Neural Inverse Text Normalization
2021 IEEE INTERNATIONAL CONFERENCE ON ACOUSTICS, SPEECH AND SIGNAL PROCESSING (ICASSP 2021)(2021)
摘要
While there have been several contributions exploring state of the art techniques for text normalization, the problem of inverse text normalization (ITN) remains relatively unexplored. The best known approaches leverage finite state transducer (FST) based models which rely on manually curated rules and are hence not scalable. We propose an efficient and robust neural solution for ITN leveraging transformer based seq2seq models and FST-based text normalization techniques for data preparation. We show that this can be easily extended to other languages without the need for a linguistic expert to manually curate them. We then present a hybrid framework for integrating Neural ITN with an FST to overcome common recoverable errors in production environments. Our empirical evaluations show that the proposed solution minimizes incorrect perturbations (insertions, deletions and substitutions) to ASR output and maintains high quality even on out of domain data. A transformer based model infused with pretraining consistently achieves a lower WER across several datasets and is able to outperform baselines on English, Spanish, German and Italian datasets.
更多查看译文
关键词
speech recognition, inverse text normalization, multilingual
AI 理解论文
溯源树
样例
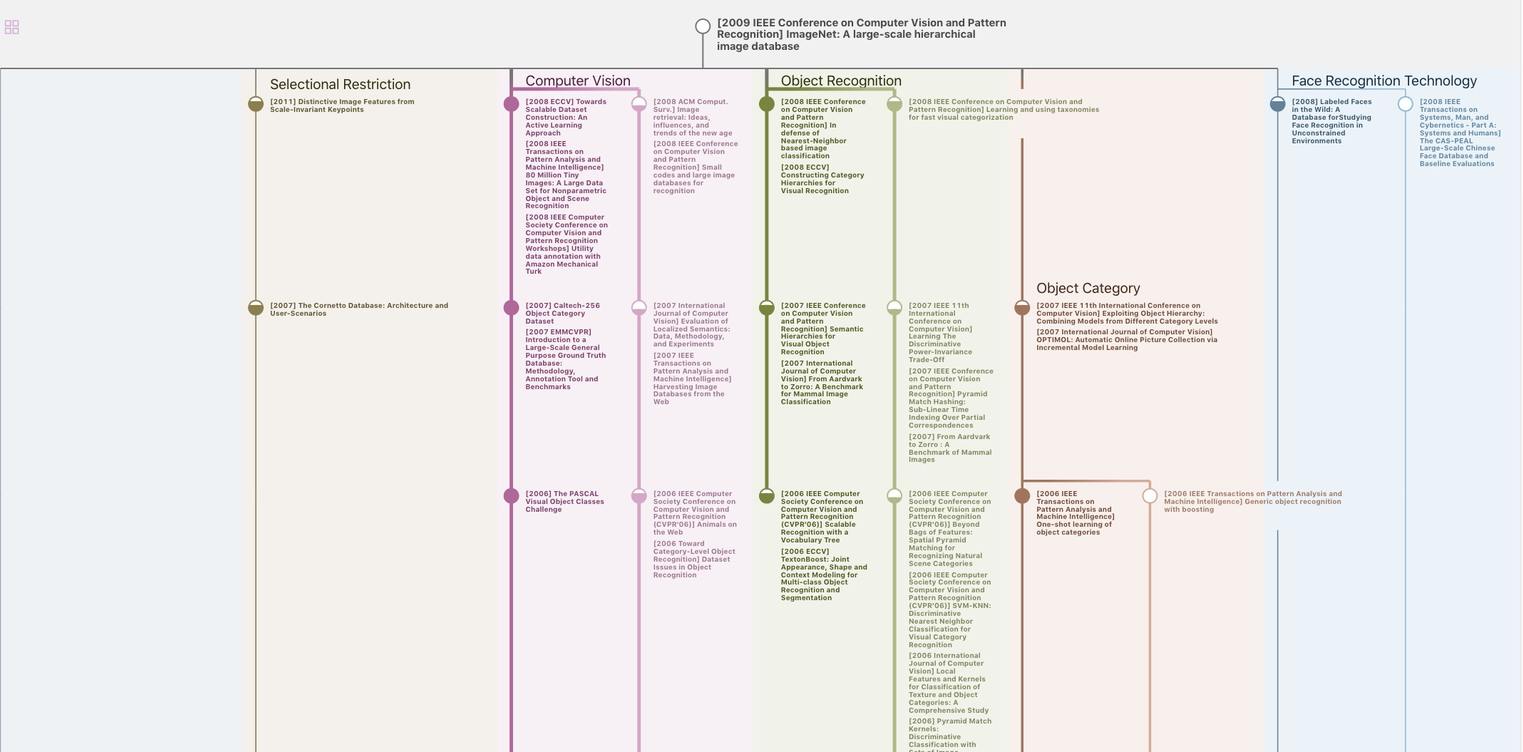
生成溯源树,研究论文发展脉络
Chat Paper
正在生成论文摘要