Complex Momentum for Learning in Games
arxiv(2021)
摘要
We generalize gradient descent with momentum for learning in differentiable games to have complex-valued momentum. We give theoretical motivation for our method by proving convergence on bilinear zero-sum games for simultaneous and alternating updates. Our method gives real-valued parameter updates, making it a drop-in replacement for standard optimizers. We empirically demonstrate that complex-valued momentum can improve convergence in adversarial games - like generative adversarial networks - by showing we can find better solutions with an almost identical computational cost. We also show a practical generalization to a complex-valued Adam variant, which we use to train BigGAN to better inception scores on CIFAR-10.
更多查看译文
关键词
momentum,learning,games,complex
AI 理解论文
溯源树
样例
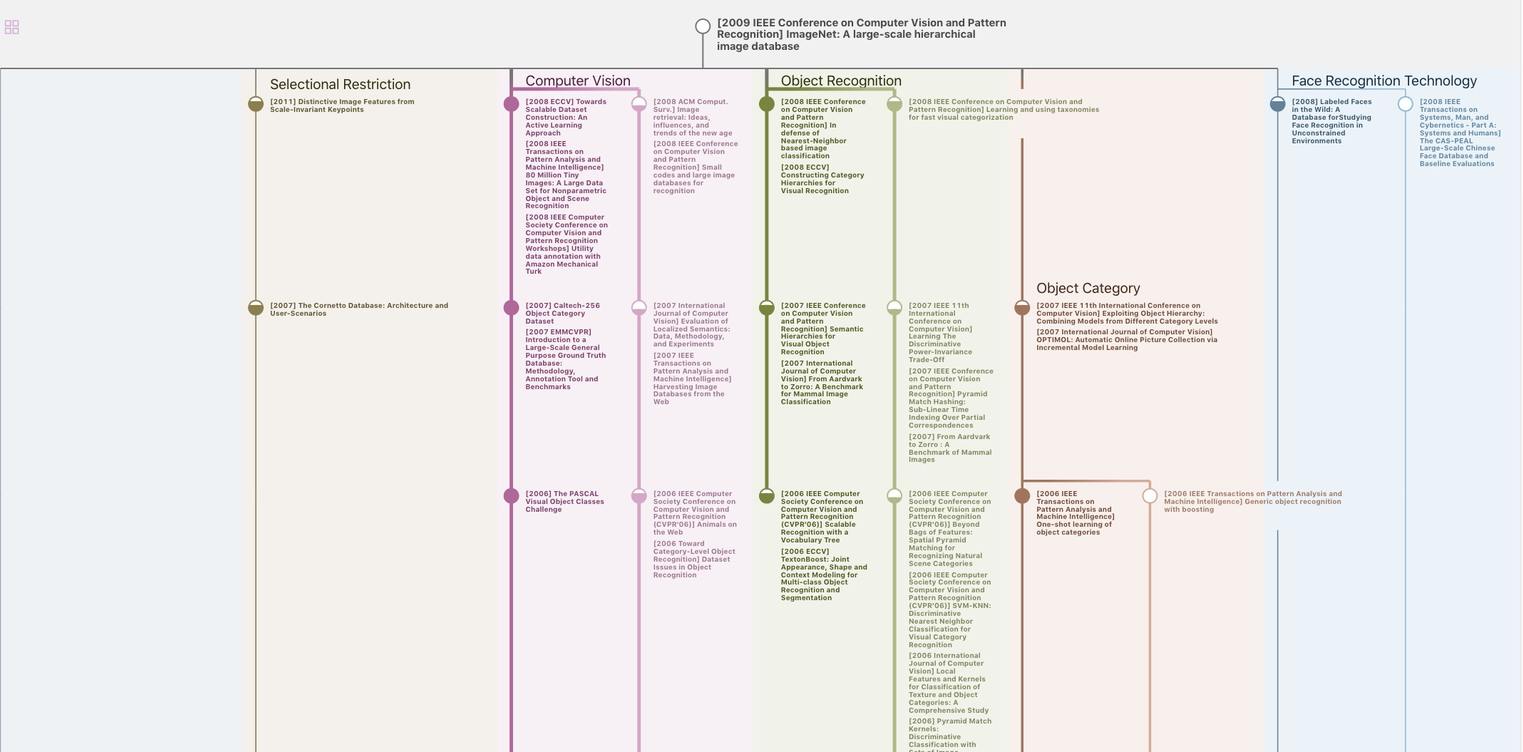
生成溯源树,研究论文发展脉络
Chat Paper
正在生成论文摘要