Higher Order Generalization Error for First Order Discretization of Langevin Diffusion
arxiv(2021)
摘要
We propose a novel approach to analyze generalization error for discretizations of Langevin diffusion, such as the stochastic gradient Langevin dynamics (SGLD). For an $\epsilon$ tolerance of expected generalization error, it is known that a first order discretization can reach this target if we run $\Omega(\epsilon^{-1} \log (\epsilon^{-1}) )$ iterations with $\Omega(\epsilon^{-1})$ samples. In this article, we show that with additional smoothness assumptions, even first order methods can achieve arbitrarily runtime complexity. More precisely, for each $N>0$, we provide a sufficient smoothness condition on the loss function such that a first order discretization can reach $\epsilon$ expected generalization error given $\Omega( \epsilon^{-1/N} \log (\epsilon^{-1}) )$ iterations with $\Omega(\epsilon^{-1})$ samples.
更多查看译文
关键词
langevin diffusion,first order discretization,higher order generalization error,first order
AI 理解论文
溯源树
样例
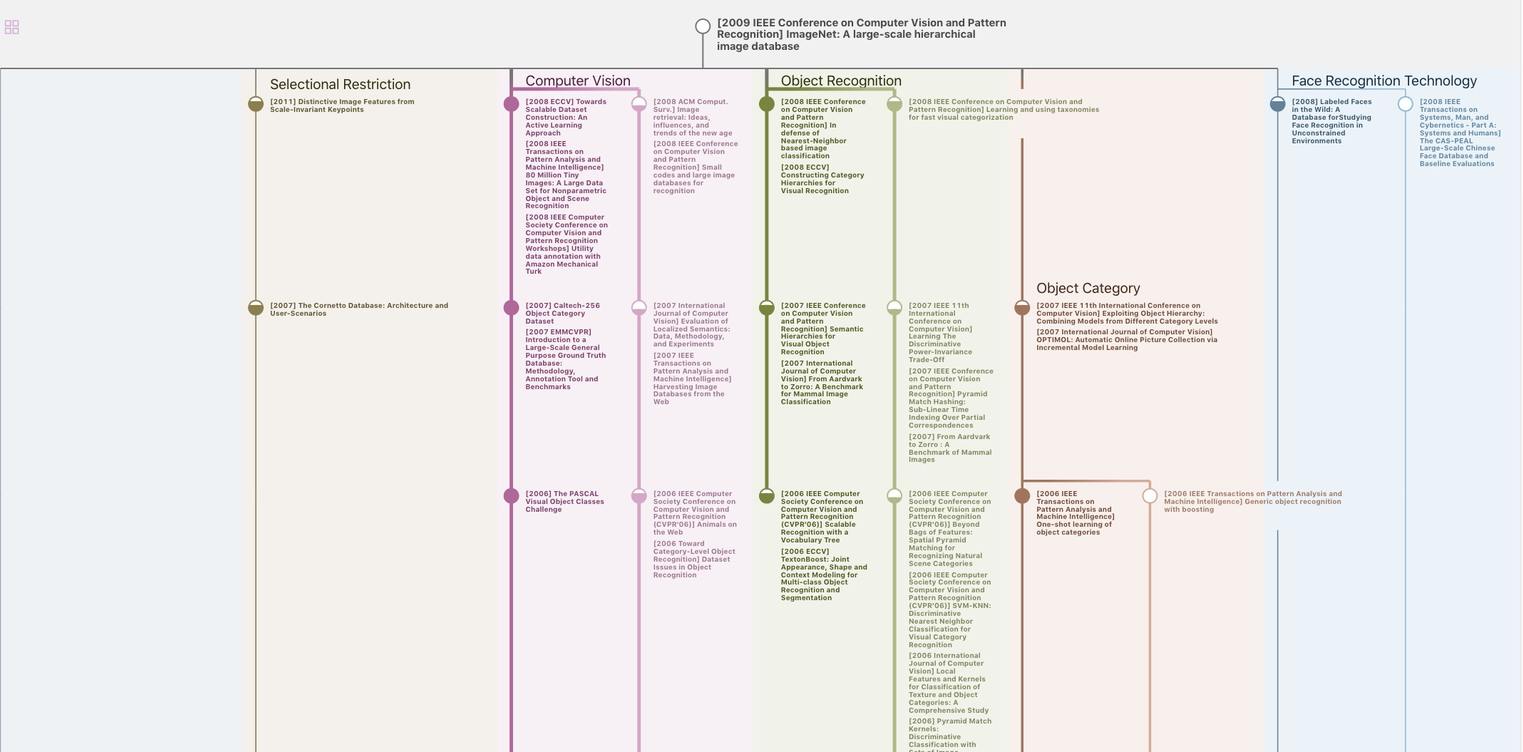
生成溯源树,研究论文发展脉络
Chat Paper
正在生成论文摘要