Fused Acoustic and Text Encoding for Multimodal Bilingual Pretraining and Speech Translation
INTERNATIONAL CONFERENCE ON MACHINE LEARNING, VOL 139(2021)
摘要
Recently, representation learning for text and speech has successfully improved many language related tasks. However, all existing methods suffer from two limitations: (a) they only learn from one input modality, while a unified representation for both speech and text is needed by tasks such as end-to-end speech translation, and as a result, (b) they can not exploit various large-scale text and speech data and their performance is limited by the scarcity of parallel speech translation data. To address these problems, we propose a Fused Acoustic and Text Masked Language Model (FAT-MLM) which jointly learns a unified representation for both acoustic and text input from various types of corpora including parallel data for speech recognition and machine translation, and even pure speech and text data. Within this cross-modal representation learning framework, we further present an end-to-end model for Fused Acoustic and Text Speech Translation (FAT-ST). Experiments on three translation directions show that by fine-tuning from FAT-MLM, our proposed speech translation models substantially improve translation quality by up to +5.9 BLEU.
更多查看译文
关键词
multimodal bilingual pretraining,speech translation,text encoding,acoustic
AI 理解论文
溯源树
样例
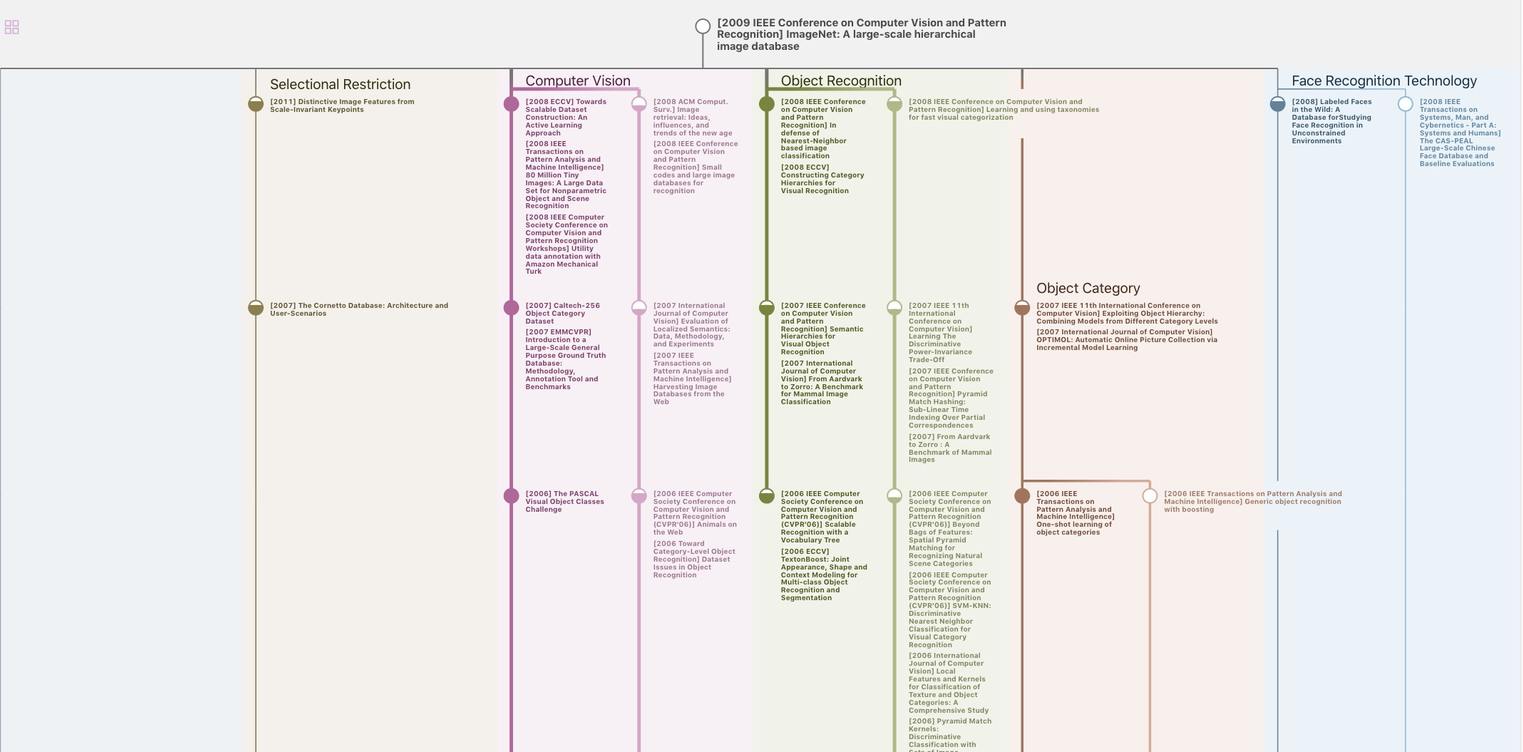
生成溯源树,研究论文发展脉络
Chat Paper
正在生成论文摘要