SMOTE-Based Framework for IoT Botnet Attack Detection.
ACeS(2020)
摘要
Internet of Things (IoT) networks are in danger of being attacked due to their heterogeneity and low power capability. There is a necessity for a more efficient method to protect IoT networks from potential attacks. Machine learning plays a vital role to explore and investigate the malicious behavior of the attacks. However, abnormal distribution of the training datasets among classes causes instability of the classification performance. This paper proposes a framework for IoT botnet attack detection with proper handling of imbalanced classes. It employed a combination of deep neural networks and Synthetic Minority Over-Sampling Technique (SMOTE). The proposed framework consists of training, testing, and evaluation phases. In the proposed framework, standard UCI benchmark datasets were used. The experiment results have demonstrated the effectiveness of the proposed framework in differentiating between normal and malicious traffic attacks.
更多查看译文
关键词
Imbalanced classes, Dataset sampling, Internet of Things (IoT), Botnet attacks
AI 理解论文
溯源树
样例
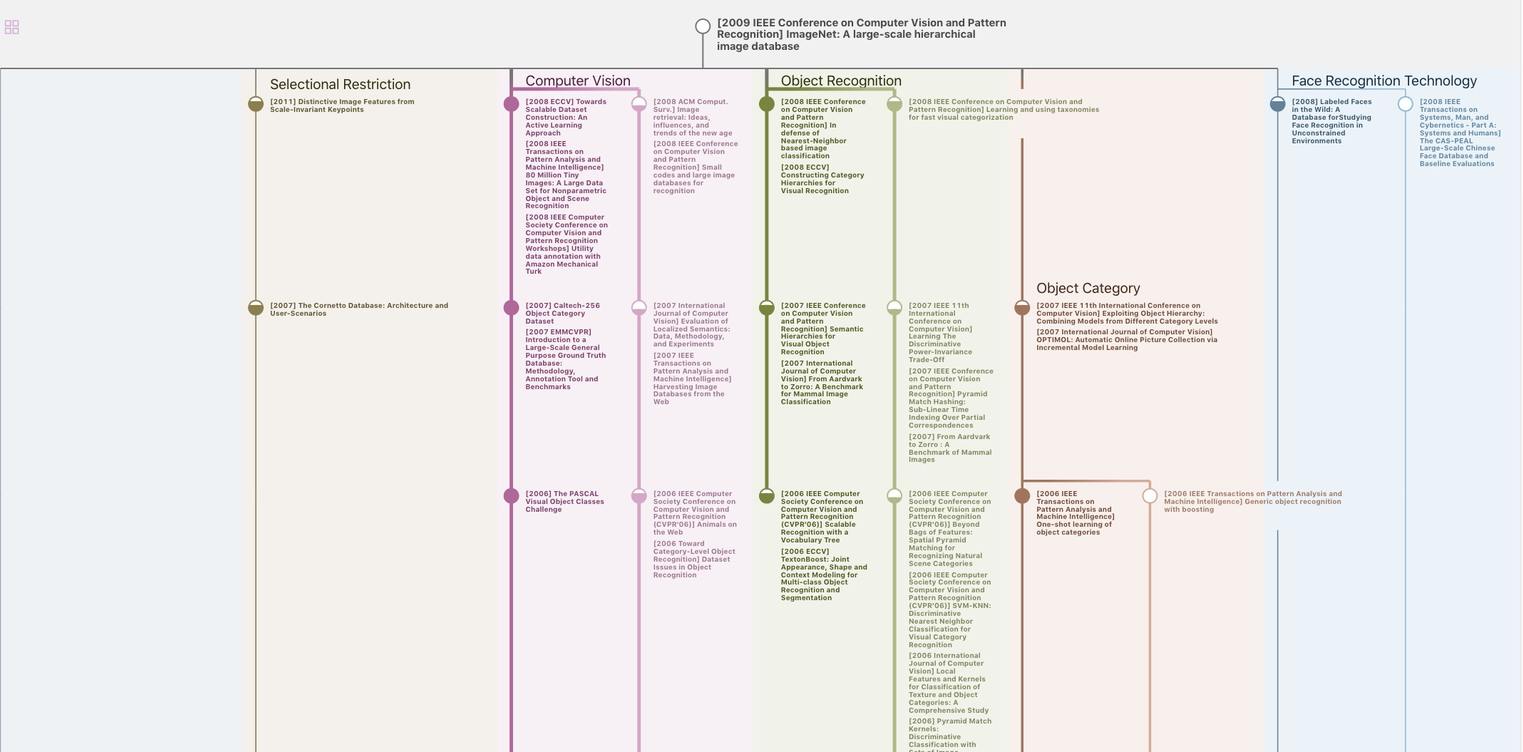
生成溯源树,研究论文发展脉络
Chat Paper
正在生成论文摘要