Neural Posterior Regularization for Likelihood-Free Inference
arxiv(2022)
摘要
A simulation is useful when the phenomenon of interest is either expensive to regenerate or irreproducible with the same context. Recently, Bayesian inference on the distribution of the simulation input parameter has been implemented sequentially to minimize the required simulation budget for the task of simulation validation to the real-world. However, the Bayesian inference is still challenging when the ground-truth posterior is multi-modal with a high-dimensional simulation output. This paper introduces a regularization technique, namely Neural Posterior Regularization (NPR), which enforces the model to explore the input parameter space effectively. Afterward, we provide the closed-form solution of the regularized optimization that enables analyzing the effect of the regularization. We empirically validate that NPR attains the statistically significant gain on benchmark performances for diverse simulation tasks.
更多查看译文
关键词
posterior-aided,likelihood-free
AI 理解论文
溯源树
样例
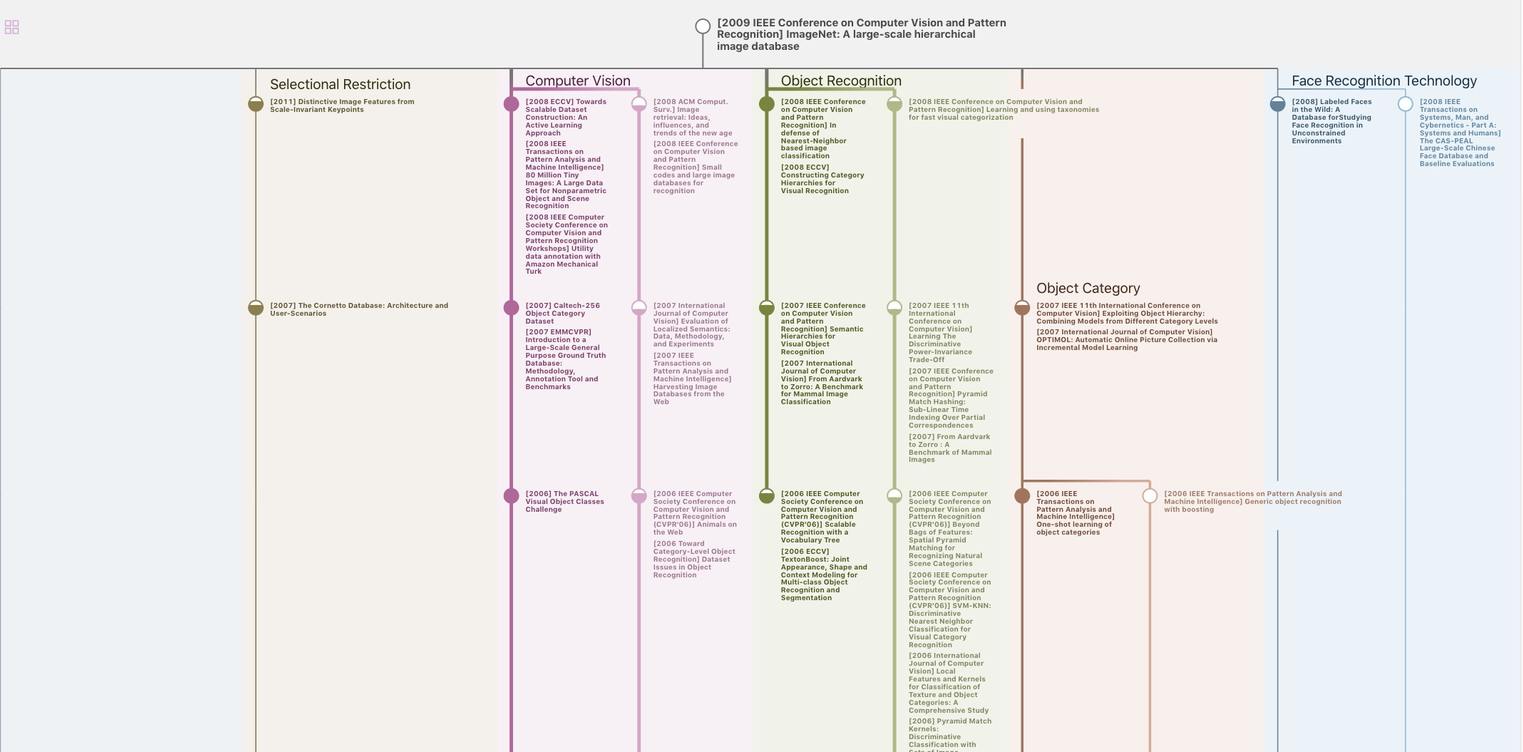
生成溯源树,研究论文发展脉络
Chat Paper
正在生成论文摘要