The local-balanced model for improved machine learning outcomes on mass spectrometry data sets and other instrumental data
ANALYTICAL AND BIOANALYTICAL CHEMISTRY(2021)
摘要
One unifying challenge when classifying biological samples with mass spectrometry data is overcoming the obstacle of sample-to-sample variability so that differences between groups, such as between a healthy set and a disease set, can be identified. Similarly, when the same sample is re-analyzed under identical conditions, instrument signals can fluctuate by more than 10%. This signal inconsistency imposes difficulties in identifying subtle differences across a set of samples, and it weakens the mass spectrometrist’s ability to effectively leverage data in domains as diverse as proteomics, metabolomics, glycomics, and imaging. We selected challenging data sets in the fields of glycomics, mass spectrometry imaging, and bacterial typing to study the problem of within-group signal variability and adapted a 30-year-old statistical approach to address the problem. The solution, “local-balanced model,” relies on using balanced subsets of training data to classify test samples. This analysis strategy was assessed on ESI-MS data of IgG-based glycopeptides and MALDI-MS imaging data of endogenous lipids, and MALDI-MS data of bacterial proteins. Two preliminary examples on non-mass spectrometry data sets are also included to show the potential generality of the method outside the field of MS analysis. We demonstrate that this approach is superior to simple normalization methods, generalizable to multiple mass spectrometry domains, and potentially appropriate in fields as diverse as physics and satellite imaging. In some cases, improvements in classification can be dramatic, with accuracy escalating from 60% with normalization alone to over 90% with the additional development described herein. Graphical abstract
更多查看译文
关键词
Software, Genomics, proteomics, Mass spectrometry, Machine learning, Imaging, Glycoprotein
AI 理解论文
溯源树
样例
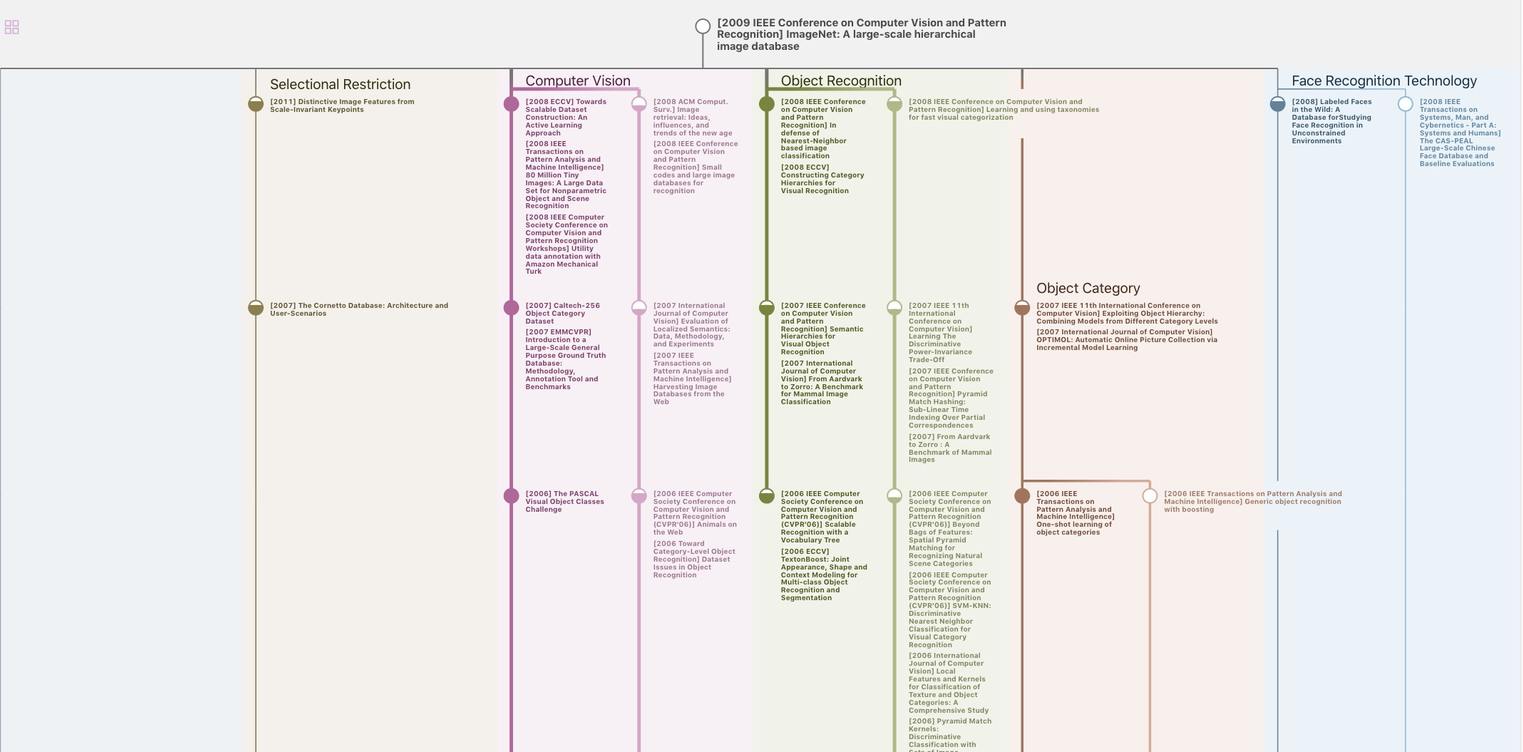
生成溯源树,研究论文发展脉络
Chat Paper
正在生成论文摘要