Towards Certifying l(infinity) Robustness using Neural Networks with l(infinity)-dist Neurons
ICML 2021(2021)
摘要
It is well-known that standard neural networks, even with a high classification accuracy, are vulnerable to small l(infinity)-norm bounded adversarial perturbations. Although many attempts have been made, most previous works either can only provide empirical verification of the defense to a particular attack method, or can only develop a certified guarantee of the model robustness in limited scenarios. In this paper, we seek for a new approach to develop a theoretically principled neural network that inherently resists l(infinity) perturbations. In particular, we design a novel neuron that uses .e ms -distance as its basic operation (which we call l(infinity)-dist neuron), and show that any neural network constructed with l(infinity)-dist neurons (called l(infinity)-dist net) is naturally a 1-Lipschitz function with respect to l(infinity)-norm. This directly provides a rigorous guarantee of the certified robustness based on the margin of prediction outputs. We then prove that such networks have enough expressive power to approximate any 1-Lipschitz function with robust generalization guarantee. We further provide a holistic training strategy that can greatly alleviate optimization difficulties. Experimental results show that using l(infinity)-dist nets as basic building blocks, we consistently achieve state-of-the-art performance on commonly used datasets: 93.09% certified accuracy on MNIST (epsilon = 0.3), 35.42% on CIFAR-10 (epsilon = 8/255) and 16.31% on TinylmageNet (epsilon = 1/255).
更多查看译文
关键词
robustness,neural networks,neurons
AI 理解论文
溯源树
样例
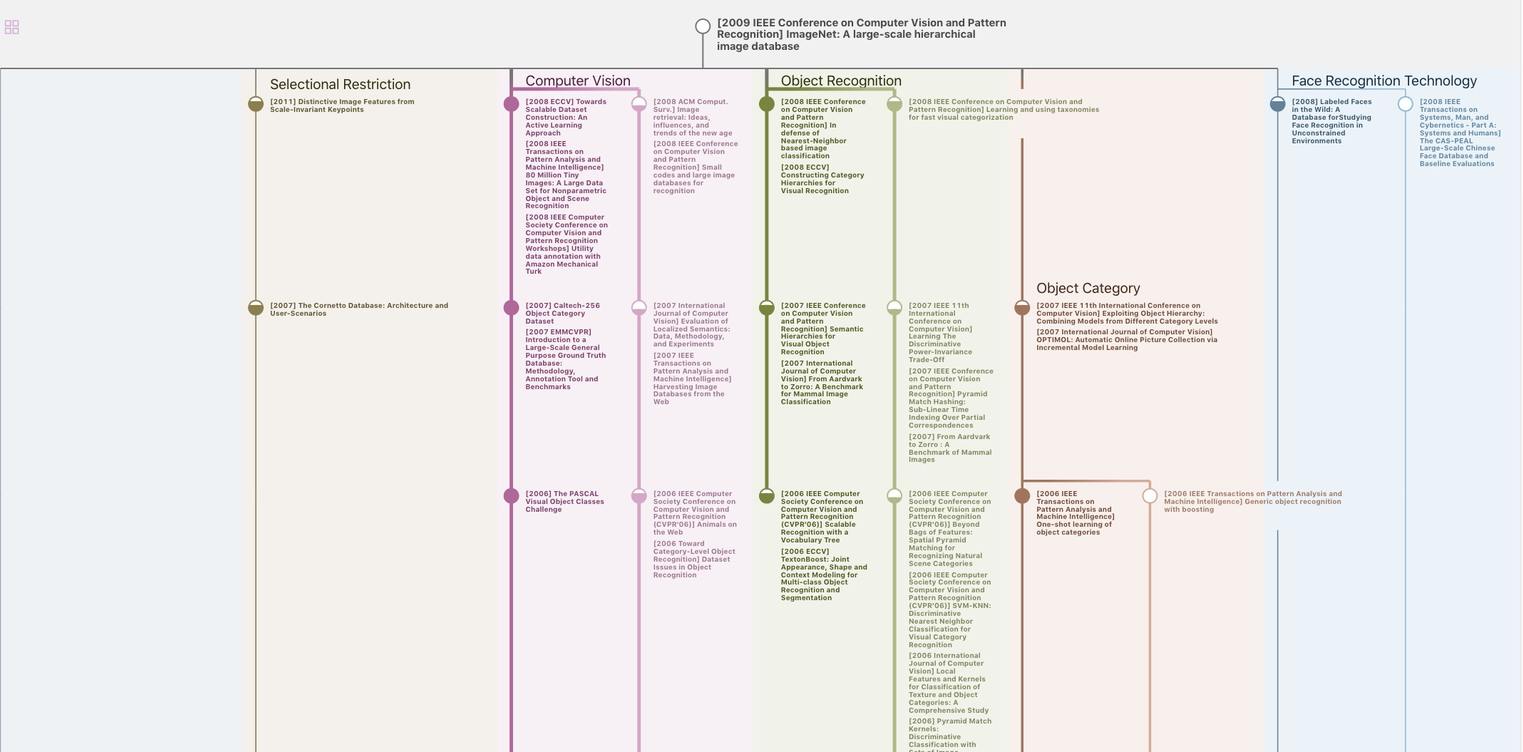
生成溯源树,研究论文发展脉络
Chat Paper
正在生成论文摘要