Learning to Detect Salient Object With Multi-Source Weak Supervision
IEEE Transactions on Pattern Analysis and Machine Intelligence(2022)
摘要
High-cost pixel-level annotations makes it appealing to train saliency detection models with weak supervision. However, a single weak supervision source hardly contain enough information to train a well-performing model. To this end, we introduce a unified two-stage framework to learn from category labels, captions, web images and unlabeled images. In the first stage, we design a classification network (CNet) and a caption generation network (PNet), which learn to predict object categories and generate captions, respectively, meanwhile highlights the potential foreground regions. We present an attention transfer loss to transmit supervisions between two tasks and an attention coherence loss to encourage the networks to detect generally salient regions instead of task-specific regions. In the second stage, we create two complementary training datasets using CNet and PNet, i.e., natural image dataset with noisy labels for adapting saliency prediction network (SNet) to natural image input, and synthesized image dataset by pasting objects on background images for providing SNet with accurate ground-truth. During the testing phases, we only need SNet to predict saliency maps. Experiments indicate the performance of our method compares favorably against unsupervised, weakly supervised methods and even some supervised methods.
更多查看译文
关键词
Saliency,salient object detection,weak supervision
AI 理解论文
溯源树
样例
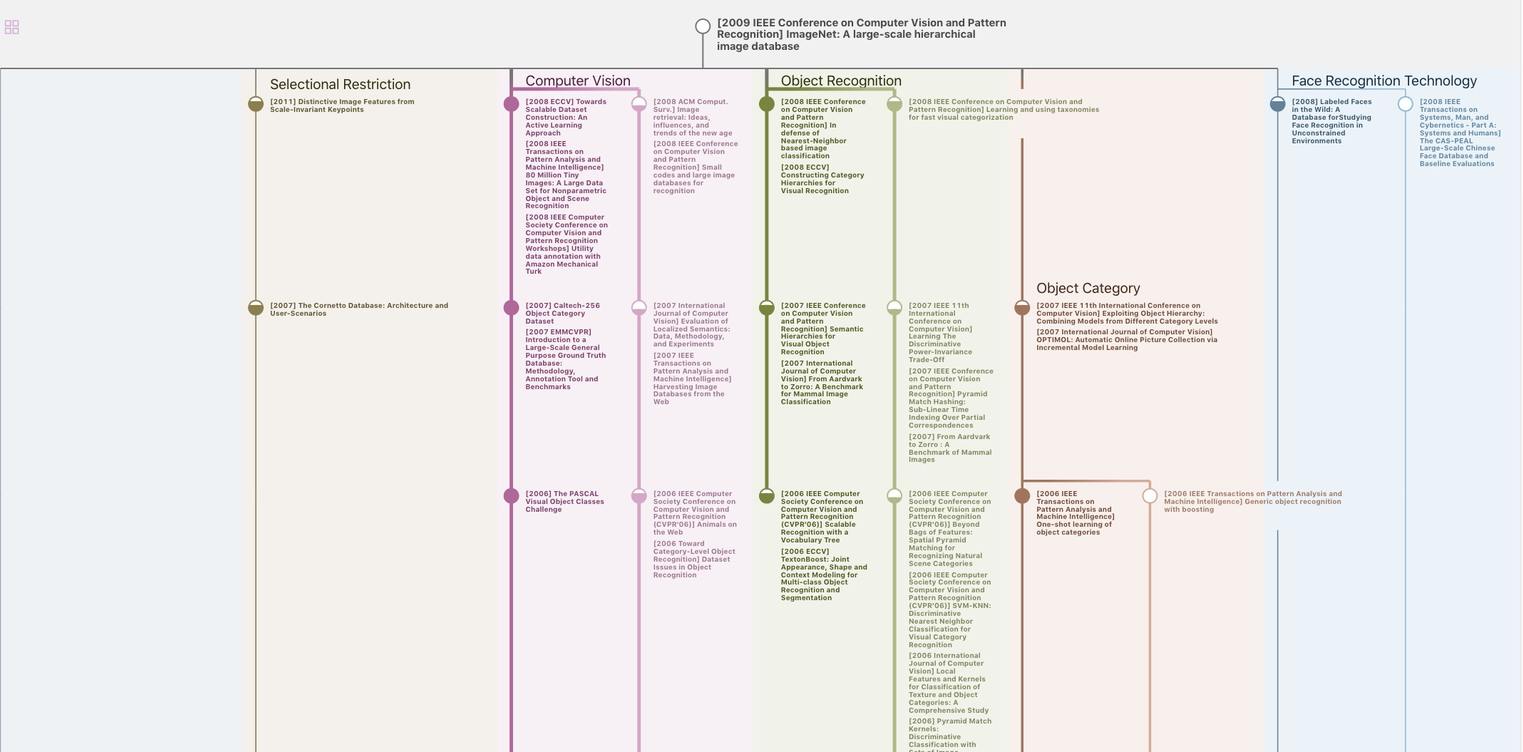
生成溯源树,研究论文发展脉络
Chat Paper
正在生成论文摘要