A Novel Approach To Fully Representing The Diversity In Conditional Dependencies For Learning Bayesian Network Classifier
INTELLIGENT DATA ANALYSIS(2021)
摘要
Bayesian network classifiers (BNCs) have proved their effectiveness and efficiency in the supervised learning framework. Numerous variations of conditional independence assumption have been proposed to address the issue of NP-hard structure learning of BNC. However, researchers focus on identifying conditional dependence rather than conditional independence, and information-theoretic criteria cannot identify the diversity in conditional (in)dependencies for different instances. In this paper, the maximum correlation criterion and minimum dependence criterion are introduced to sort attributes and identify conditional independencies, respectively. The heuristic search strategy is applied to find possible global solution for achieving the trade-off between significant dependency relationships and independence assumption. Our extensive experimental evaluation on widely used benchmark data sets reveals that the proposed algorithm achieves competitive classification performance compared to state-of-the-art single model learners (e.g., TAN, KDB, KNN and SVM) and ensemble learners (e.g., ATAN and AODE).
更多查看译文
关键词
Bayesian network classifier, maximum correlation criterion, minimum dependence criterion, conditional independence, conditional mutual information
AI 理解论文
溯源树
样例
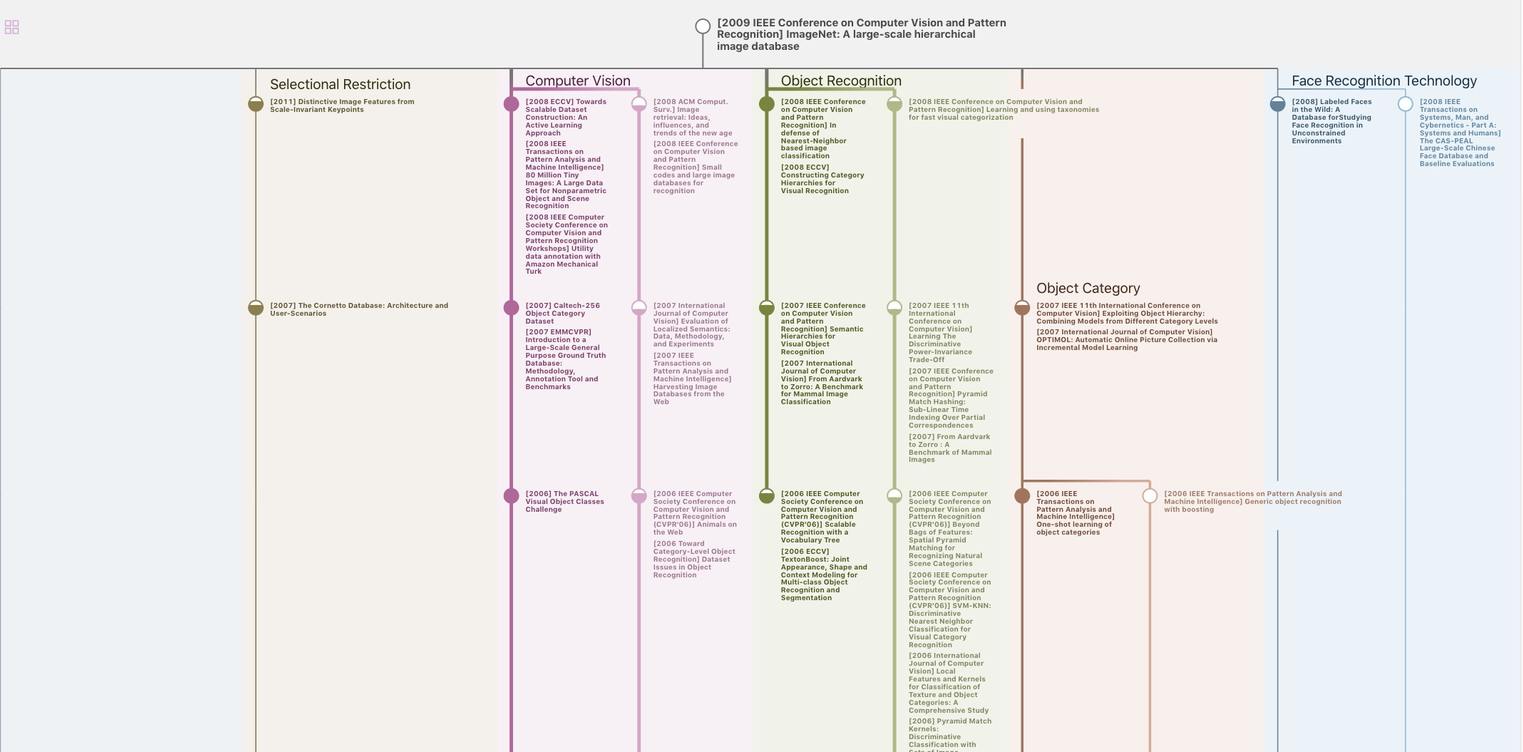
生成溯源树,研究论文发展脉络
Chat Paper
正在生成论文摘要