Automated Tip Conditioning For Scanning Tunneling Spectroscopy
JOURNAL OF PHYSICAL CHEMISTRY A(2021)
摘要
Scanning tunneling spectroscopy (STS), a technique that records the change in the tunneling current as a function of the bias (dI/dV) across the gap between a tip and the sample, is a powerful tool to characterize the electronic structure of single molecules and nanomaterials. While performing STS, the structure and condition of the scanning probe microscopy (SPM) tips are critical for reliably obtaining high quality point spectra. Here, we present an automated program based on machine learning models that can identify the Au(111) Shockley surface state in dI/dV point spectra and perform tip conditioning on clean or sparsely covered gold surfaces with minimal user intervention. We employed a straightforward height-based segmentation algorithm to analyze STM topographic images to identify tip conditioning positions and used 1789 archived dI/dV spectra to train machine learning models that can ascertain the condition of the tip by evaluating the quality of the spectroscopic data. Decision tree based ensemble and boosting models and deep neural networks (DNNs) have been shown to reliably identify tips in suitable conditions for STS. We expect the automated program to reduce operational costs and time, increase reproducibility in surface science studies, and accelerate the discovery and characterization of novel nanomaterials by STM. The strategies presented in this paper can readily be adapted to STM tip conditioning on a wide variety of other common substrates.
更多查看译文
AI 理解论文
溯源树
样例
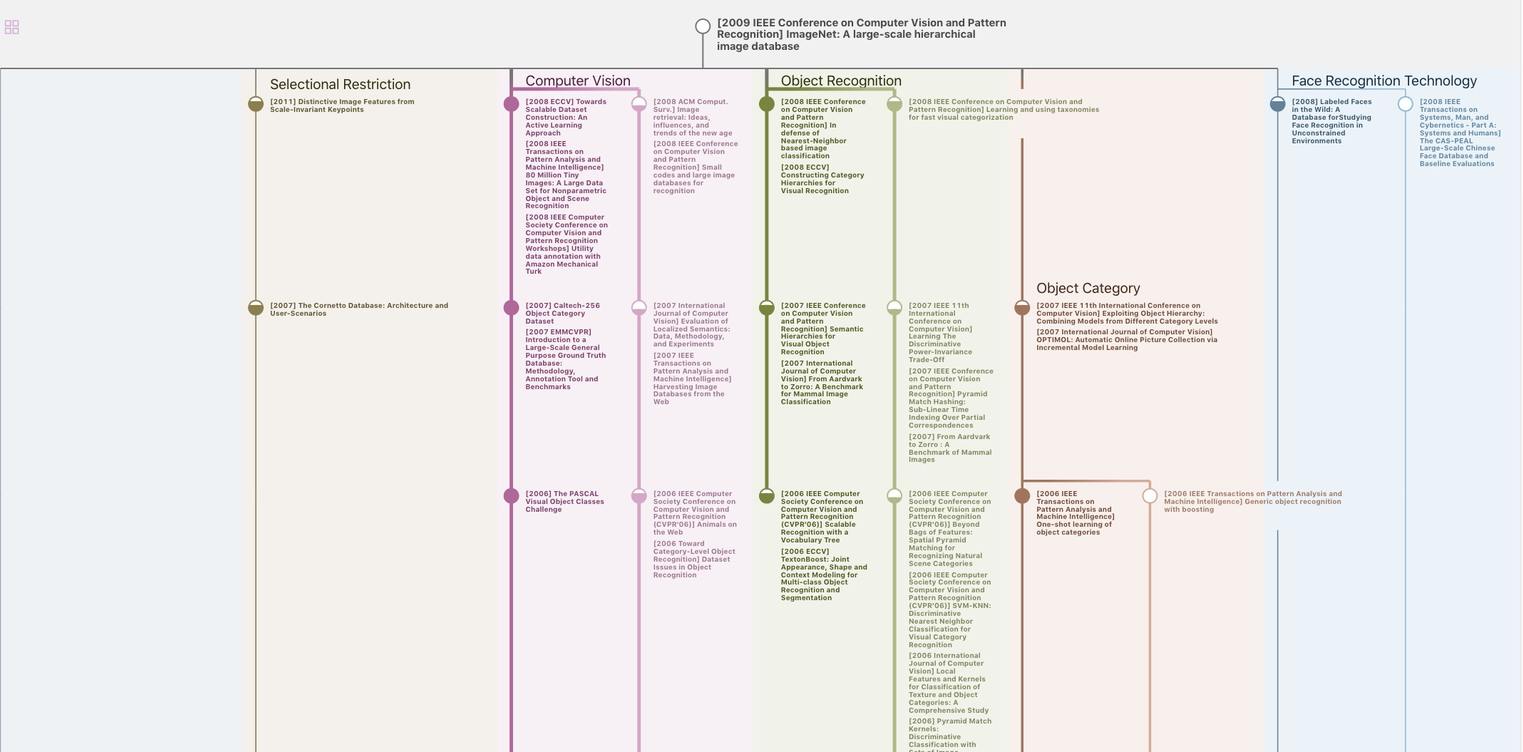
生成溯源树,研究论文发展脉络
Chat Paper
正在生成论文摘要