LEARNING MULTI-MODAL VOLUMETRIC PROSTATE REGISTRATION WITH WEAK INTER-SUBJECT SPATIAL CORRESPONDENCE
2021 IEEE 18TH INTERNATIONAL SYMPOSIUM ON BIOMEDICAL IMAGING (ISBI)(2021)
摘要
Recent studies demonstrated the eligibility of convolutional neural networks (CNNs) for solving the image registration problem. CNNs enable faster transformation estimation and greater generalization capability needed for better support during medical interventions. Conventional fully-supervised training requires a lot of high-quality ground truth data such as voxel-to-voxel transformations, which typically are attained in a too tedious and error-prone manner. In our work, we use weakly-supervised learning, which optimizes the model indirectly only via segmentation masks that are a more accessible ground truth than the deformation fields. Concerning the weak supervision, we investigate two segmentation similarity measures: multiscale Dice similarity coefficient (mDSC) and the similarity between segmentation-derived signed distance maps (SDMs). We show that the combination of mDSC and SDM similarity measures results in a more accurate and natural transformation pattern together with a stronger gradient coverage. Furthermore, we introduce an auxiliary input to the neural network for the prior information about the prostate location in the MR sequence, which mostly is available preoperatively. This approach significantly outperforms the standard two-input models. With weakly labelled MR-TRUS prostate data, we showed registration quality comparable to the state-of-the-art deep learning-based method.
更多查看译文
关键词
multi-modal registration, image-guided prostate biopsy, weakly-supervised learning
AI 理解论文
溯源树
样例
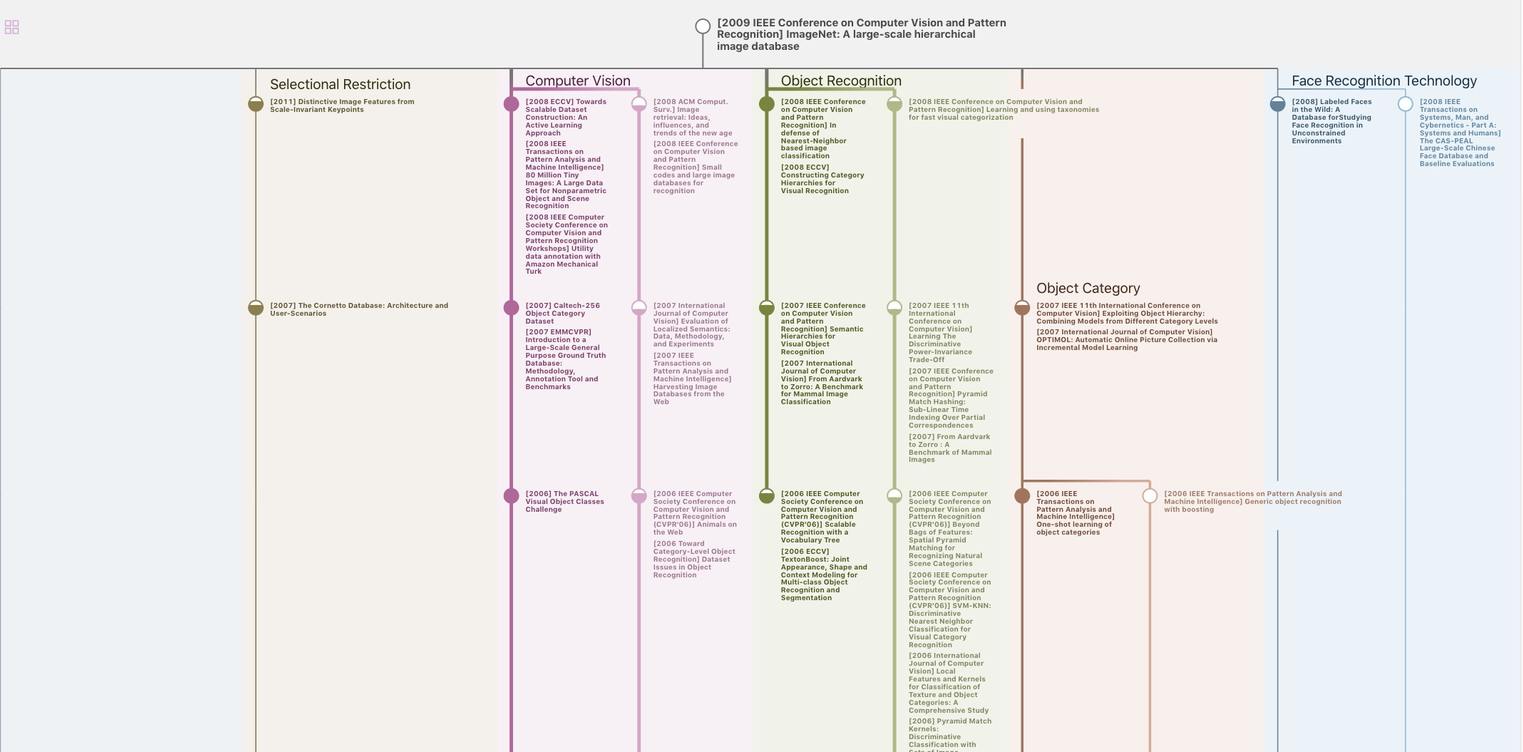
生成溯源树,研究论文发展脉络
Chat Paper
正在生成论文摘要