Vision Global Localization with Semantic Segmentation and Interest Feature Points.
IROS(2020)
摘要
In this work, we present a vision-only global localization architecture for autonomous vehicle applications, and achieves centimeter-level accuracy and high robustness in various scenarios. We first apply pixel-wise segmentation to the front-view mono camera and extract the semantic features, e.g. pole-like objects, lane markings, and curbs, which are robust to illumination, viewing angles and seasonal changes. For the scenes without enough semantic information, we extract interest feature points on static backgrounds, such as ground surface and buildings, assisted by our semantic segmentation. We create the visual global map with semantic feature map layers extracted from LiDAR point-cloud semantic map and the point feature map layer built with a fixed-pose SFM. A lumped Levenberg-Marquardt optimization solver is then applied to minimize the cost from two types of observations. We further evaluate the accuracy and robustness of our method with road tests on Alibaba’s autonomous delivery vehicles in multiple scenarios as well as a KAIST urban dataset.
更多查看译文
关键词
vision global localization,semantic segmentation,interest feature points,vision-only global localization architecture,autonomous vehicle applications,centimeter-level accuracy,pixel-wise segmentation,front-view mono camera,pole-like objects,lane markings,semantic information,ground surface,buildings,visual global map,semantic feature map layers,LiDAR point-cloud semantic map,point feature map layer,Alibaba's autonomous delivery vehicles
AI 理解论文
溯源树
样例
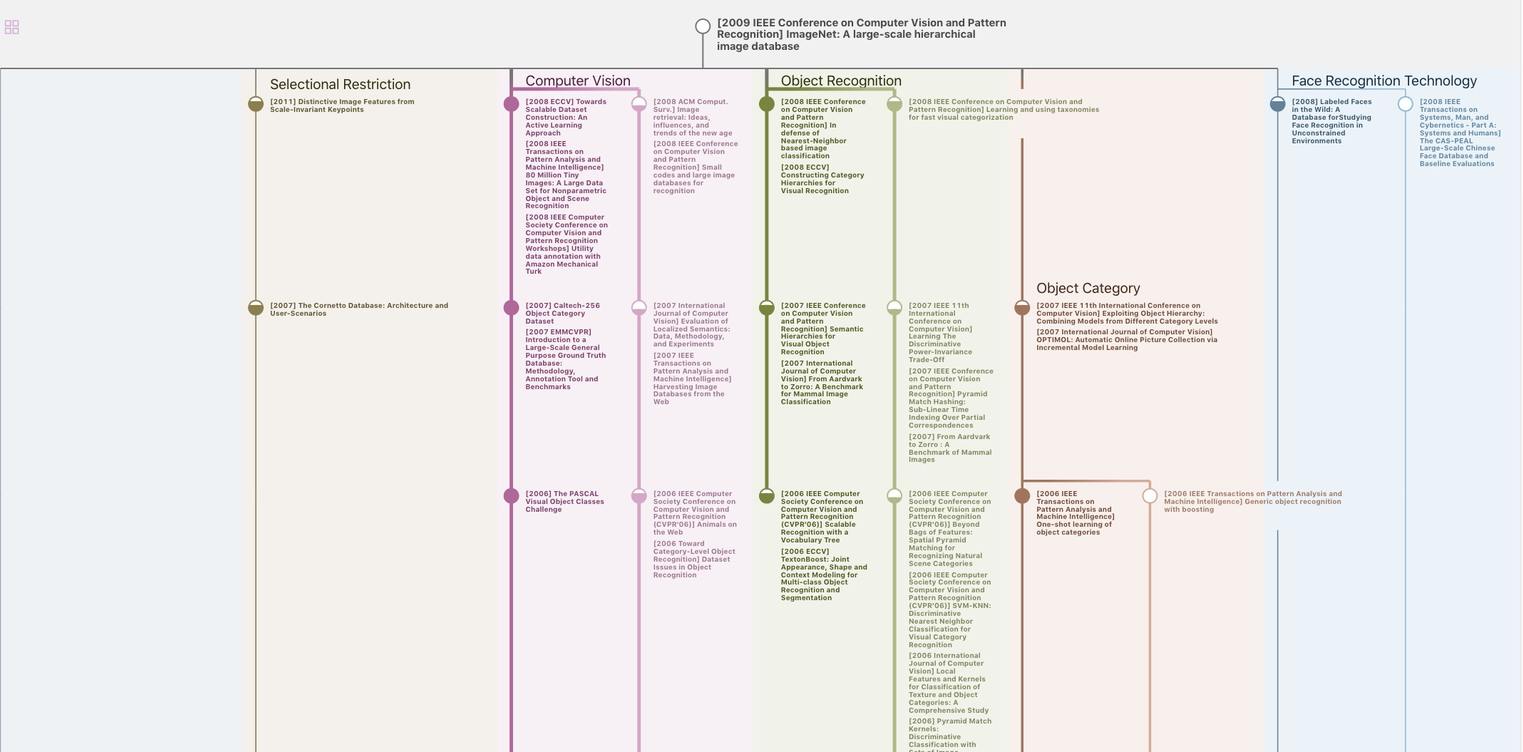
生成溯源树,研究论文发展脉络
Chat Paper
正在生成论文摘要