Demystifying Inductive Biases for (Beta-)VAE Based Architectures
INTERNATIONAL CONFERENCE ON MACHINE LEARNING, VOL 139(2021)
摘要
The performance of beta-Variational-Autoencoders (beta-VAEs) and their variants on learning semantically meaningful, disentangled representations is unparalleled. On the other hand, there are theoretical arguments suggesting the impossibility of unsupervised disentanglement. In this work, we shed light on the inductive bias responsible for the success of VAE-based architectures. We show that in classical datasets the structure of variance induced by the generating factors is conveniently aligned with the latent directions fostered by the VAE objective. This builds the pivotal bias on which the disentangling abilities of VAEs rely. By small, elaborate perturbations of existing datasets, we hide the convenient correlation structure that is easily exploited by a variety of architectures. To demonstrate this, we construct modified versions of standard datasets in which (i) the generative factors are perfectly preserved; (ii) each image undergoes a mild transformation causing a small change of variance; (iii) the leading VAE-based disentanglement architectures fail to produce disentangled representations while the performance of a non-variational method remains unchanged.
更多查看译文
关键词
inductive biases,architectures
AI 理解论文
溯源树
样例
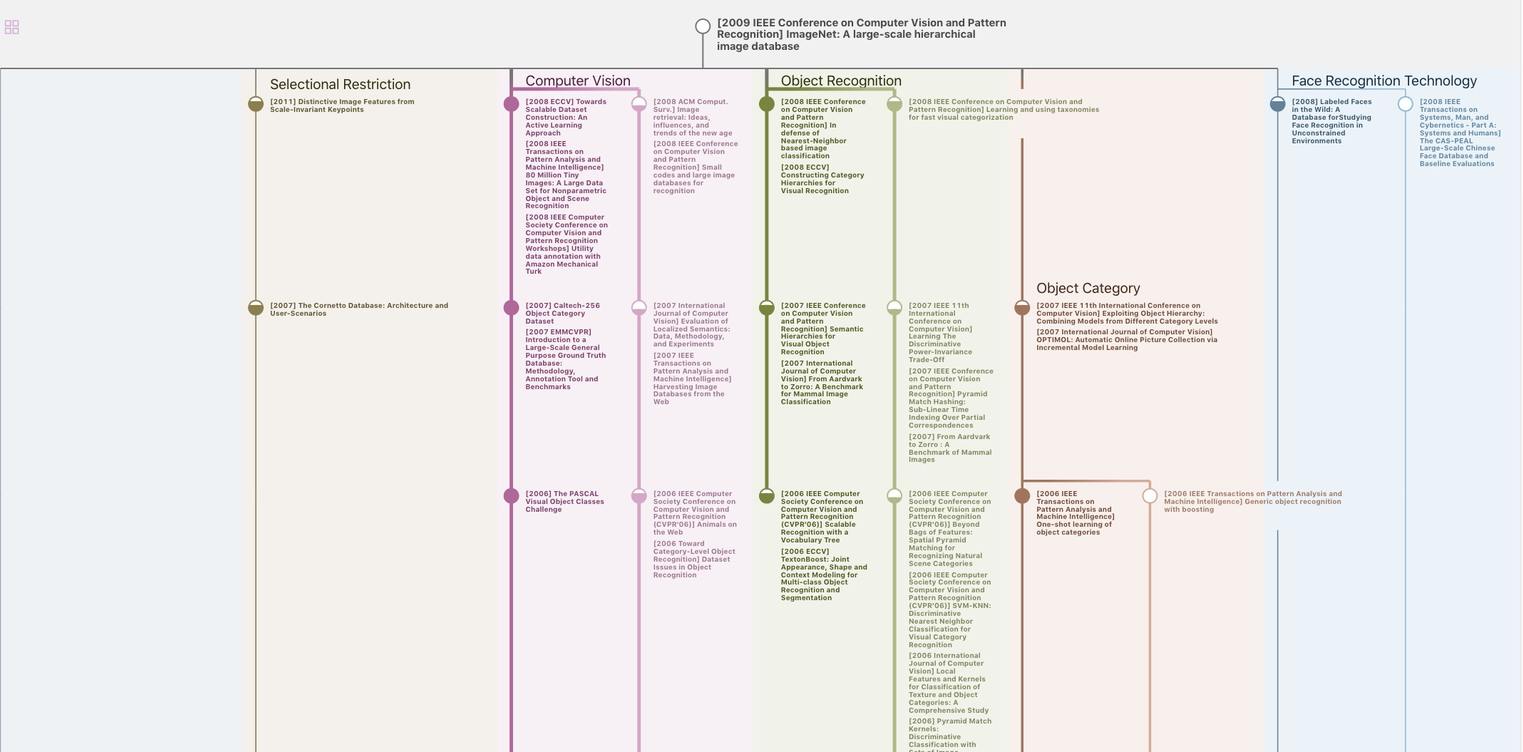
生成溯源树,研究论文发展脉络
Chat Paper
正在生成论文摘要