Hardware-Aware In Situ Learning Based on Stochastic Magnetic Tunnel junctions
PHYSICAL REVIEW APPLIED(2022)
摘要
One of the big challenges of current electronics is the design and implementation of hardware neural networks that perform fast and energy-efficient machine learning. Spintronics is a promising catalyst for this field with the capabilities of nanosecond operation and compatibility with existing microelectronics. Considering large-scale, viable neuromorphic systems however, variability of device properties is a serious concern. In this paper, we show an autonomously operating circuit that performs hardware-aware machine learning utilizing probabilistic neurons built with stochastic magnetic tunnel junctions. We show that in situ learning of weights and biases in a Boltzmann machine can counter device-to-device variations and learn the probability distribution of meaningful operations such as a full adder. This scalable autonomously operating learning circuit using spintronics-based neurons could be especially of interest for standalone artificial-intelligence devices capable of fast and efficient learning at the edge.
更多查看译文
AI 理解论文
溯源树
样例
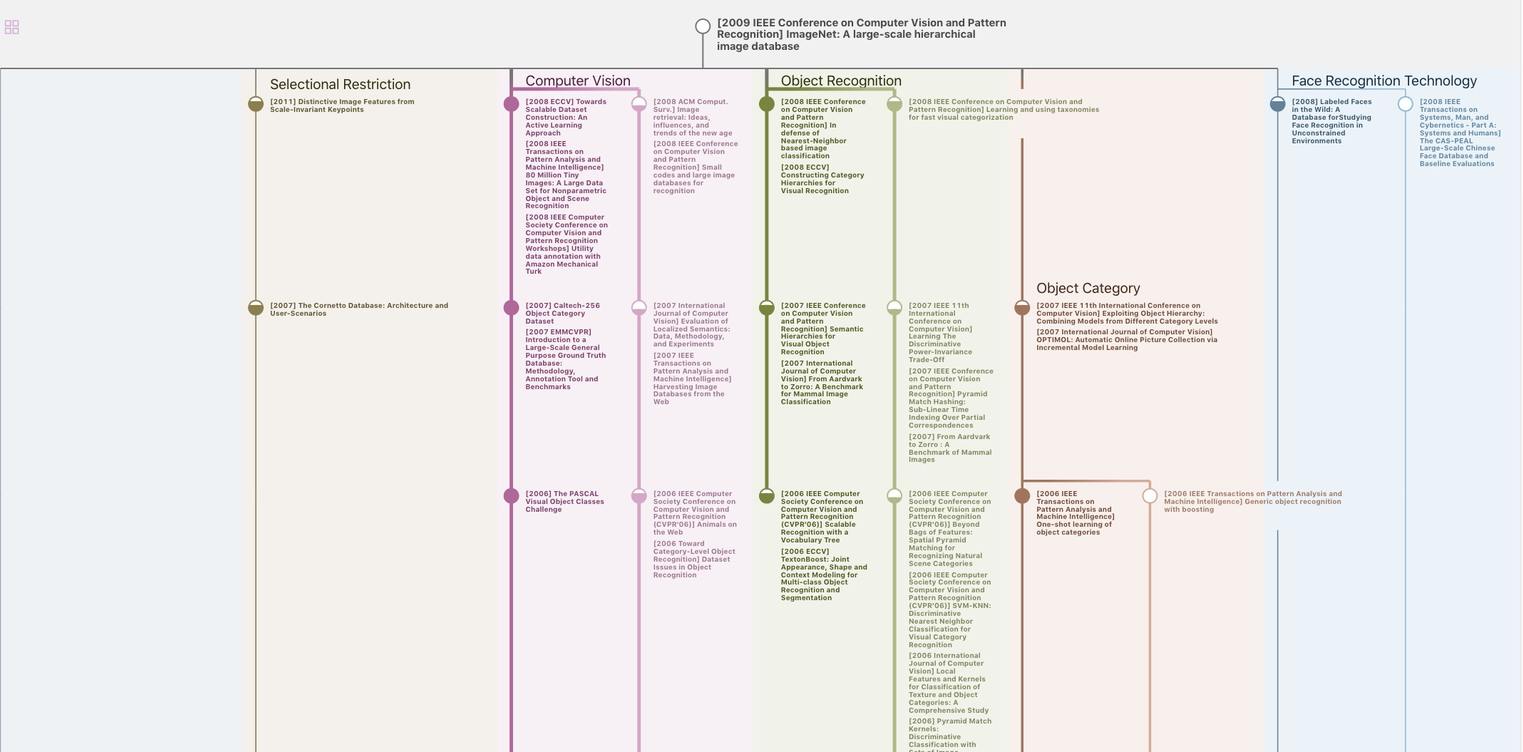
生成溯源树,研究论文发展脉络
Chat Paper
正在生成论文摘要