Heterogeneous Security Events Prioritization Using Auto-encoders.
CRiSIS(2020)
摘要
In a large monitored information system, analysts are confronted with a huge number of heterogeneous events or alerts produced by audit mechanisms or Intrusion Detection Systems. Even though they can use SIEM software to collect and analyse these events (In this paper we call events all events or alerts produced by the monitoring processes), detecting previously unknown threats is tedious. Event prioritization tools can help the analyst focus on potentially anomalous events. To compute a measure of priority among events, we propose in this paper to define the notion of an anomaly score for each attribute of the analyzed events and a method for regrouping events in clusters to reduce the number of alerts the analysts have to qualify. The anomaly score is computed using neural networks (i.e., auto-encoders) trained on a normal dataset of events, and then used to provide the analyst with the information of the difference between normal learned events and the events actually produced by the monitoring system. Additionally, the auto-encoders also provide a way to regroup similar events via clustering.
更多查看译文
关键词
Heterogeneous logs,Anomaly detection,Anomaly score,Cybersecurity,Intrusion detection,Machine learning
AI 理解论文
溯源树
样例
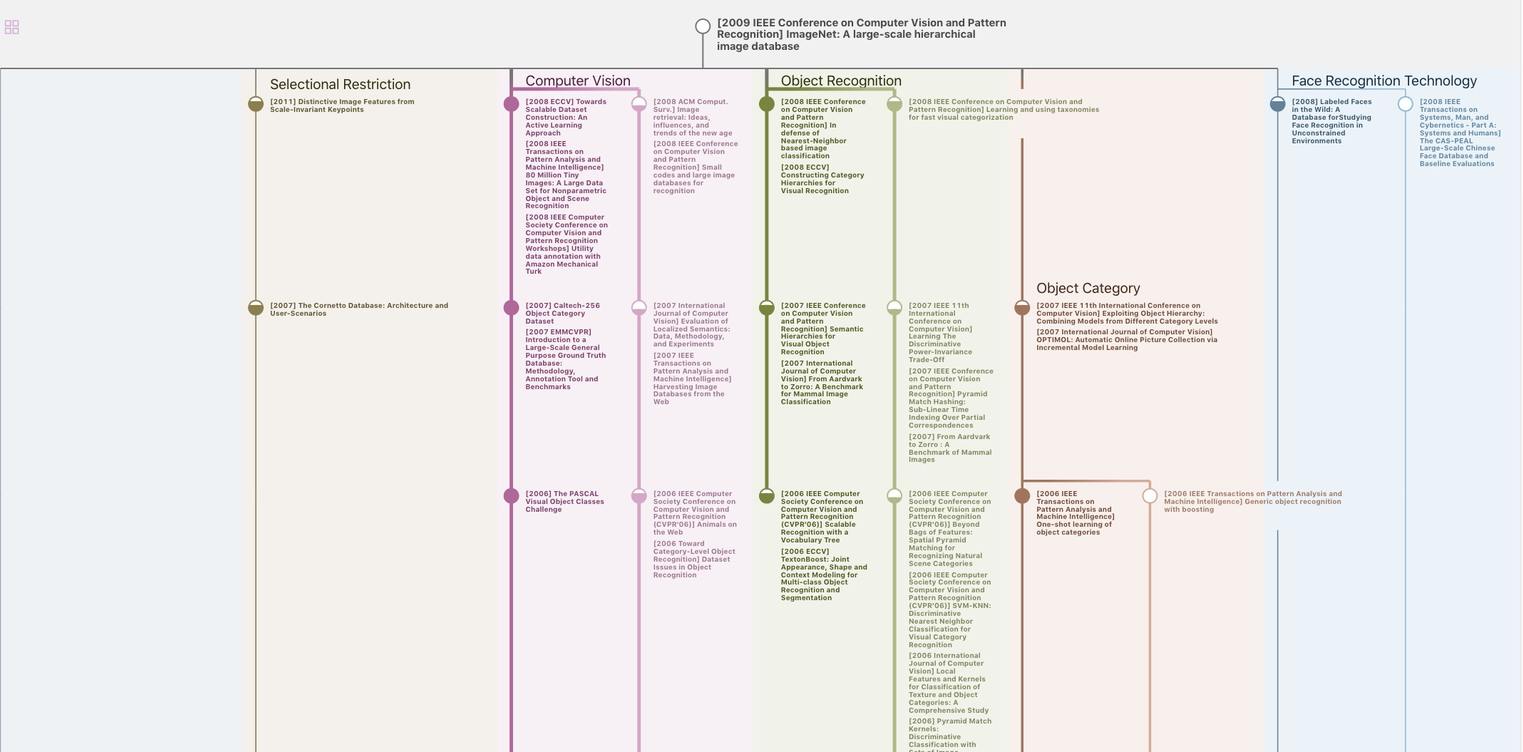
生成溯源树,研究论文发展脉络
Chat Paper
正在生成论文摘要