Generating Fake Cyber Threat Intelligence Using Transformer-Based Models
2021 INTERNATIONAL JOINT CONFERENCE ON NEURAL NETWORKS (IJCNN)(2021)
摘要
Cyber-defense systems are being developed to automatically ingest Cyber Threat Intelligence (CTI) that contains semi-structured data and/or text to populate knowledge graphs. A potential risk is that fake CTI can be generated and spread through Open-Source Intelligence (OSINT) communities or on the Web to effect a data poisoning attack on these systems. Adversaries can use fake CTI examples as training input to subvert cyber defense systems, forcing their models to learn incorrect inputs to serve the attackers' malicious needs. In this paper, we show how to automatically generate fake CTI text descriptions using transformers. Given an initial prompt sentence, a public language model like GPT-2 with fine-tuning can generate plausible CTI text that can mislead cyber-defense systems. We use the generated fake CTI text to perform a data poisoning attack on a Cybersecurity Knowledge Graph (CKG) and a cybersecurity corpus. The attack introduced adverse impacts such as returning incorrect reasoning outputs, representation poisoning, and corruption of other dependent AI-based cyber defense systems. We evaluate with traditional approaches and conduct a human evaluation study with cyber-security professionals and threat hunters. Based on the study, professional threat hunters were equally likely to consider our fake generated CTI and authentic CTI as true.
更多查看译文
关键词
Cybersecurity, Cyber Threat Intelligence, Artificial Intelligence, Data Poisoning Attack
AI 理解论文
溯源树
样例
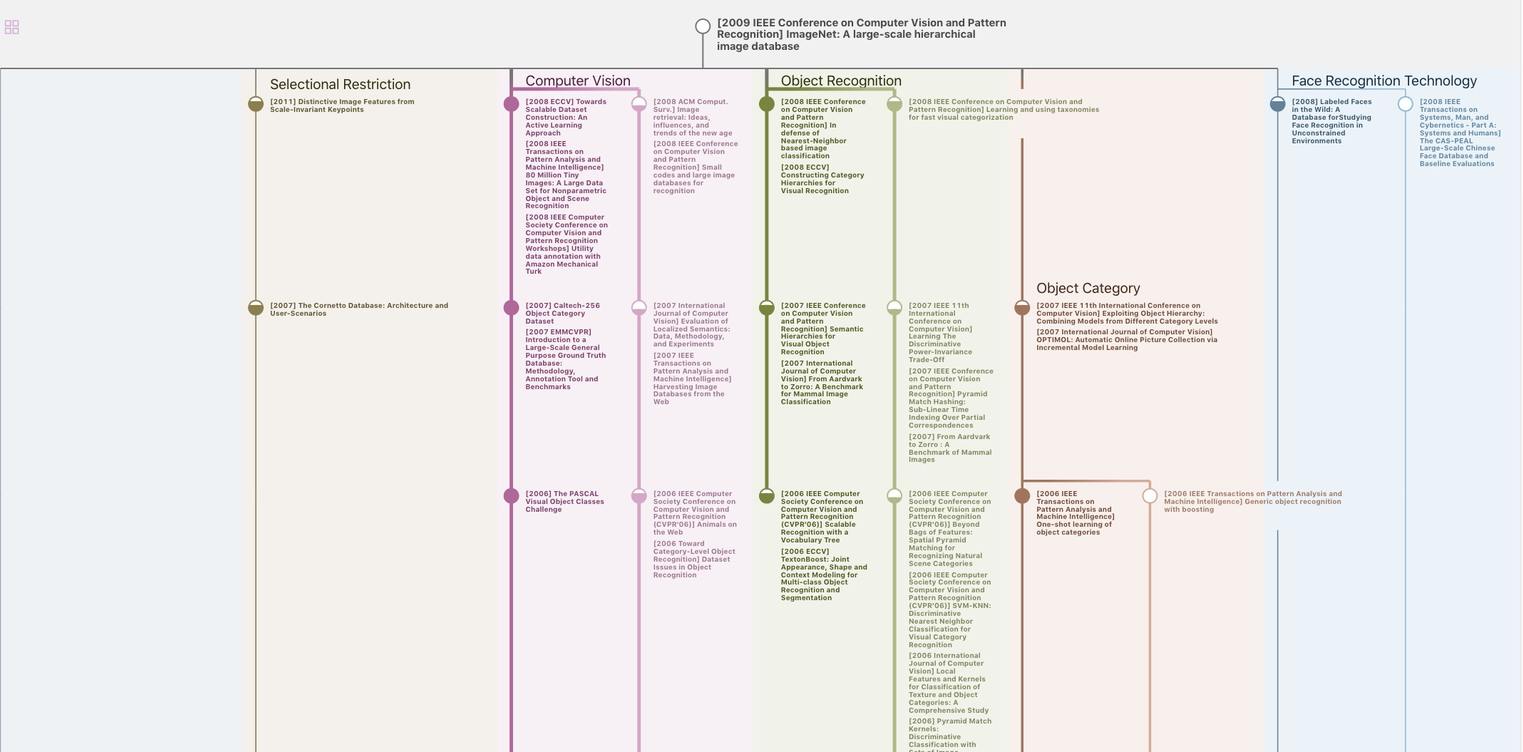
生成溯源树,研究论文发展脉络
Chat Paper
正在生成论文摘要