Horseshoe shrinkage methods for Bayesian fusion estimation
COMPUTATIONAL STATISTICS & DATA ANALYSIS(2022)
摘要
Estimation and structure learning of high-dimensional signals via a normal sequence model are considered, where the underlying parameter vector is piecewise constant, or has a block structure. A Bayesian fusion estimation method is developed by using the Horseshoe prior to induce a strong shrinkage effect on successive differences in the mean parameters, simultaneously imposing sufficient prior concentration for nonzero values of the same. Fast and efficient computational procedures are presented via Markov Chain Monte Carlo methods exploring the full posterior distributions of the underlying parameters, and theoretical justifications of the approach are also provided by deriving posterior convergence rates and establishing selection consistency under suitable assumptions. The proposed method is extended to signal de-noising over arbitrary graphs and efficient computational methods are developed along with theoretical guarantees. The superior performance of the Horseshoe based Bayesian fusion estimation method is demonstrated through extensive simulations and two real-life examples on signal de noising in biological and geophysical applications. The estimation performance of the method is also demonstrated on a real-world large network for the graph signal de-noising problem. (C) 2022 Elsevier B.V. All rights reserved.
更多查看译文
关键词
Bayesian shrinkage, Fusion estimation, Horseshoe prior, Piecewise constant functions, Posterior convergence rate
AI 理解论文
溯源树
样例
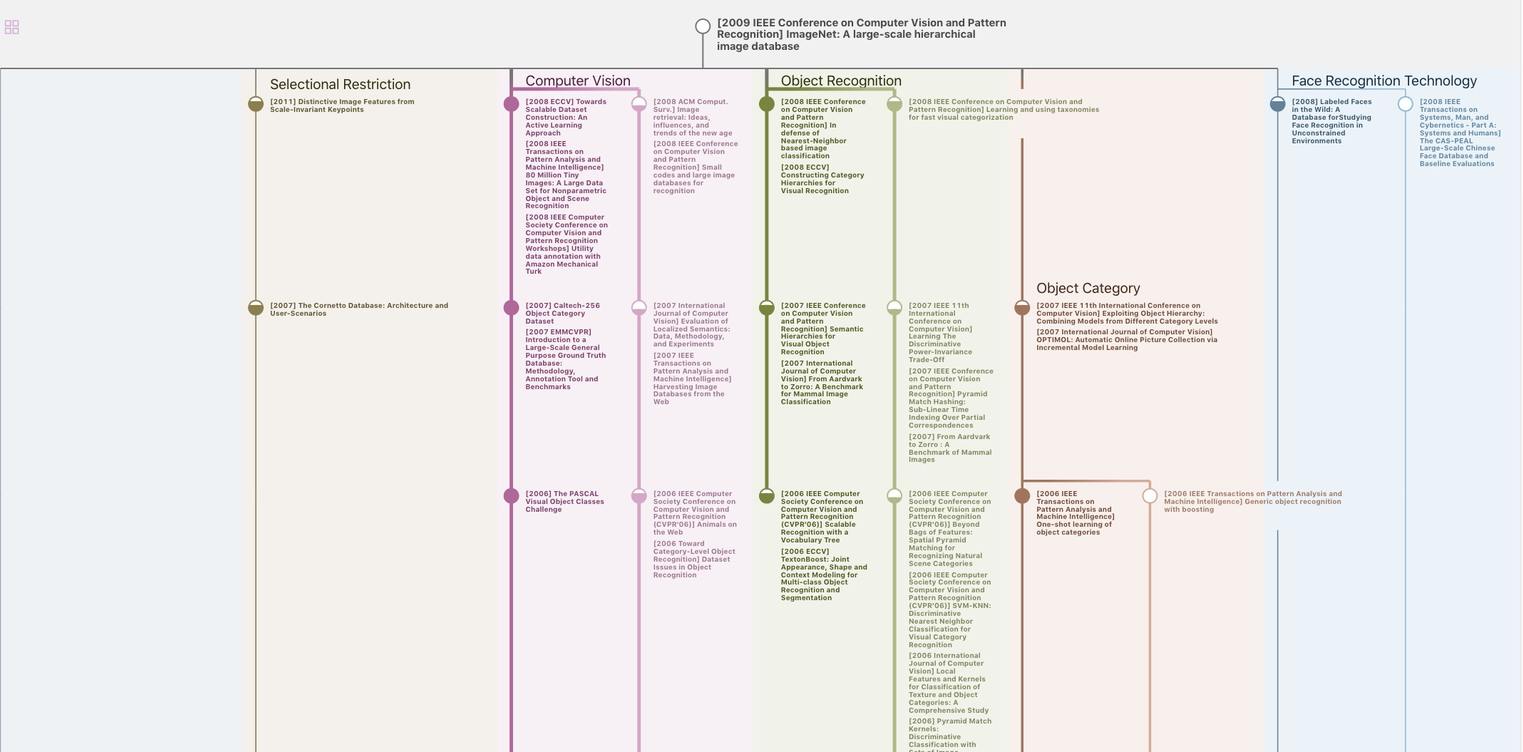
生成溯源树,研究论文发展脉络
Chat Paper
正在生成论文摘要