A New Look and Convergence Rate of Federated Multi-Task Learning with Laplacian Regularization
arxiv(2022)
摘要
Non-Independent and Identically Distributed (non- IID) data distribution among clients is considered as the key factor that degrades the performance of federated learning (FL). Several approaches to handle non-IID data such as personalized FL and federated multi-task learning (FMTL) are of great interest to research communities. In this work, first, we formulate the FMTL problem using Laplacian regularization to explicitly leverage the relationships among the models of clients for multi-task learning. Then, we introduce a new view of the FMTL problem, which in the first time shows that the formulated FMTL problem can be used for conventional FL and personalized FL. We also propose two algorithms FedU and dFedU to solve the formulated FMTL problem in communication-centralized and decentralized schemes, respectively. Theoretically, we prove that the convergence rates of both algorithms achieve linear speedup for strongly convex and sublinear speedup of order 1/2 for nonconvex objectives. Experimentally, we show that our algorithms outperform the algorithm FedAvg, FedProx, SCAFFOLD, and AFL in FL settings, MOCHA in FMTL settings, as well as pFedMe and Per-FedAvg in personalized FL settings.
更多查看译文
关键词
Federated learning (FL),federated multi-task learning (FMTL),Laplacian regularization,personalized learning
AI 理解论文
溯源树
样例
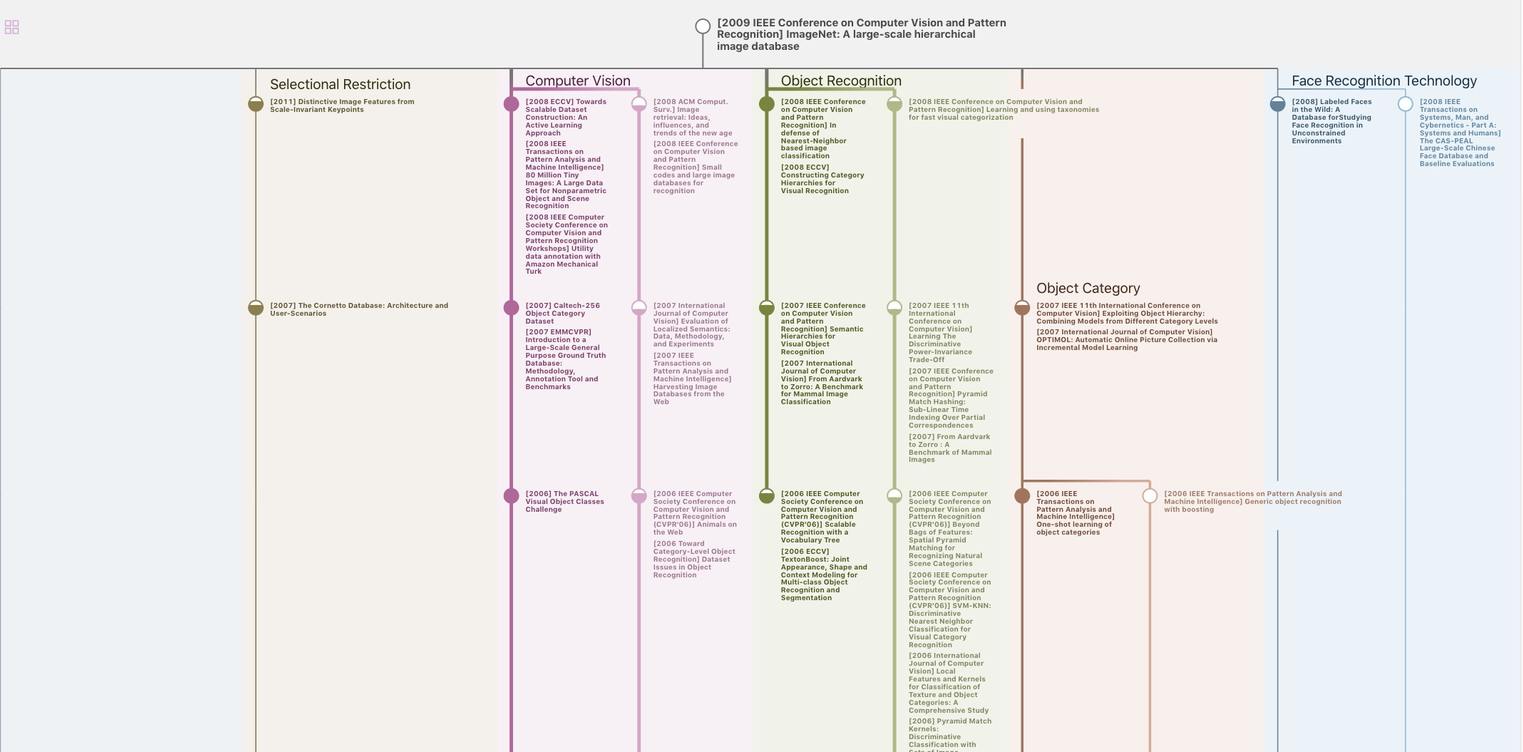
生成溯源树,研究论文发展脉络
Chat Paper
正在生成论文摘要