Domain Adversarial Reinforcement Learning
arxiv(2021)
摘要
We consider the problem of generalization in reinforcement learning where visual aspects of the observations might differ, e.g. when there are different backgrounds or change in contrast, brightness, etc. We assume that our agent has access to only a few of the MDPs from the MDP distribution during training. The performance of the agent is then reported on new unknown test domains drawn from the distribution (e.g. unseen backgrounds). For this "zero-shot RL" task, we enforce invariance of the learned representations to visual domains via a domain adversarial optimization process. We empirically show that this approach allows achieving a significant generalization improvement to new unseen domains.
更多查看译文
关键词
reinforcement,learning
AI 理解论文
溯源树
样例
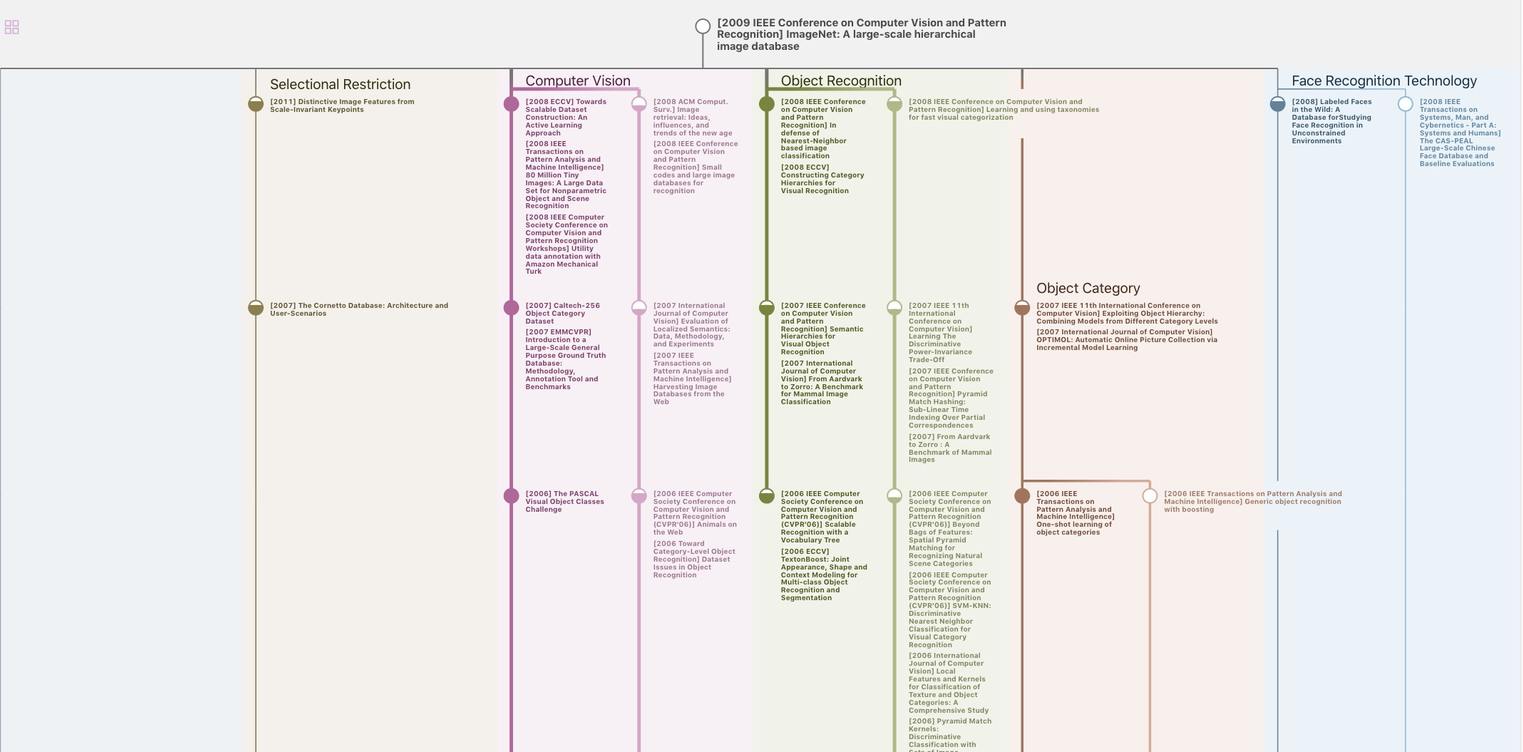
生成溯源树,研究论文发展脉络
Chat Paper
正在生成论文摘要