Adapting Virtual Embodiment Through Reinforcement Learning
IEEE Transactions on Visualization and Computer Graphics(2022)
摘要
In Virtual Reality, having a virtual body opens a wide range of possibilities as the participant’s avatar can appear to be quite different from oneself for the sake of the targeted application (e.g., for perspective-taking). In addition, the system can partially manipulate the displayed avatar movement through some distortion to make the overall experience more enjoyable and effective (e.g., training, exercising, rehabilitation). Despite its potential, an excessive distortion may become noticeable and break the feeling of being embodied into the avatar. Past researches have shown that individuals have a relatively high tolerance to movement distortions and a great variability of individual sensitivities to distortions. In this article, we propose a method taking advantage of Reinforcement Learning (RL) to efficiently identify the magnitude of the maximum distortion that does not get noticed by an individual (further noted the detection threshold). We show through a controlled experiment with subjects that the RL method finds a more robust detection threshold compared to the adaptive staircase method, i.e., it is more able to prevent subjects from detecting the distortion when its amplitude is equal or below the threshold. Finally, the associated majority voting system makes the RL method able to handle more noise within the forced choices input than adaptive staircase. This last feature is essential for future use with physiological signals as these latter are even more susceptible to noise. It would then allow to calibrate embodiment individually to increase the effectiveness of the proposed interactions.
更多查看译文
关键词
Computer Graphics,Humans,Movement,User-Computer Interface,Virtual Reality
AI 理解论文
溯源树
样例
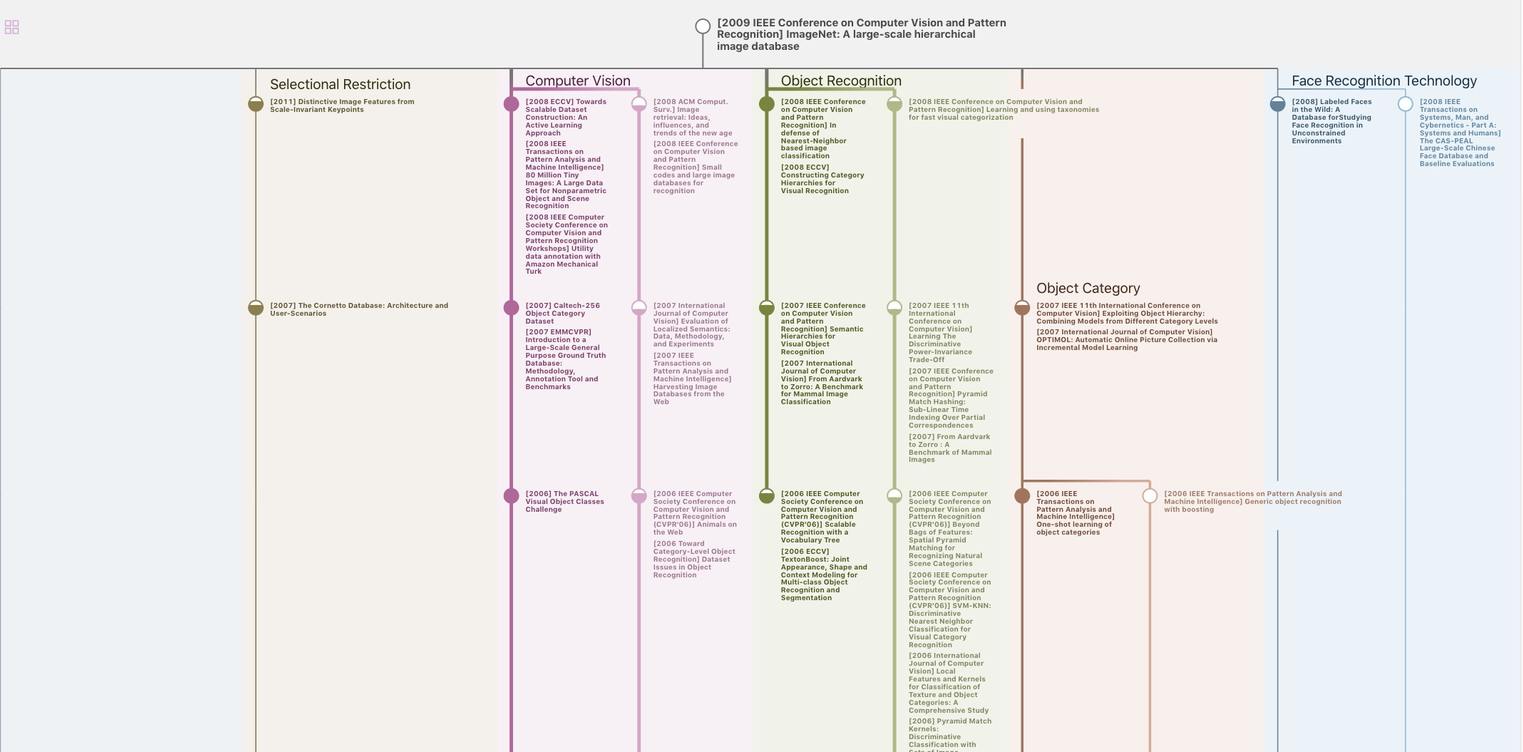
生成溯源树,研究论文发展脉络
Chat Paper
正在生成论文摘要