Configuration Space Decomposition for Learning-based Collision Checking in High-DOF Robots.
IROS(2020)
摘要
Motion planning for robots of high degrees-of-freedom (DOFs) is an important problem in robotics with sampling-based methods in configuration space $\\mathcal{C}$ as one popular solution. Recently, machine learning methods have been introduced into sampling-based motion planning methods, which train a classifier to distinguish collision free subspace from in-collision subspace in $\\mathcal{C}$. In this paper, we propose a novel configuration space decomposition method and show two nice properties resulted from this decomposition. Using these two properties, we build a composite classifier that works compatibly with previous machine learning methods by using them as the elementary classifiers. Experimental results are presented, showing that our composite classifier outperforms state-of-the-art single-classifier methods by a large margin. A real application of motion planning in a multi-robot system in plant phenotyping using three UR5 robotic arms is also presented.
更多查看译文
关键词
composite classifier,elementary classifiers,multirobot system,UR5 robotic arms,learning-based collision checking,degrees-of-freedom,DOFs,robotics,sampling-based methods,configuration space,machine learning,sampling-based motion planning,collision free subspace,in-collision subspace,configuration space decomposition
AI 理解论文
溯源树
样例
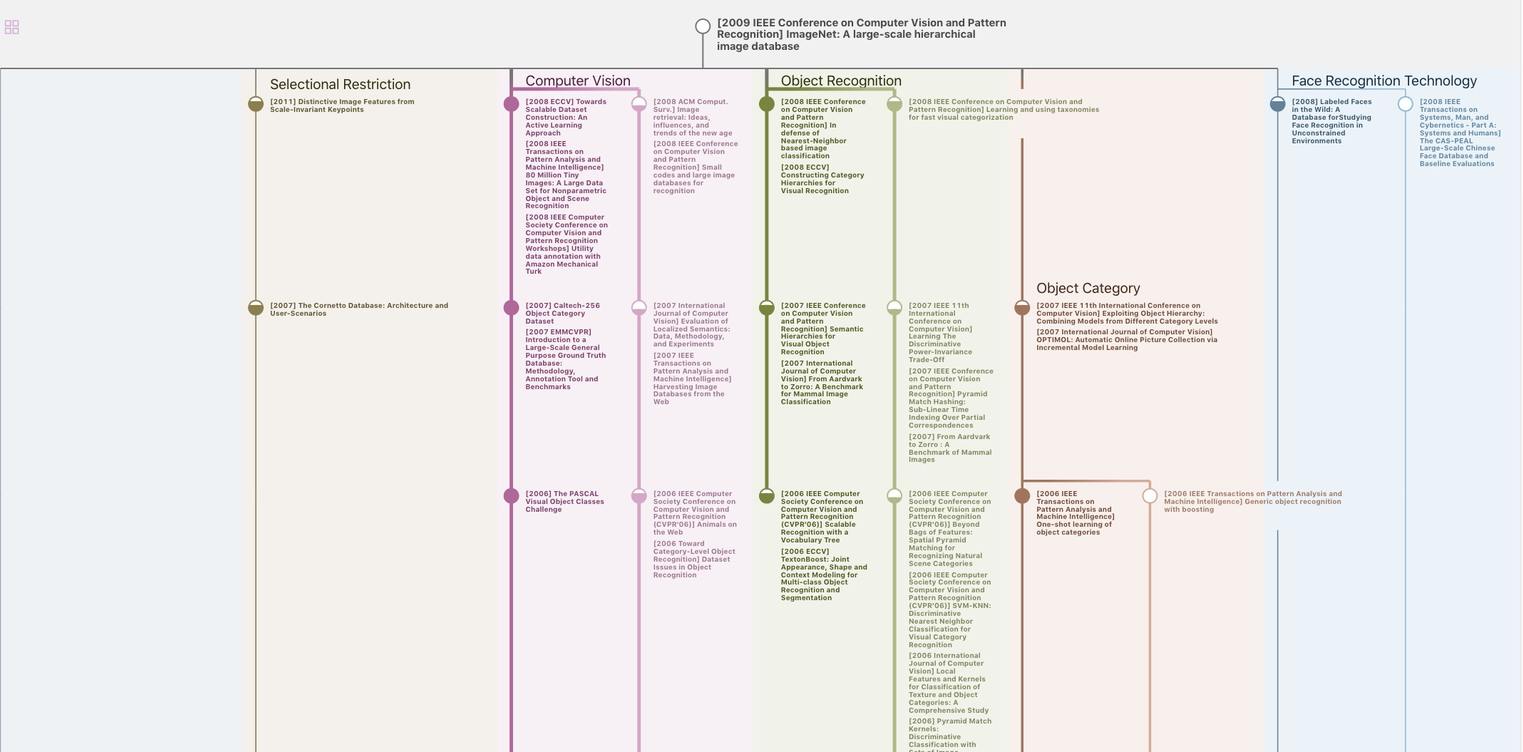
生成溯源树,研究论文发展脉络
Chat Paper
正在生成论文摘要