Think Global and Act Local: Bayesian Optimisation over High-Dimensional Categorical and Mixed Search Spaces
INTERNATIONAL CONFERENCE ON MACHINE LEARNING, VOL 139(2021)
摘要
High-dimensional black-box optimisation remains an important yet notoriously challenging problem. Despite the success of Bayesian optimisation methods on continuous domains, domains that are categorical, or that mix continuous and categorical variables, remain challenging. We propose a novel solution - we combine local optimisation with a tailored kernel design, effectively handling high-dimensional categorical and mixed search spaces, whilst retaining sample efficiency. We further derive convergence guarantee for the proposed approach. Finally, we demonstrate empirically that our method outperforms the current baselines on a variety of synthetic and real-world tasks in terms of performance, computational costs, or both.
更多查看译文
关键词
bayesian optimisation,mixed search spaces,act local,high-dimensional
AI 理解论文
溯源树
样例
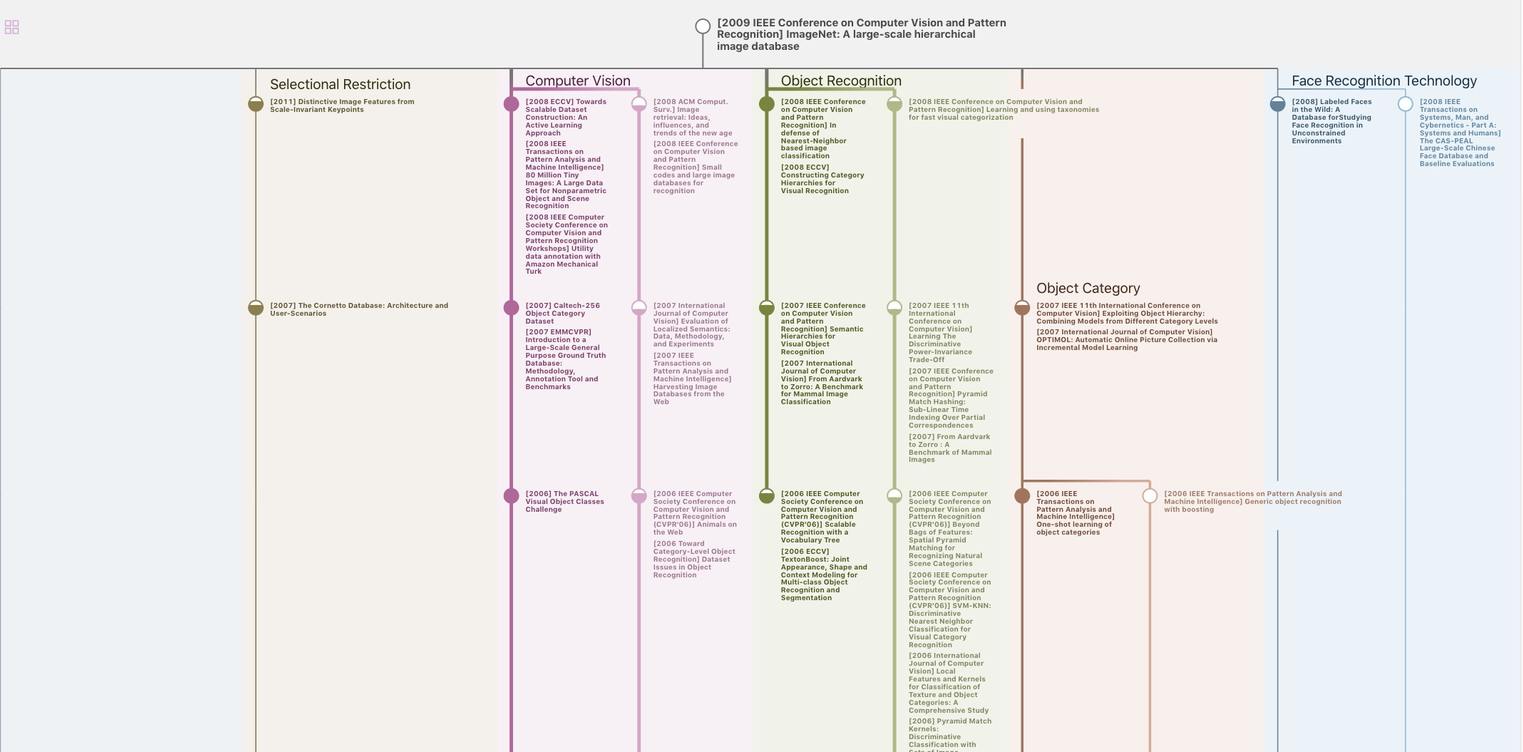
生成溯源树,研究论文发展脉络
Chat Paper
正在生成论文摘要