Informational Entropy Threshold as a Physical Mechanism for Explaining Tree-like Decision Making in Humans.
Entropy (Basel, Switzerland)(2022)
摘要
While approaches based on physical grounds (such as the drift-diffusion model-DDM) have been exhaustively used in psychology and neuroscience to describe perceptual decision making in humans, similar approaches to complex situations, such as sequential (tree-like) decisions, are still scarce. For such scenarios that involve a reflective prospection of future options, we offer a plausible mechanism based on the idea that subjects can carry out an internal computation of the uncertainty about the different options available, which is computed through the corresponding Shannon entropy. When the amount of information gathered through sensory evidence is enough to reach a given threshold in the entropy, this will trigger the decision. Experimental evidence in favor of this entropy-based mechanism was provided by exploring human performance during navigation through a maze on a computer screen monitored with the help of eye trackers. In particular, our analysis allows us to prove that (i) prospection is effectively used by humans during such navigation tasks, and an indirect quantification of the level of prospection used is attainable; in addition, (ii) the distribution of decision times during the task exhibits power-law tails, a feature that our entropy-based mechanism is able to explain, unlike traditional (DDM-like) frameworks.
更多查看译文
关键词
decision making,entropy,eye-tracking
AI 理解论文
溯源树
样例
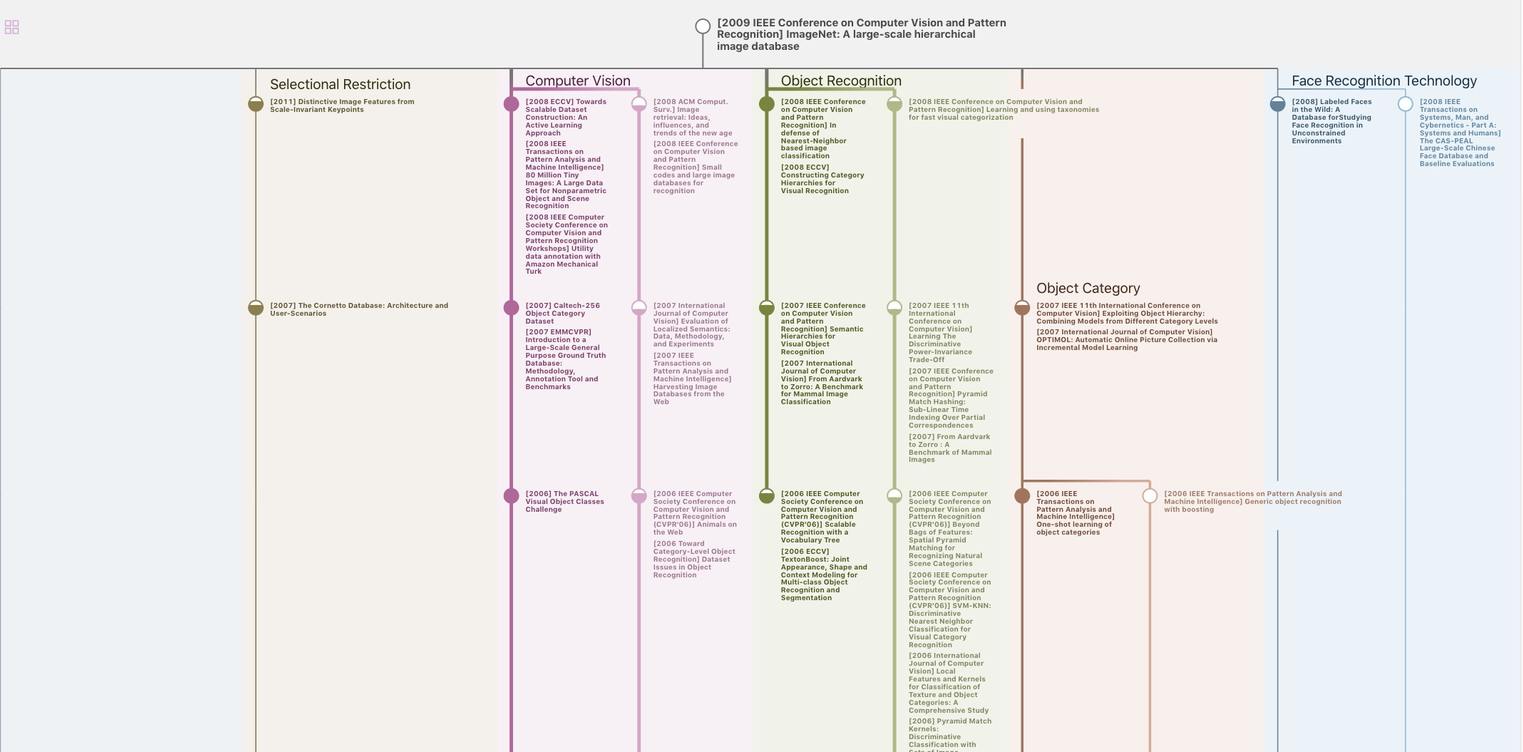
生成溯源树,研究论文发展脉络
Chat Paper
正在生成论文摘要