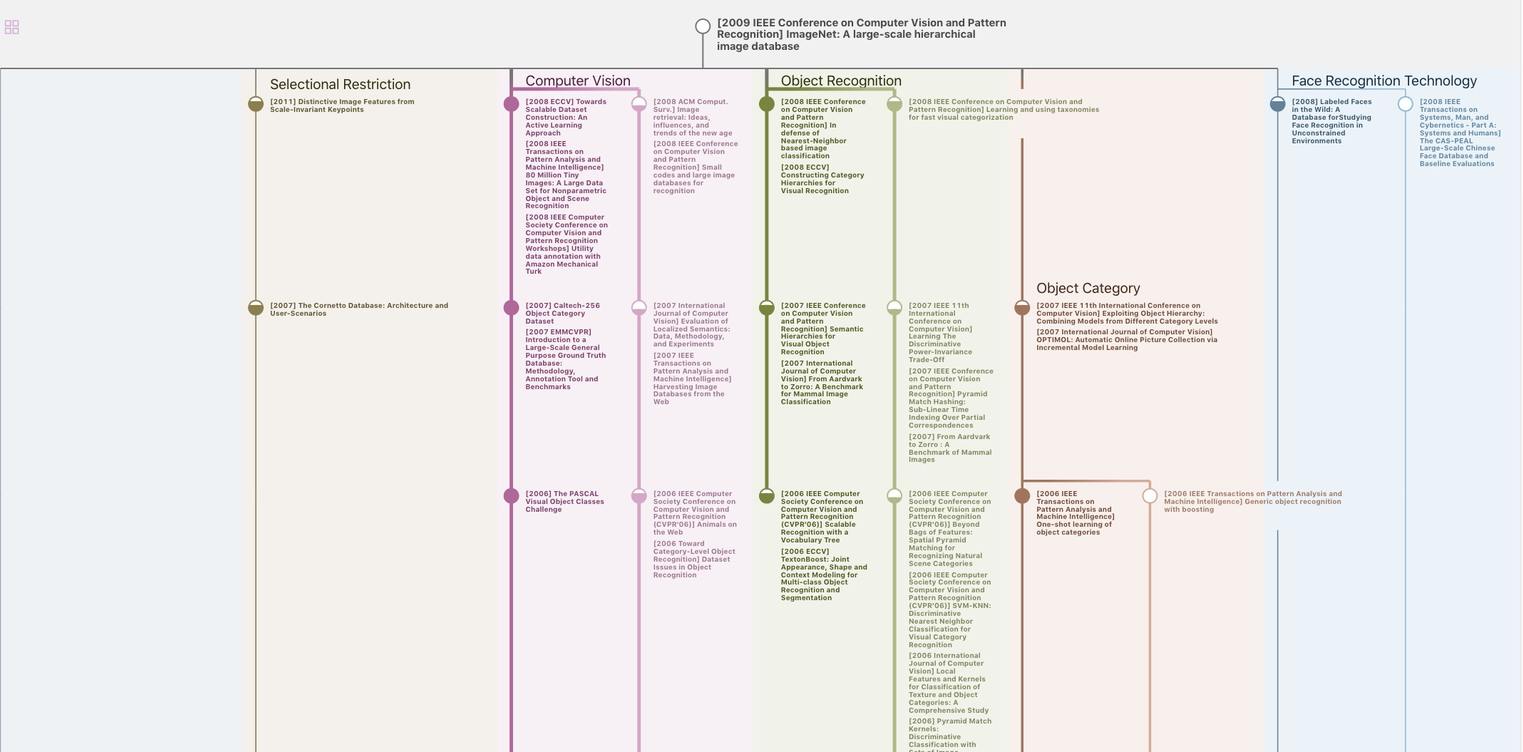
生成溯源树,研究论文发展脉络
Chat Paper
正在生成论文摘要