CONTRASTIVE UNSUPERVISED LEARNING FOR SPEECH EMOTION RECOGNITION
2021 IEEE INTERNATIONAL CONFERENCE ON ACOUSTICS, SPEECH AND SIGNAL PROCESSING (ICASSP 2021)(2021)
摘要
Speech emotion recognition (SER) is a key technology to enable more natural human-machine communication. However, SER has long suffered from a lack of public large-scale labeled datasets. To circumvent this problem, we investigate how unsupervised representation learning on unlabeled datasets can benefit SER. We show that the contrastive predictive coding (CPC) method can learn salient representations from unlabeled datasets, which improves emotion recognition performance. In our experiments, this method achieved state-of-the-art concordance correlation coefficient (CCC) performance for all emotion primitives (activation, valence, and dominance) on IEMOCAP. Additionally, on the MSP-Podcast dataset, our method obtained considerable performance improvements compared to baselines.
更多查看译文
关键词
Speech emotion recognition, Contrastive predictive coding, Unsupervised pre-training
AI 理解论文
溯源树
样例
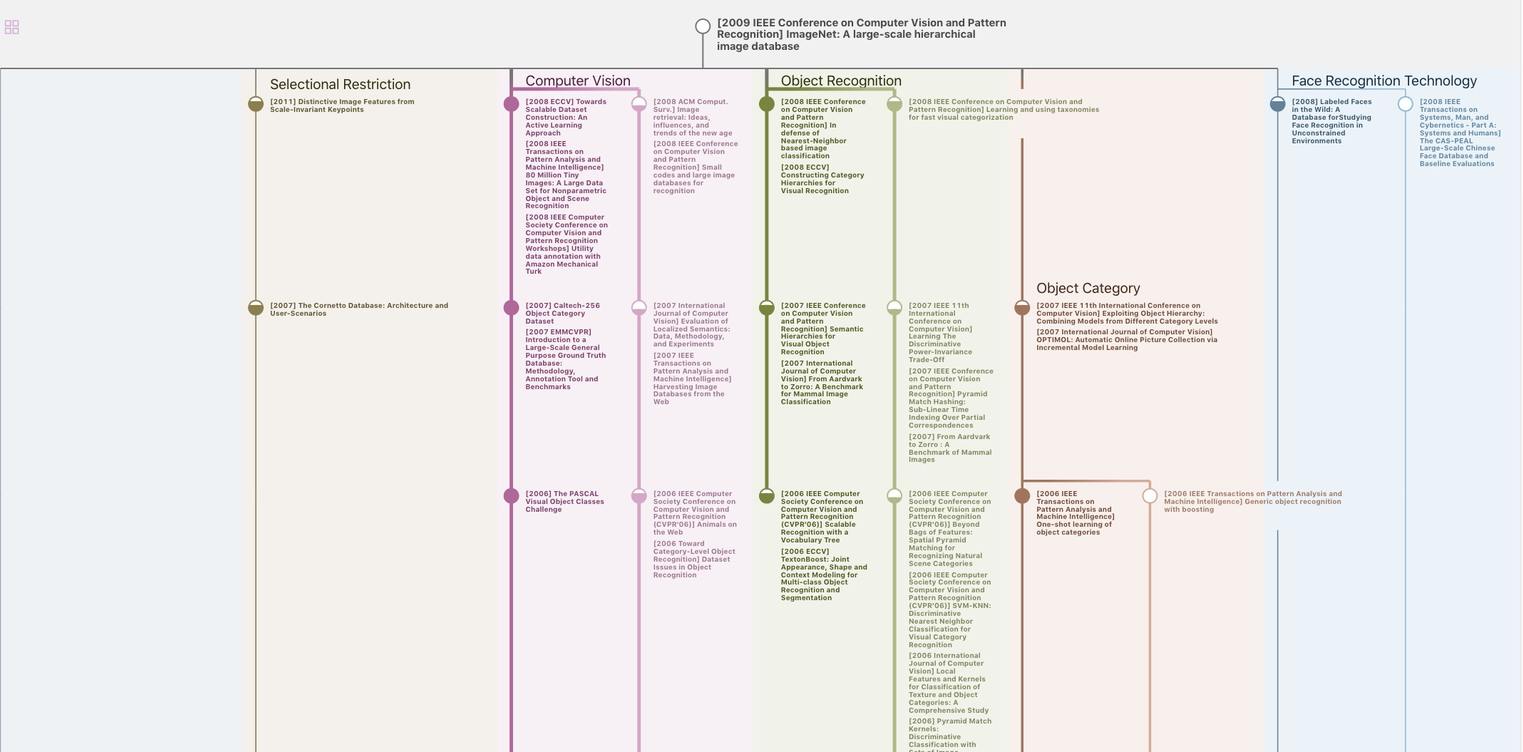
生成溯源树,研究论文发展脉络
Chat Paper
正在生成论文摘要